Adaptive optimal control of linear discrete-time networked control systems with two-channel stochastic dropouts
SIAM JOURNAL ON CONTROL AND OPTIMIZATION(2023)
摘要
This paper investigates the adaptive optimal control problem and proposes fundamentally novel non-model-based approaches for linear discrete-time networked control systems (NCSs) with both sensor and actuator two-channel stochastic dropouts by using directly the data transmitted via communication networks. First, we formulate a modified algebraic Riccati equation parameterized by the system dynamics and the network-induced packet dropouts probabilities, whose solvability is related to a critical arrival probability. To deal with this problem, two model-based reinforcement learning algorithms, policy iteration (PI) and value iteration (VI), are designed with their convergence proofs. To enable the application for NCSs with unknown system dynamics, two novel online PI and VI algorithms are designed. These algorithms develop a new theoretical framework to solve the Bellman function with stochastic dropouts by using directly the data transmitted via networks. Furthermore, a bilevel learning algorithm is proposed to approximate the critical arrival probability. Last but not least, an extension of the developed online VI algorithm is presented for stochastic systems with both unmeasurable noises and stochastic dropouts.
更多查看译文
关键词
reinforcement learning,adaptive optimal control,modified algebraic Riccati equa-tion,communication networks
AI 理解论文
溯源树
样例
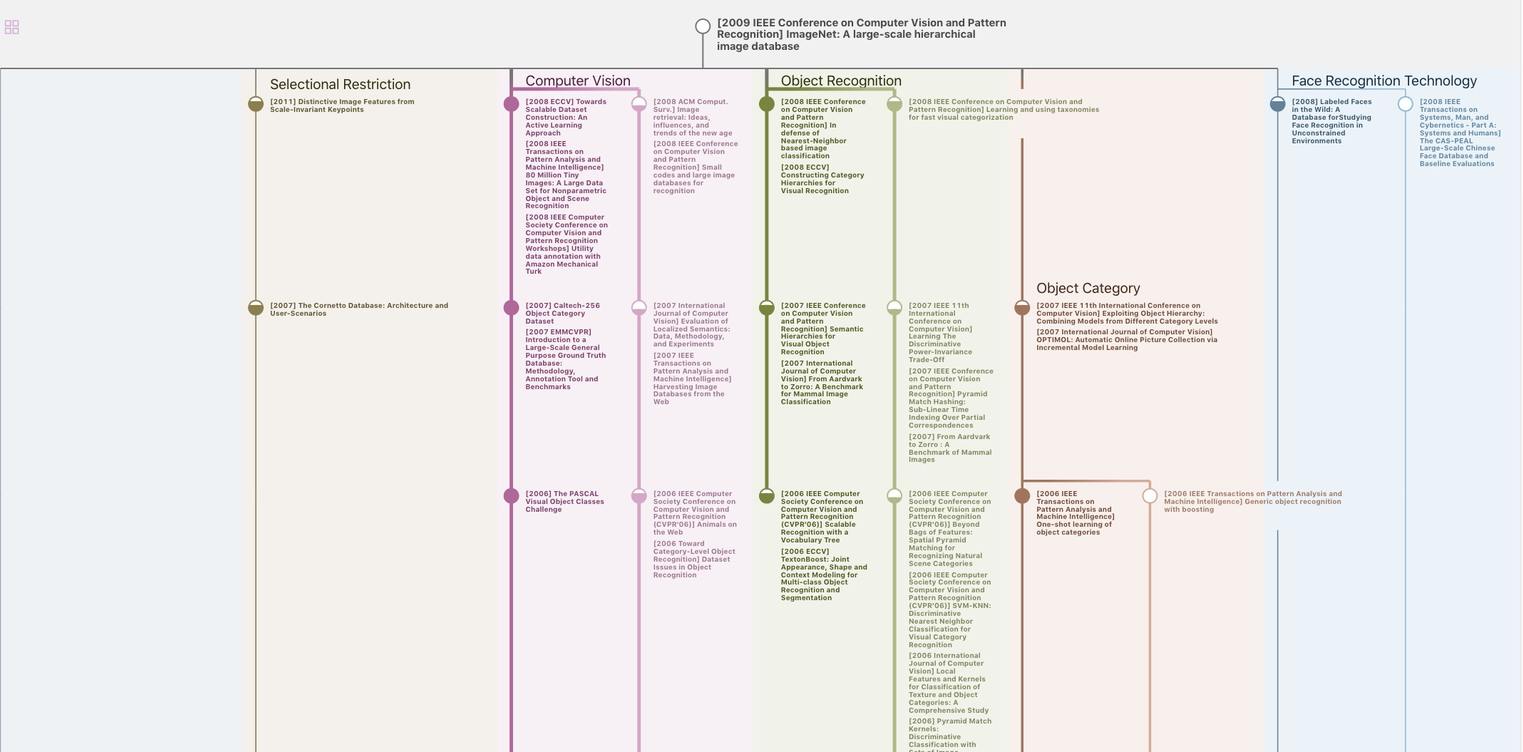
生成溯源树,研究论文发展脉络
Chat Paper
正在生成论文摘要