Monte Carlo Denoising via Multi-scale Auxiliary Feature Fusion Guided Transformer
SIGGRAPH Asia Technical Communications(2023)
摘要
Deep learning-based single-frame Monte Carlo denoising techniques have demonstrated remarkable results in photo-realistic rendering research. However, the current state-of-the-art methods relying on self-attention mechanisms underutilize auxiliary features and struggle to preserve intricate high-frequency details in complex scenes. Employing a generative adversarial architecture, we present a transformer-based denoising network guided by multi-scale auxiliary feature. The proposed U-shaped denoising network extracts multi-scale texture and geometric features from auxiliaries, modulating them to guide the improved transformer module's denoising process. The improved transformer module employs cross-channel self-attention to capture non-local relationships with near-linear computational complexity. Additionally, a gating mechanism is introduced in the transformer module's feed-forward network, enhancing information flow. Extensive experiments on noisy images with varied per-pixel sampling rates demonstrate the method's superiority in quantitative metrics and visual perception compared with state-of-the-art methods. Our method excels notably in intricate scenes with complex hair and texture details, which are historically challenging to denoise.
更多查看译文
关键词
Monte Carlo denoising,Transformer,self-attention
AI 理解论文
溯源树
样例
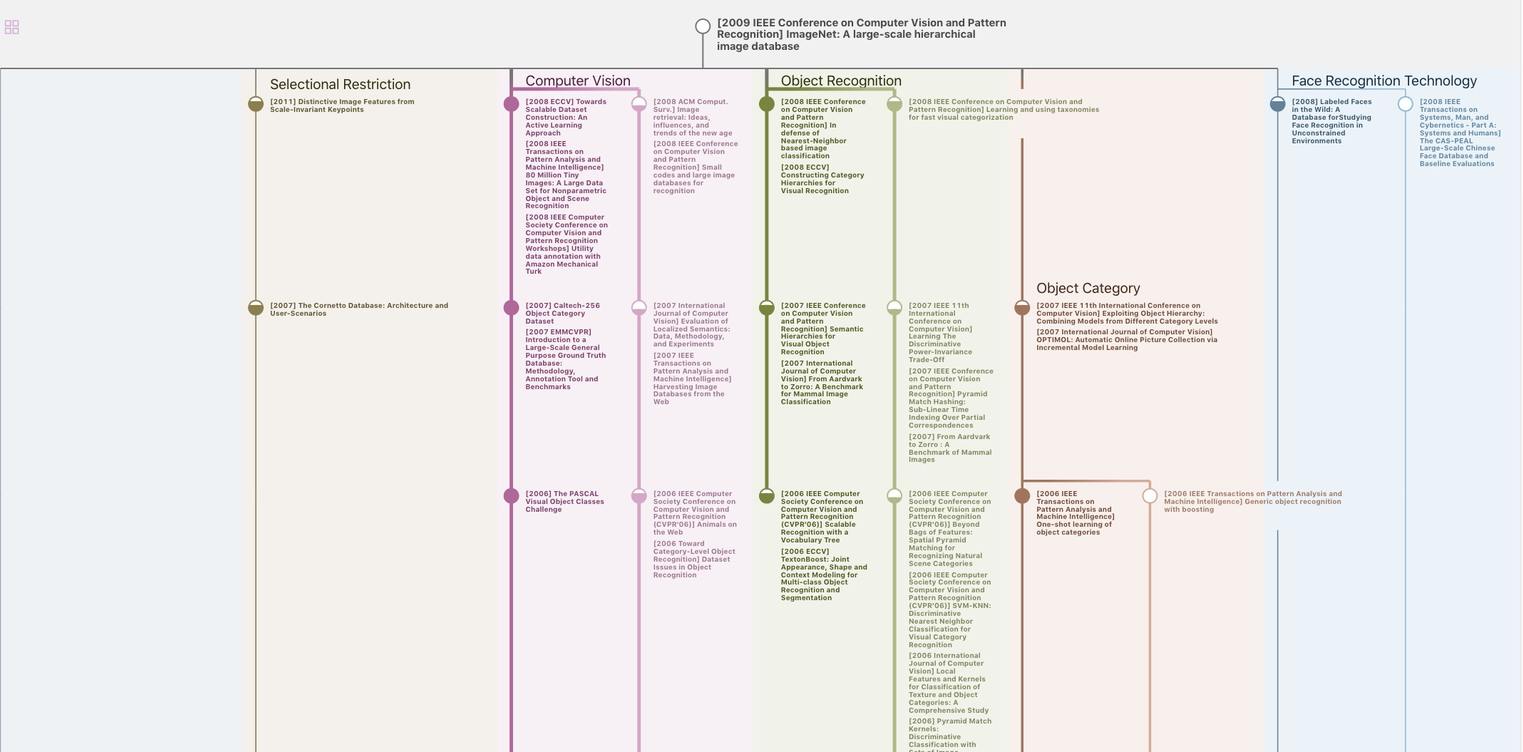
生成溯源树,研究论文发展脉络
Chat Paper
正在生成论文摘要