Data-driven analysis of crustal and subduction seismic environments using interpretation of deep learning-based generalized ground motion models
EXPERT SYSTEMS WITH APPLICATIONS(2024)
摘要
Studies on understanding the regional seismological differences based on the variations in the characteristics of the ground motion waves recorded during seismic events have provided independent insights into the different seismic environments of the world. This study aims to showcase the differences between three of the major seismic environments of the world including Japanese subduction, Chilean subduction, and Californian crustal. The study is based on developing deep learning (DL)-based surrogate generalized ground motion models (GGMMs) and analyzing them to understand the patterns between the earthquake source parameters and the resulting ground motion waveforms' engineering characteristics. The GGMMs are developed using long short-term memory (LSTM) based recurrent neural networks (RNNs), which are trained using six earthquake source and site parameters as the inputs and a 25 x 1 vector of amplitude-, duration-, and energy-based ground motion intensity measures (IMs). The GGMMs are trained and evaluated using carefully selected large datasets of ground motion records from the Japanese subduction, Chilean subduction, and Californian crustal sources (similar to 2000 records from each source). The models are developed in two settings: i) three independent GGMMs using the three datasets of each source, ii) one combined GGMM using the combined dataset. While the former provides individual surrogate models of the regional seismic environments and allows relative comparison among the three environments, the latter acts as a global seismic surrogate model and allows comparison in absolute terms. The seismic environments are investigated by analyzing the two types of GGMMs using explainable artificial intelligence (XAI) and game theory based Shapley explanations (SHAP). As the direct physical study of the seismic environments is not generally feasible/practical, the proposed GGMMs surrogating the process becomes a source of knowledge. By interpreting them, inferences about the seismic environments are derived. Results indicate the peculiar nature of the earthquakes arising from the three seismic backgrounds, further emphasizing the importance of conducting independent regional seismic hazard and risk analysis. In particular, the role of magnitude and rupture distance is observed to have a significantly different impact on the different IMs of the three different environments. The study further sets a novel basis to utilize advanced DL and XAI methods in understanding convoluted physics and engineering phenomena.
更多查看译文
关键词
Generalized ground motion models,Deep learning,Seismic environments,Seismology,Explainable artificial intelligence,Machine learning,Recurrent neural networks
AI 理解论文
溯源树
样例
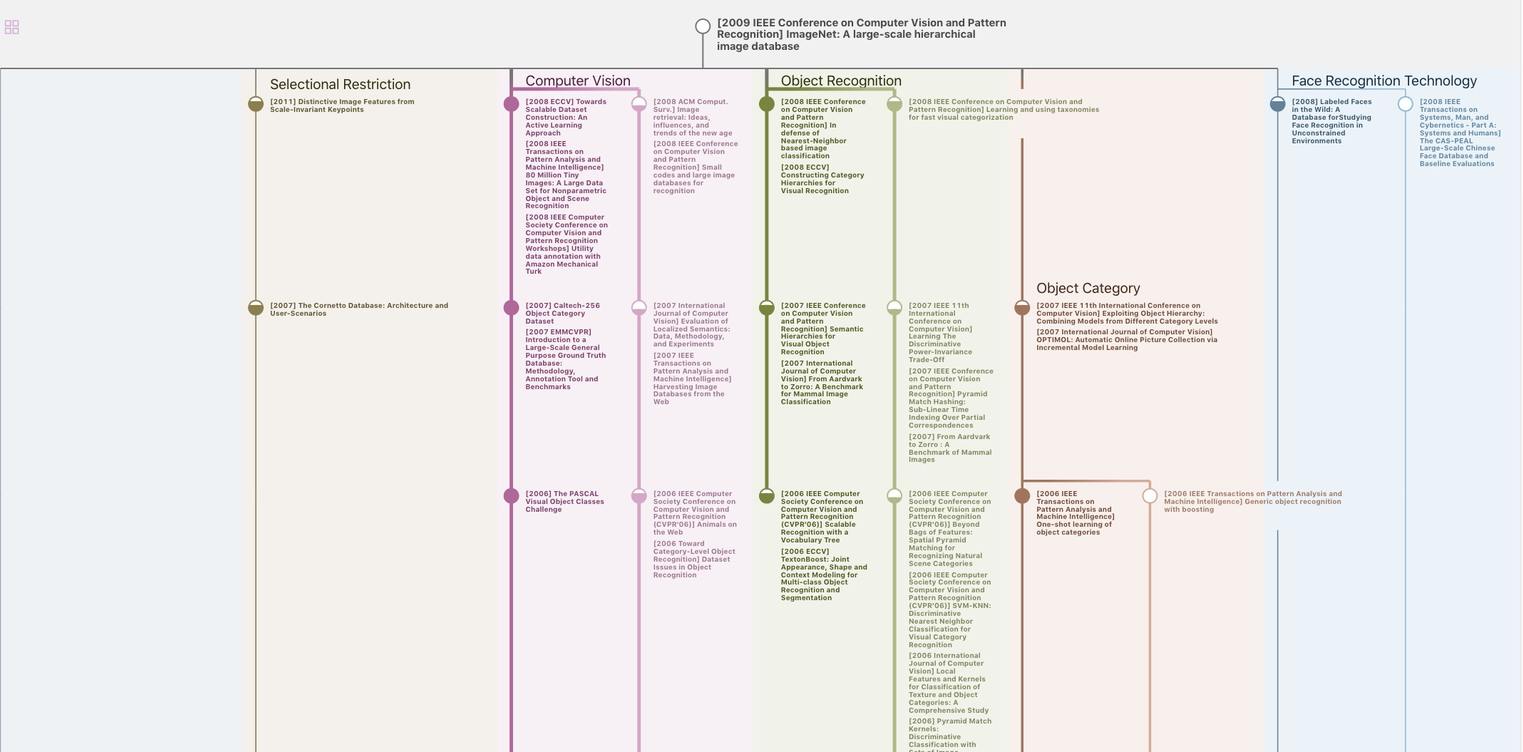
生成溯源树,研究论文发展脉络
Chat Paper
正在生成论文摘要