Gradient-Assisted Calibration for Financial Agent-Based Models
PROCEEDINGS OF THE 4TH ACM INTERNATIONAL CONFERENCE ON AI IN FINANCE, ICAIF 2023(2023)
摘要
Agent-based modelling (ABMing) is a promising approach to modelling and reasoning about complex systems such as financial markets. However, the application of ABMs in practice is often impeded by the models' complexity and the ensuing difficulty of performing parameter inference and optimisation tasks. This in turn has motivated efforts directed towards the construction of differentiable ABMs, enabled by recently developed effective auto-differentiation frameworks, as a strategy for addressing these challenges. In this paper, we discuss and present experiments that demonstrate how differentiable programming may be used to implement and calibrate heterogeneous ABMs in finance. We begin by considering in more detail the difficulties inherent in constructing gradients for discrete ABMs. Secondly, we illustrate solutions to these difficulties, by using a discrete agent-based market simulation model as a case study. Finally, we show through numerical experiments how our differentiable implementation of this discrete ABM enables the use of powerful tools from probabilistic machine learning and conditional generative modelling to perform robust parameter inferences and uncertainty quantification, in a simulation-efficient manner.
更多查看译文
关键词
agent-based models,automatic differentiation,parameter inference
AI 理解论文
溯源树
样例
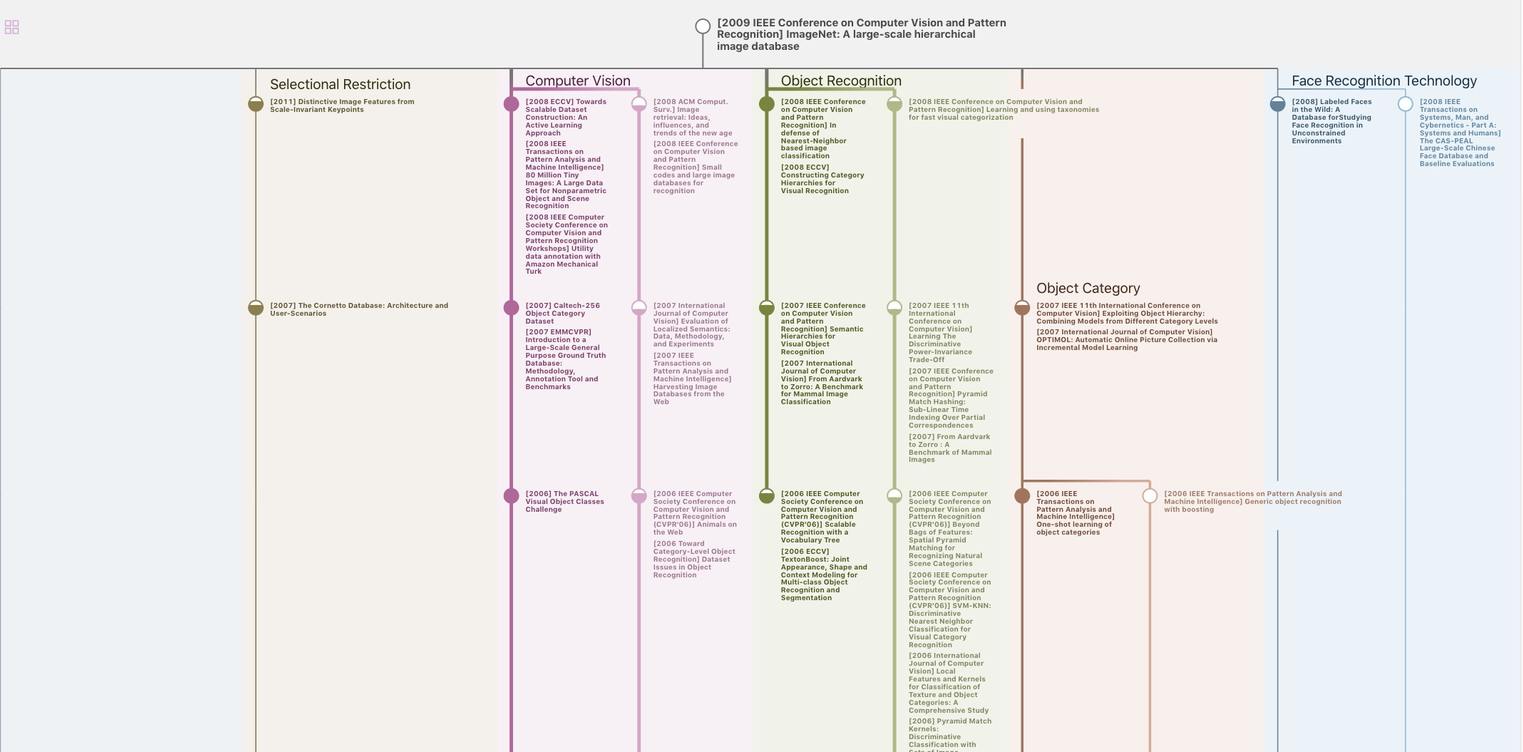
生成溯源树,研究论文发展脉络
Chat Paper
正在生成论文摘要