An application of deep reinforcement learning and vendor-managed inventory in perishable supply chain management
ENGINEERING APPLICATIONS OF ARTIFICIAL INTELLIGENCE(2024)
摘要
This article delves into the challenging supply chain management domain, explicitly addressing the intricate issue of perishable inventory allocation within a two-echelon supply chain. The approach outlined here leverages deep reinforcement learning with a keen understanding of the inherent stochasticity arising from uncertain demands and variable supply conditions. The examined supply chain encompasses two retailers and a central distribution center operating under a vendor-managed inventory system. The primary goal of this research is to combat the prevalent problems of wastage and shortages frequently encountered at the retail level in such supply chains. The study employs the Advantage Actor-Critic (A2C) algorithm, tailored to handle the continuous action space inherent in inventory allocation. To rigorously evaluate this approach, empirical data from a real-world blood supply chain in Tabriz is used for numerical experiments. This practical case study involves a single distribution center and two hospitals. The outcomes of these experiments affirm the effectiveness of the A2C algorithm, showcasing its ability to address the complex inventory allocation problem successfully. Furthermore, the research highlights that the algorithm outperforms existing supply chain policies, underscoring the pivotal role of optimal allocation in enhancing efficiency and operational excellence in perishable supply chains.
更多查看译文
关键词
Deep reinforcement learning,Perishable inventory,Inventory allocation,Vendor managed inventory,Policy gradient,Advantage actor-critic
AI 理解论文
溯源树
样例
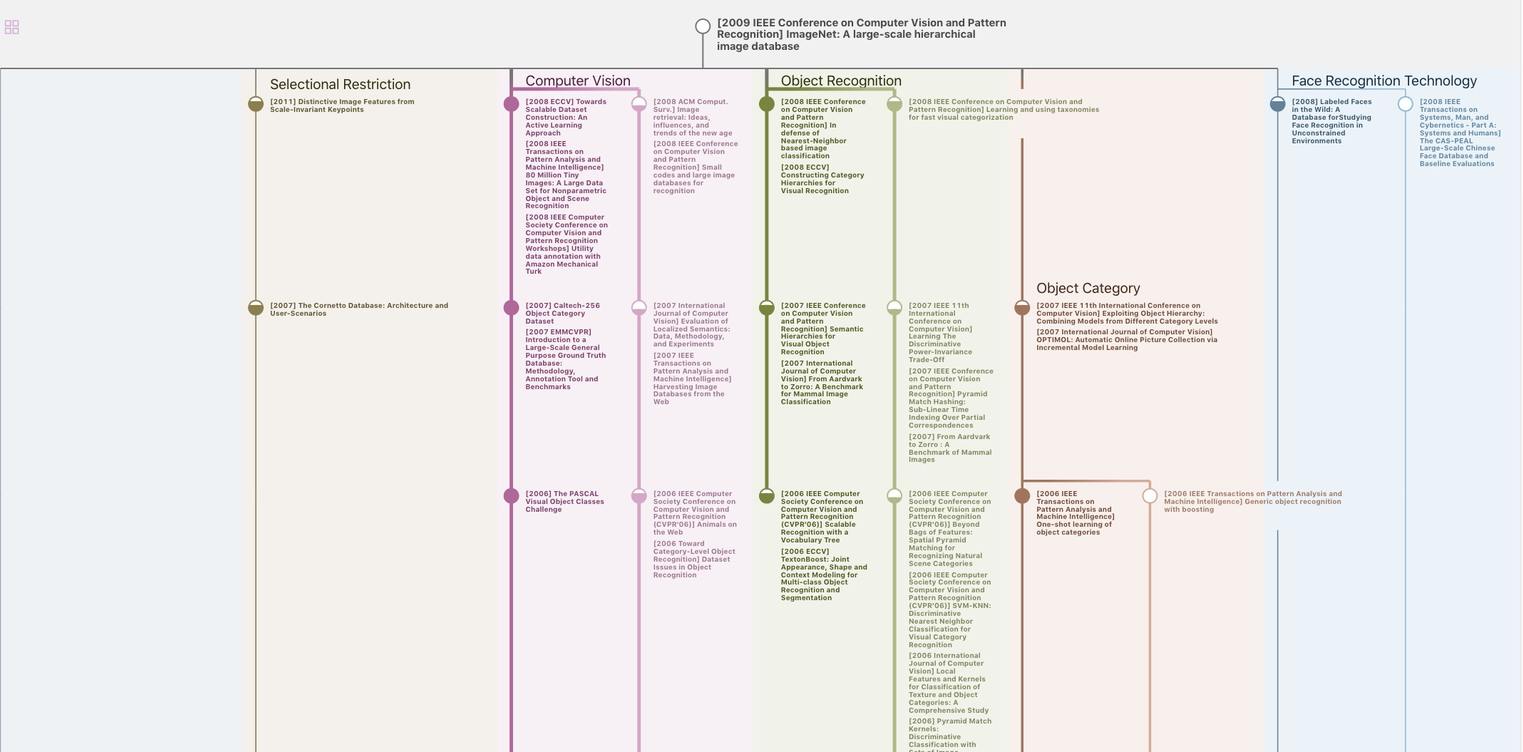
生成溯源树,研究论文发展脉络
Chat Paper
正在生成论文摘要