Preserving Potential Neighbors for Low-Degree Nodes via Reweighting in Link Prediction
NEURAL INFORMATION PROCESSING, ICONIP 2023, PT II(2024)
摘要
Link prediction is an important task for graph data. Methods based on graph neural networks achieve high accuracy by simultaneously modeling the node attributes and structure of the observed graph. However, these methods often get worse performance for low-degree nodes. After theoretical analysis, we find that current link prediction methods focus more on negative samples for low-degree nodes, which makes it hard to find potential neighbors for these nodes during inference. In order to improve the performance on low-degree nodes, we first design a nodewise score to quantify how seriously the training is biased to negative samples. Based on the score, we develop a reweighting method called harmonic weighting(HAW) to help the model preserve potential neighbors for low-degree nodes. Experimental results show that the model combined with HAW can achieve better performance on most datasets. By detailedly analyzing the performance on nodes with different degrees, we find that HAW can preserve more potential neighbors for low-degree nodes without reducing the performance of other nodes.
更多查看译文
关键词
Link prediction,Sample reweighting,Harmonic weighting
AI 理解论文
溯源树
样例
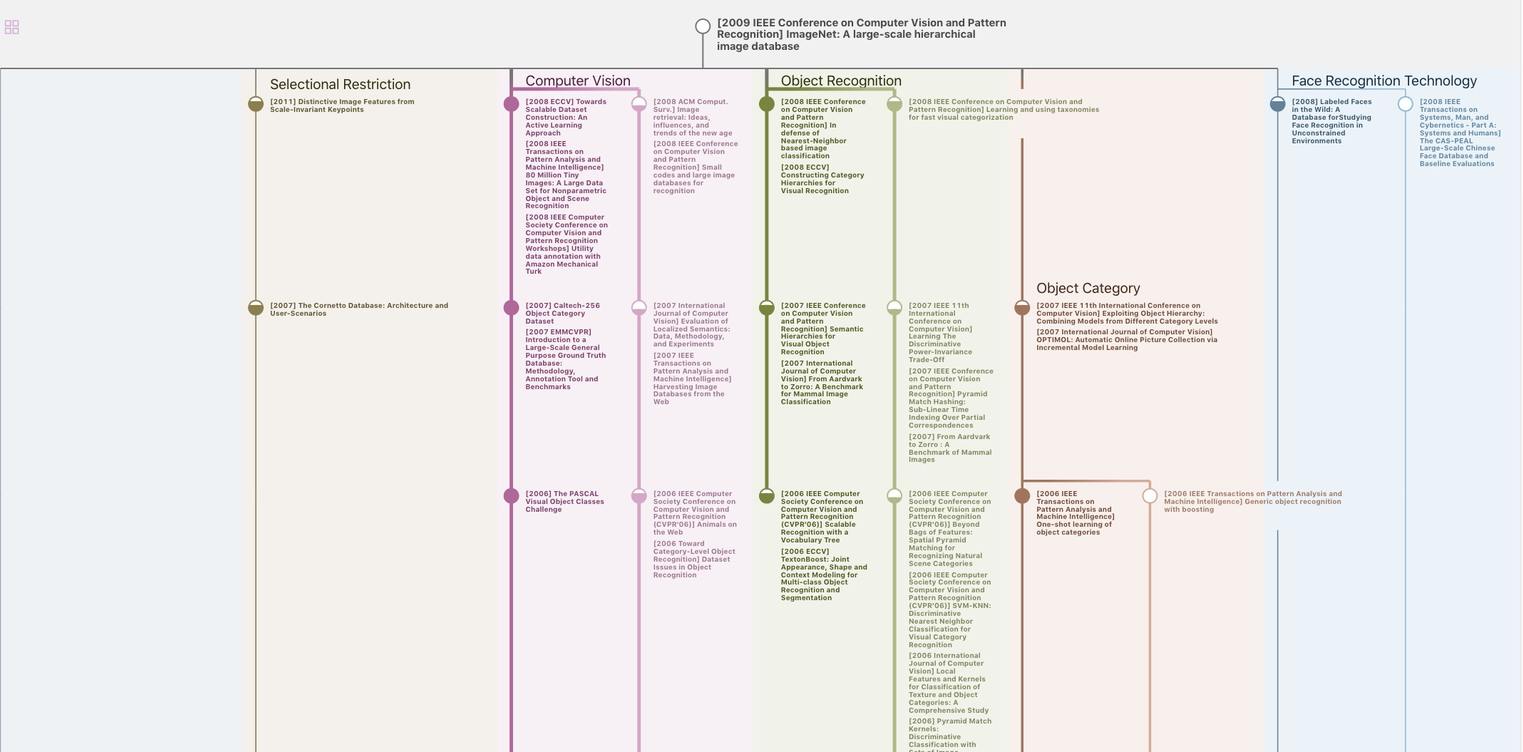
生成溯源树,研究论文发展脉络
Chat Paper
正在生成论文摘要