Cryptography-Inspired Federated Learning for Generative Adversarial Networks and Meta Learning.
ADMA (2)(2023)
摘要
Federated learning (FL) aims to derive a “better” global model without direct access to individuals’ training data. It is traditionally done by aggregation over individual gradients with differentially private (DP) noises. We study an FL variant as a new point in the privacy-performance space. Namely, cryptographic aggregation is over local models instead of gradients; each contributor then locally trains their model using a DP version of Adam upon the “feedback” (e.g., fake samples from GAN – generative adversarial networks) derived from the securely-aggregated global model. Intuitively, this achieves the best of both worlds – more “expressive” models are processed in the encrypted domain instead of just gradients, without DP’s shortcoming, while heavyweight cryptography is minimized (at only the first step instead of the entire process). Practically, we showcase this new FL variant over GAN and meta-learning, for securing new data and new tasks.
更多查看译文
关键词
federated learning,generative adversarial networks,adversarial networks,cryptography-inspired
AI 理解论文
溯源树
样例
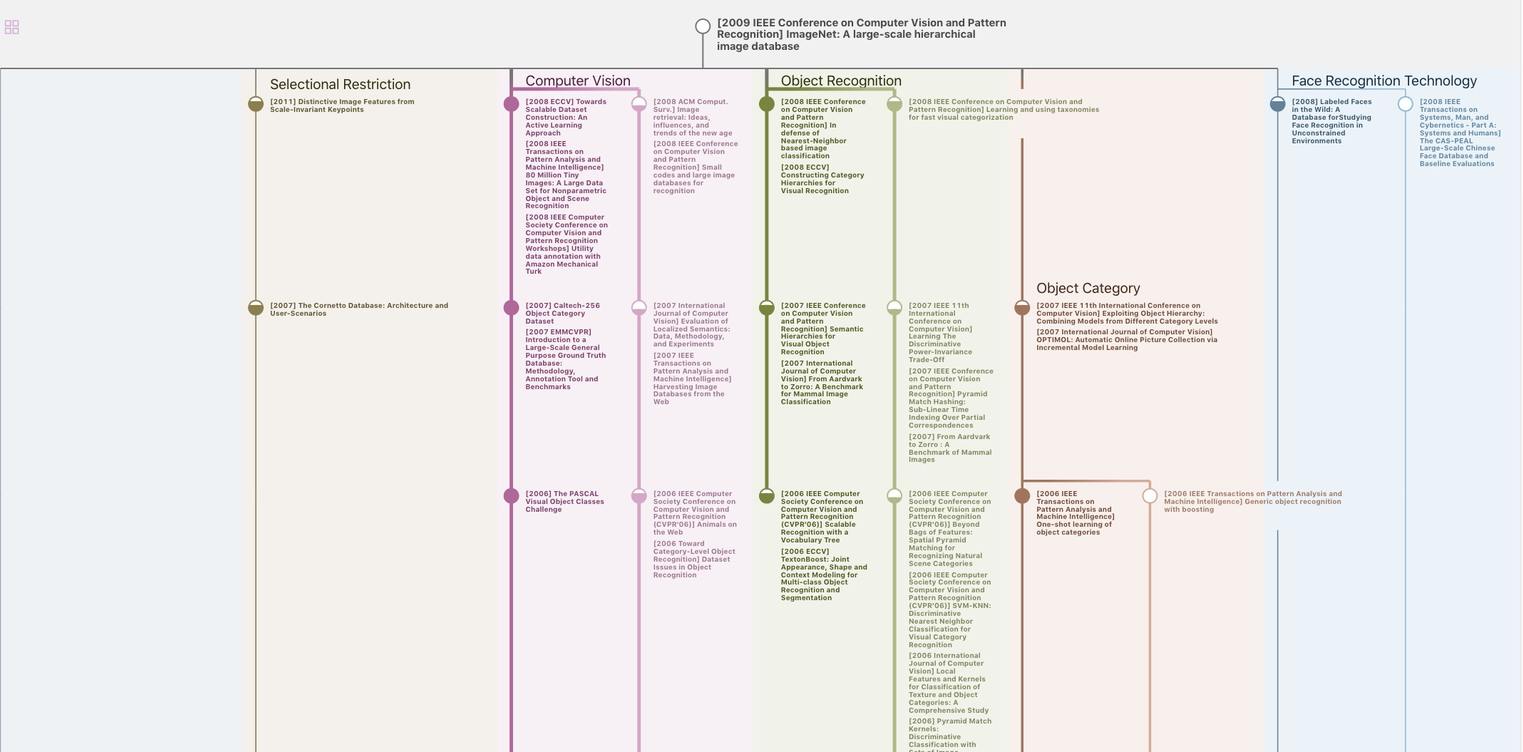
生成溯源树,研究论文发展脉络
Chat Paper
正在生成论文摘要