DSteganoM: Deep steganography for motion capture data
EXPERT SYSTEMS WITH APPLICATIONS(2024)
摘要
Motion capture (mocap) data stores the skeleton movement of recorded objects or humans and is essential for various 3D applications, such as games, animations, virtual reality, surveillance and medical. The exploration of steganography using mocap data is still limited despite its wide-range of potential practical applications. Compared to image, audio, and video steganography, the challenges in mocap steganography arise from differences between the temporal lengths and topologies of the cover and secret data, non-zero residuals between mocap data sequences, non-repetitive movements in different chunks of the mocap data. Deep neural network (DNN) based hiding models could be a potential solution to address these challenges in mocap steganography. DNN-based steganography has been adopted in many recent works and has shown promising results in steganographic techniques in various domains, including image, audio and video; however, to date, no prior work has investigated the application of DNN models for hiding mocap data. In this paper, we investigate solutions to deal with the challenges in mocap steganography and propose a novel end-to-end deep neural network model, DSteganoM, to hide a sequence of skeletal motions into another. The proposed model is capable of learning and exploring the redundancy of spatial and temporal Euclidean positional information in each skeletal motion for the hiding task. In addition, by introducing upsampling and pooling mechanisms in our network architecture, the DSteganoM model also can hide mocap data of different topologies with different dimensions. Experimental results show that the proposed DSteganoM model outperforms other state-of-the-art models and can visually reconstruct the cover and secret with high resistance to signal perturbation. Our work bridges the research gap in applying deep steganography to address the challenges in mocap data hiding.
更多查看译文
关键词
Steganography,Deep learning,Motion capture,Convolutional neural network,Data hiding
AI 理解论文
溯源树
样例
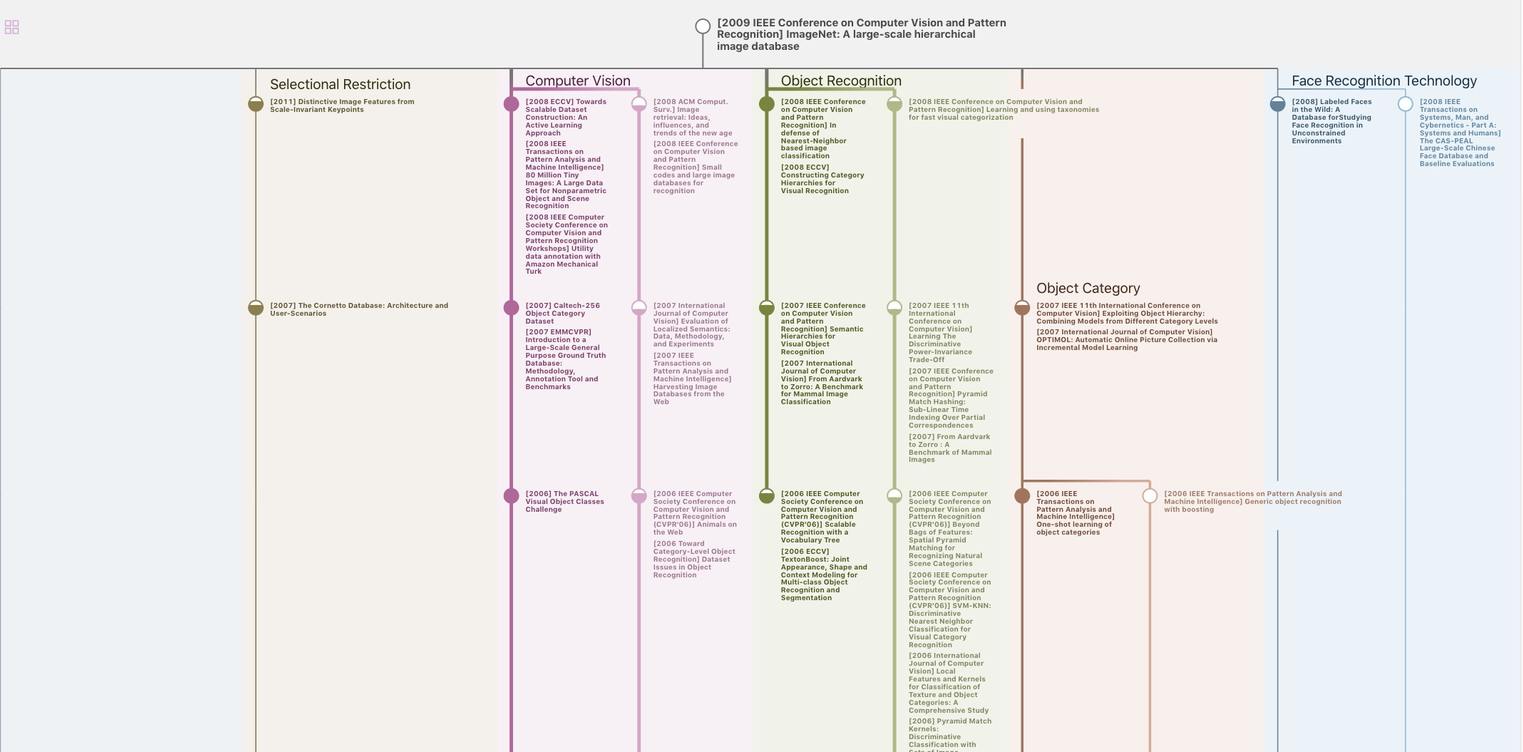
生成溯源树,研究论文发展脉络
Chat Paper
正在生成论文摘要