SRSCL: A strong-relatedness-sequence-based fine-grained collective entity linking method for heterogeneous information networks
EXPERT SYSTEMS WITH APPLICATIONS(2024)
摘要
The development of efficient methods for mining information from heterogeneous information networks (HINs) has become essential for improving the accuracy of collective entity linking in the absence of third-party knowledge bases. Currently, there remain three major challenges in the latest research: (1) The objective function of collective entity linking does not fully embrace the concept of "collective linking". (2) The objective function employs the mean value rather than the maximum value of the entity relatedness as a link parameter, while discounting the importance of the strong logical associations between the text and language for meaning recognition. (3) The objective function utilizes only one type of 2-hop path to contribute to entity relatedness, thereby disregarding other types of 2-hop path that exist in actual HINs. To address the aforementioned issues, this paper proposes a strong-relatedness-sequence-based fine-grained collective entity linking method (SRSCL). The SRSCL is capable of capturing the contextual information of the entity in the HINs, thereby providing improved accuracy in entity linking. Specifically, SRSCL constructs a knowledge representation learning model and proposes an overall semantic similarity model for entity mentions and candidate entities to solve the objective function and thereby reflect the idea of "collective linking". Additionally, a strong-relatednesssequence-based overall relatedness measurement model is proposed for candidate entities to emphasize the strong logical associations between them. Furthermore, SRSCL defines three types of 2-hop path and evaluates the importance of each path to accurately measure the relatedness of entities. Finally, the experimental results demonstrate that the proposed SRSCL is more effective in capturing the overall relatedness of entities than the latest model. Particularly, when the number of entity mentions contained in one sliding window is greater than 6, the proposed SRSCL improves the precision, recall and F1 score by more than 10% compared with the latest model.
更多查看译文
关键词
Collective entity linking,Heterogeneous information network,Overall relatedness,Strong relatedness sequence,Knowledge representation
AI 理解论文
溯源树
样例
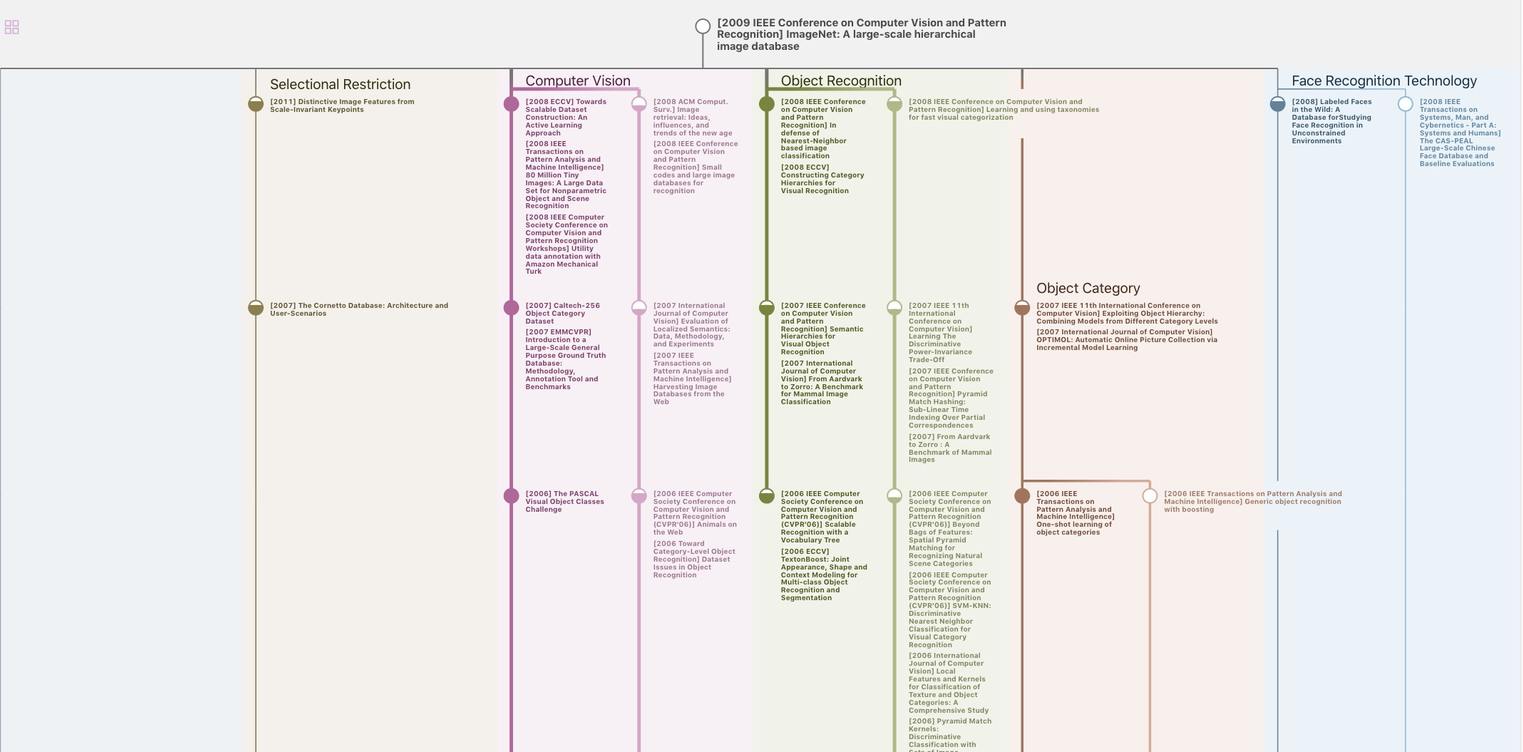
生成溯源树,研究论文发展脉络
Chat Paper
正在生成论文摘要