VIFST: Video Inpainting Localization Using Multi-view Spatial-Frequency Traces.
PRICAI (3)(2023)
摘要
Video inpainting techniques based on deep learning have shown promise in removing unwanted objects from videos. However, their misuse can lead to harmful outcomes. While current methods excel in identifying known forgeries, they struggle when facing unfamiliar ones. Thus, it is crucial to design a video inpainting localization method that exhibits better generalization. The key hurdle lies in devising a network that can extract more generalized forgery features. A notable observation is that the forgery regions often exhibit disparities in forgery traces, such as boundaries, pixel distributions, and region characteristics, when contrasted with the original areas. These traces are prevalent in various inpainted videos, and harnessing them could bolster the detection’s versatility. Based on these multi-view traces, we introduce a three-stage solution termed VIFST: 1) The Spatial and Frequency Branches capture diverse traces, including edges, pixels, and regions, from different viewpoints; 2) local feature learning via CNN-based MaxPoolFormer; and 3) global context feature learning through Transformer-based InterlacedFormer. By integrating local and global feature learning networks, VIFST enhances fine-grained pixel-level detection performance. Extensive experiments demonstrate the effectiveness of our method and its superior generalization performance compared to state-of-the-art approaches. The source code for our method has been published on GitHub: https://github.com/lajlksdf/UVL .
更多查看译文
关键词
video inpainting localization,multi-view,spatial-frequency
AI 理解论文
溯源树
样例
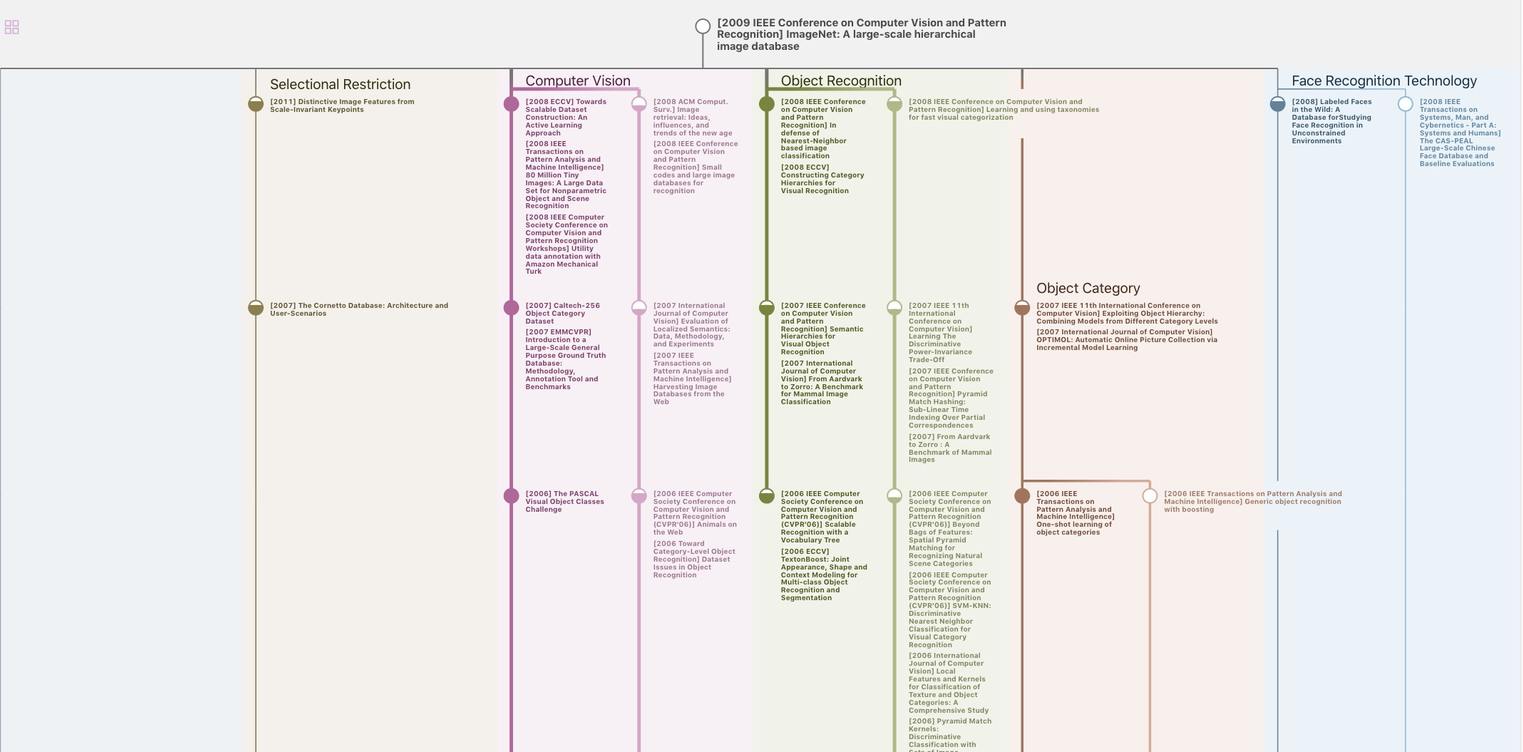
生成溯源树,研究论文发展脉络
Chat Paper
正在生成论文摘要