OPT: Optimal Proposal Transfer for Efficient Yield Optimization for Analog and SRAM Circuits
2023 IEEE/ACM INTERNATIONAL CONFERENCE ON COMPUTER AIDED DESIGN, ICCAD(2023)
摘要
Yield optimization is one of the central challenges in submicrometer integrated circuit manufacture. However, yield optimization is computationally expensive due to intensive yield estimation and intractable optimization processes. In this work, we first reinvent the state-of-the-art all sensitivity adversarial importance sampling (ASAIS) yield optimization from a Laplace approximation perspective, which also reveals its limitations and suggests improvements. We then generalize it with infinite components and discover the key ingredient in yield optimization to be an effective proposal distribution transfer (OPT) procedure, which is captured using conditional normalizing flow (CNF). To deliver a reliable yield optimization pipeline that accounts for the uncertainty due to the lack of data, we propose sequential ensemble, the first empirical uncertainty estimation that enables tractable Bayesian yield optimization without introducing an extra surrogate for the first time. We conduct extensive experiments against five state-of-the-art baselines and show that the proposed method delivers superior performance: a speedup of 1.01x-11.94x (5.57x on average) with higher yield designs, and most importantly, excellent robustness and consistency in all our experiments on analog and SRAM circuits.
更多查看译文
关键词
Yield Estimation,Yield Optimization,Importance Sampling,Conditional Normalizing Flow
AI 理解论文
溯源树
样例
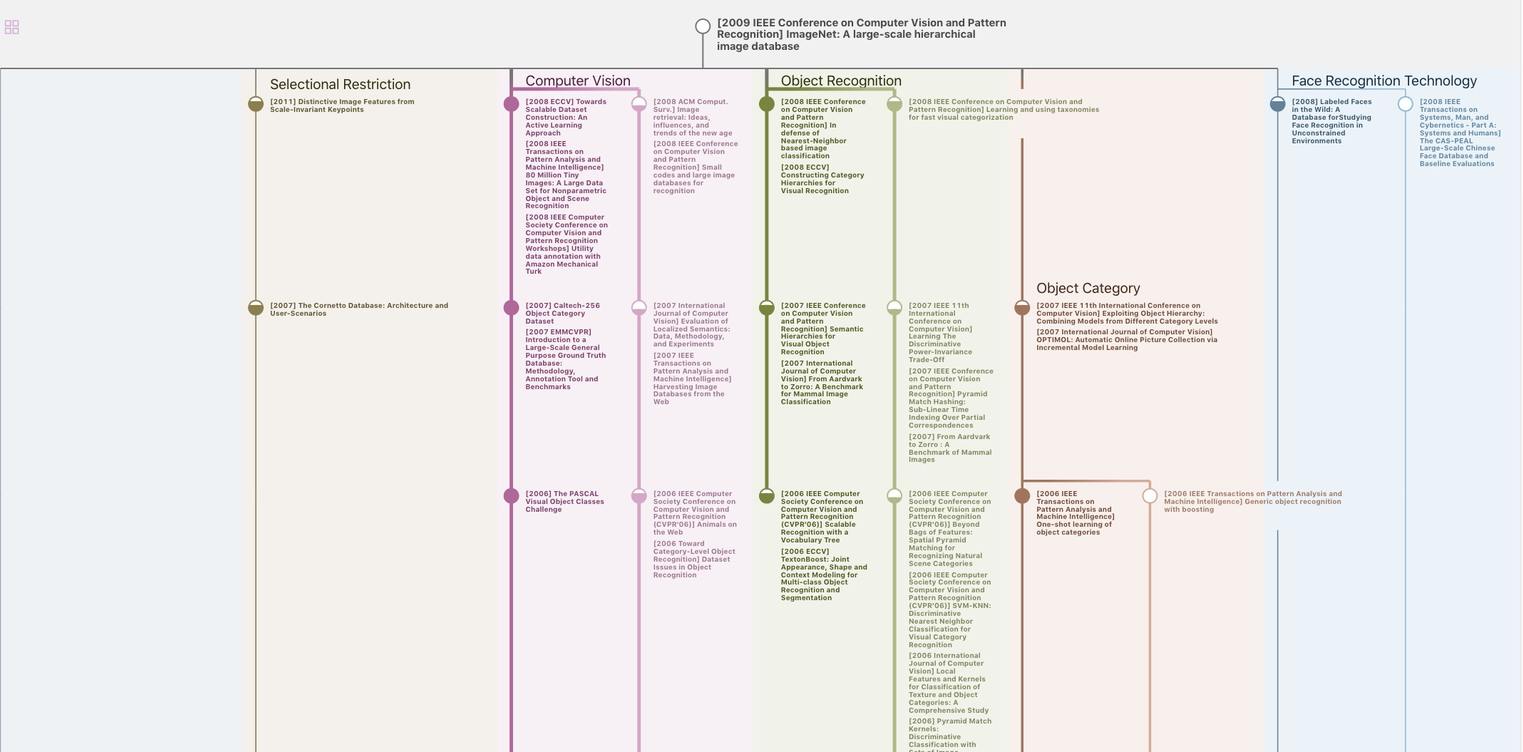
生成溯源树,研究论文发展脉络
Chat Paper
正在生成论文摘要