Invited Paper: 2023 ICCAD CAD Contest Problem C: Static IR Drop Estimation Using Machine Learning
2023 IEEE/ACM International Conference on Computer Aided Design (ICCAD)(2023)
摘要
Power delivery network (PDN) analysis is a critical aspect of the design cycle to ensure the power grid meets the current demands of the chip. Static IR drop simulation, performed as a part of PDN analysis, is crucial to the estimation of the worstcase voltage drop (IR) drop of the chip which in turn determines chip frequency and functionality. Algorithmically, the static IR drop simulation amounts to solving a large system of linear equations with billions of variables and is computationally very expensive with significantly large runtimes. This contest aims at leveraging machine learning (ML) techniques to overcome this challenge. While previous research has introduced ML-based solutions for static IR drop simulation, their performance remains untested on standardized benchmarks, obscuring the true state-of-the-art ML model. The contest releases twenty real circuit (split as ten seen during training and ten as hidden) benchmarks and hundreds of synthetic benchmarks for training ML models to predict IR drop. The synthetic data serves as a training dataset, which can then be fine-tuned using limited real circuit data for accurate predictions on unseen testcases. The goal of the contest is to train novel ML algorithms to perform the prediction with high accuracy, F1 score, and low runtimes.
更多查看译文
AI 理解论文
溯源树
样例
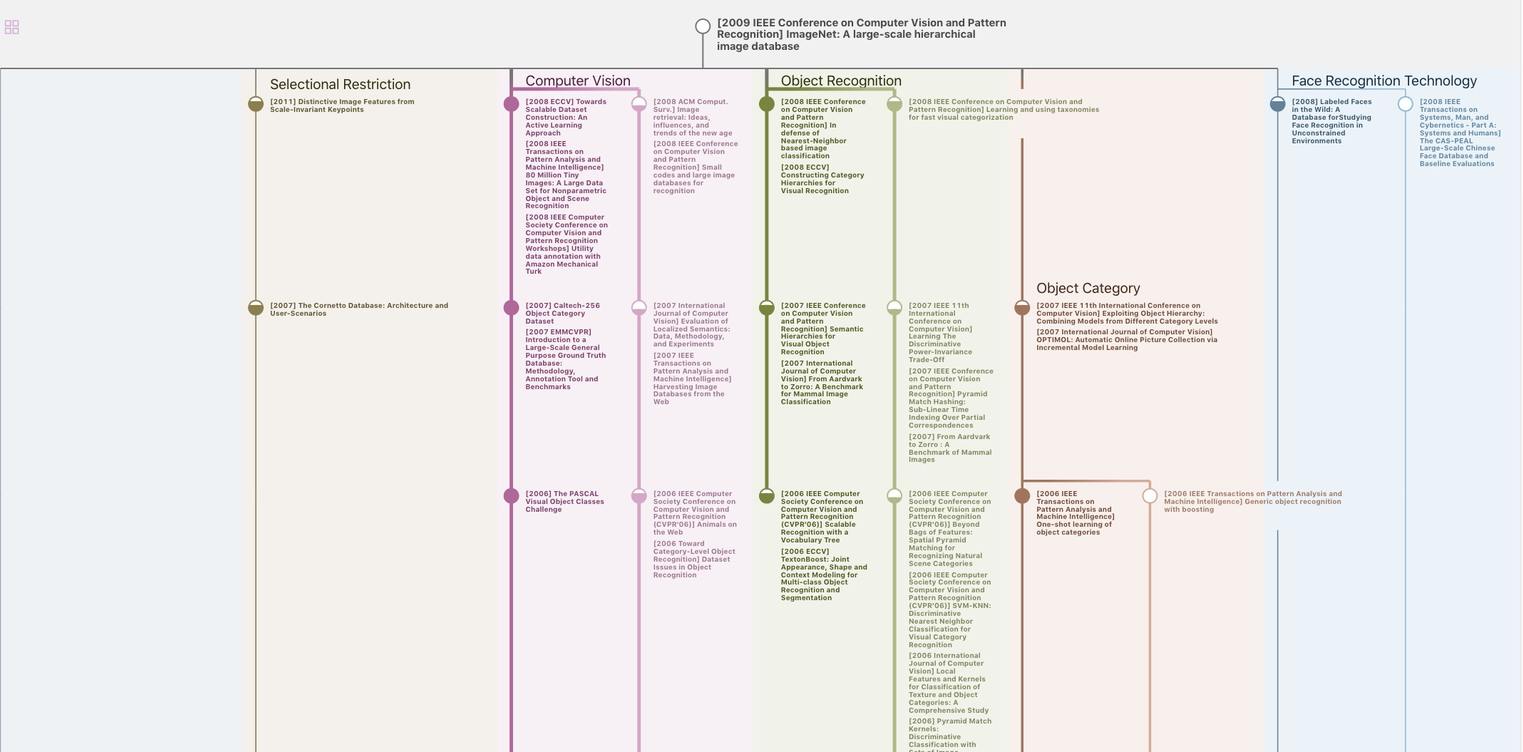
生成溯源树,研究论文发展脉络
Chat Paper
正在生成论文摘要