A novel imbalanced dataset mitigation method and ECG classification model based on combined 1D_CBAM-autoencoder and lightweight CNN model
BIOMEDICAL SIGNAL PROCESSING AND CONTROL(2024)
摘要
To address the problems of the imbalanced datasets, limited computing power, and memory capability of wearable devices, this paper proposes a new hybrid method for imbalanced dataset mitigation and a novel lightweight Convolutional Neural Network (CNN) framework for electrocardiogram(ECG) signal classification. First, data enhancement method is used by changing the amplitude of a small number of samples and combining with the weighted loss function in the deep learning model. Then, One-Dimensional Convolutional Block Attention Module (1D_CBAM) mechanism autoencoder model is proposed to compress the ECG heartbeat signals and extract the features of the ECG heartbeats. A modified one-dimensional lightweight CNN model based on MobileNet architecture is eventually used to categorize various arrhythmias utilizing the above features. Using the MIT-BIH arrhythmia dataset, the proposed model is tested by dividing the MIT Database(MITDB) into Dataset1 and Dataset2, in conjunction with suggestions from AAMI and other heartbeat signal classification methods. The experimental results show that the proposed imbalanced dataset mitigation method has higher performance than other methods in this paper, the proposed model has the most stable convergence and the lowest reconstruction loss (approximately 0.1) in the feature extraction process under the same conditions. During the classification process, the lightweight CNN model has approximately 4x fewer trainable parameters(Params: 12,788) and its performance [Dataset1: F1-Score: 96.24% ACC: 98.21%; Dataset2: F1-Score: 98.73% ACC: 99.61%] is comparable to the traditional CNN model [Dataset1: F1-Score: 96.98% ACC: 99.17%; Dataset2: F1-Score: 99.18% ACC: 99.72%].
更多查看译文
关键词
Electrocardiogram (ECG),ImbalanCed Dataset Mitigation Method,Convolutional Block Attention Module(CBAM),Lightweight CNN model,ECG classification
AI 理解论文
溯源树
样例
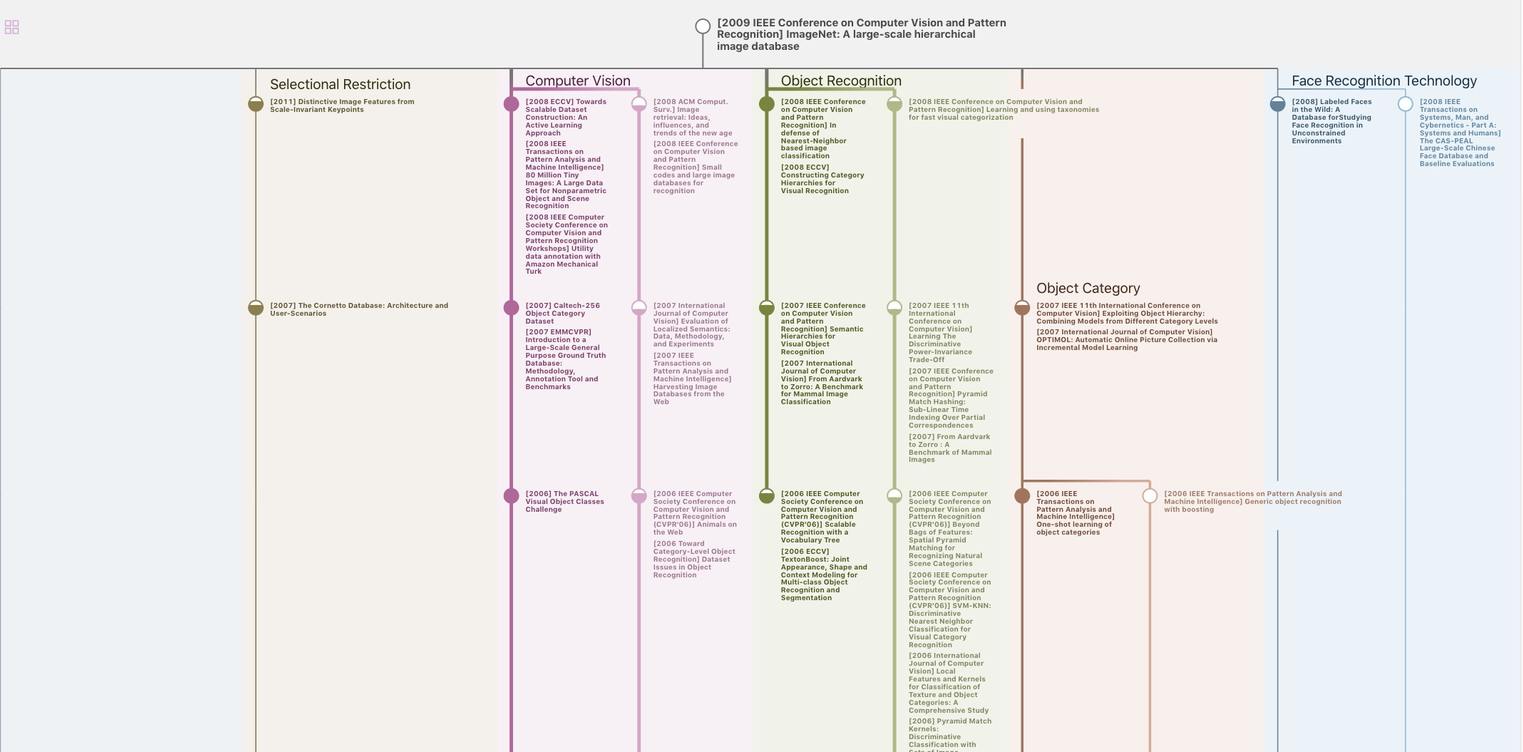
生成溯源树,研究论文发展脉络
Chat Paper
正在生成论文摘要