Multi-view subspace clustering via consistent and diverse deep latent representations
Inf. Sci.(2023)
摘要
Multi-view subspace clustering has recently received great interest in the communities of computer vision and pattern recognition, as it can overcome the limitation of conventional single-view subspace clustering by fusing complementary information from multi-view features. Recent work emphasizes the learning of latent representations rather than the affinity graph from each view to accomplish the task. Although they try to obtain consistent latent representations, it is difficult for these methods to design the term explicitly enforcing the desirable property such as diversity on the latent representation in their models. In this situation, we do not know if these methods can collect sufficient complementary information from multi-view features. In this paper, we propose Consistent and Diverse Deep Latent Representations (CDDLR) to address this problem. Our method provides a framework for enforcing necessary properties such as consistency and diversity on the learned latent representation by relating K-means with spectral clustering. Adopting the Laplacian regularized deep neural networks and novel strategies about diversity and consistency, CDDLR can learn the consistent and diverse deep latent representation for multi-view subspace clustering. Our experiment shows that for CALTECH and Football, our proposed method improves accuracy by 11.37% and 4.01%, respectively, compared to the second-best method.
更多查看译文
关键词
Multi-view subspace clustering,Deep latent representations,Diversity,Consistency
AI 理解论文
溯源树
样例
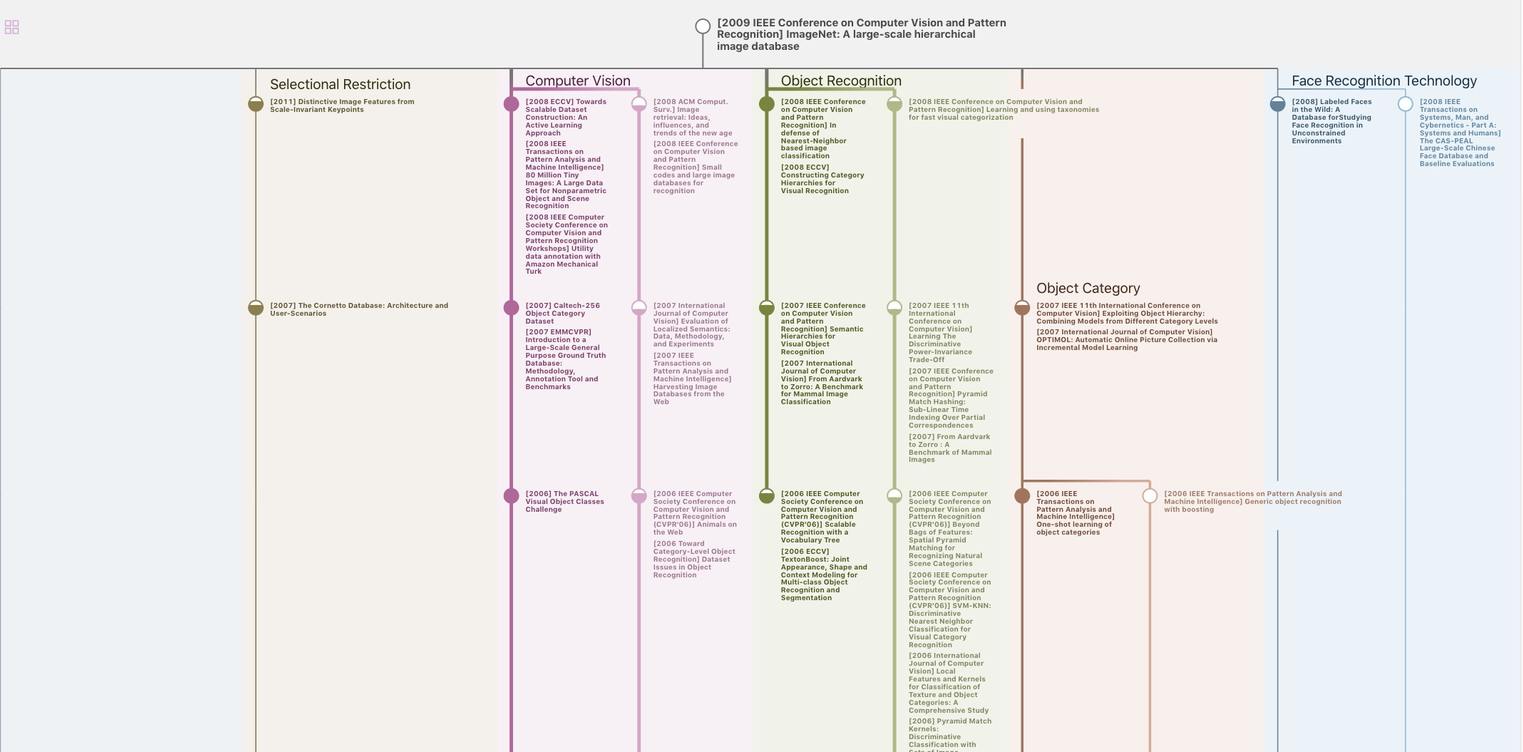
生成溯源树,研究论文发展脉络
Chat Paper
正在生成论文摘要