Confidence-based interactable neural-symbolic visual question answering
NEUROCOMPUTING(2024)
摘要
Visual question answering (VQA) task demands proficiency in processing multi-modal information, and the ability to reason effectively using the information. One promising method for this task is neural-symbolic (NS) learning, which leverages the strengths of both neural network (NN) learning and symbolic reasoning to achieve efficient VQA. However, current NS approaches do not account for the uncertain nature of NN learning and can only provide a single answer to a question without any indication of its confidence, thereby limiting their ability to handle incorrect reasoning. To address this limitation, we propose a confidence based neural-symbolic (CBNS) approach, which evaluates the confidence of the NN inferences based on uncertainty quantification and makes confidence-based reasoning. The proposed approach comprises three main components: (1) a probabilistic question parser that generates multiple program candidates, each with a corresponding confidence evaluation; (2) a probabilistic scene perception module that provides object-based scene representation and confidence evaluations for each attribute of objects in an image; and (3) a confidence based program executor that provides answers with confidence evaluations throughout the inference process by leveraging the confidence evaluations of the scene representation and programs. Additionally, we present a data augmentation method to improve the training efficiency of NS learning. The proposed approach allows user interactions and feedback on the weak links based on confidence evaluations. Experiments on CLEVR and GQA datasets demonstrate that the proposed approach was effective in identifying the correctness of predictions and led to a promising performance improvement with a significantly reduced computation cost.
更多查看译文
关键词
Confidence-based neural-symbolic methods,Interactable neural-symbolic methods,Visual question answering
AI 理解论文
溯源树
样例
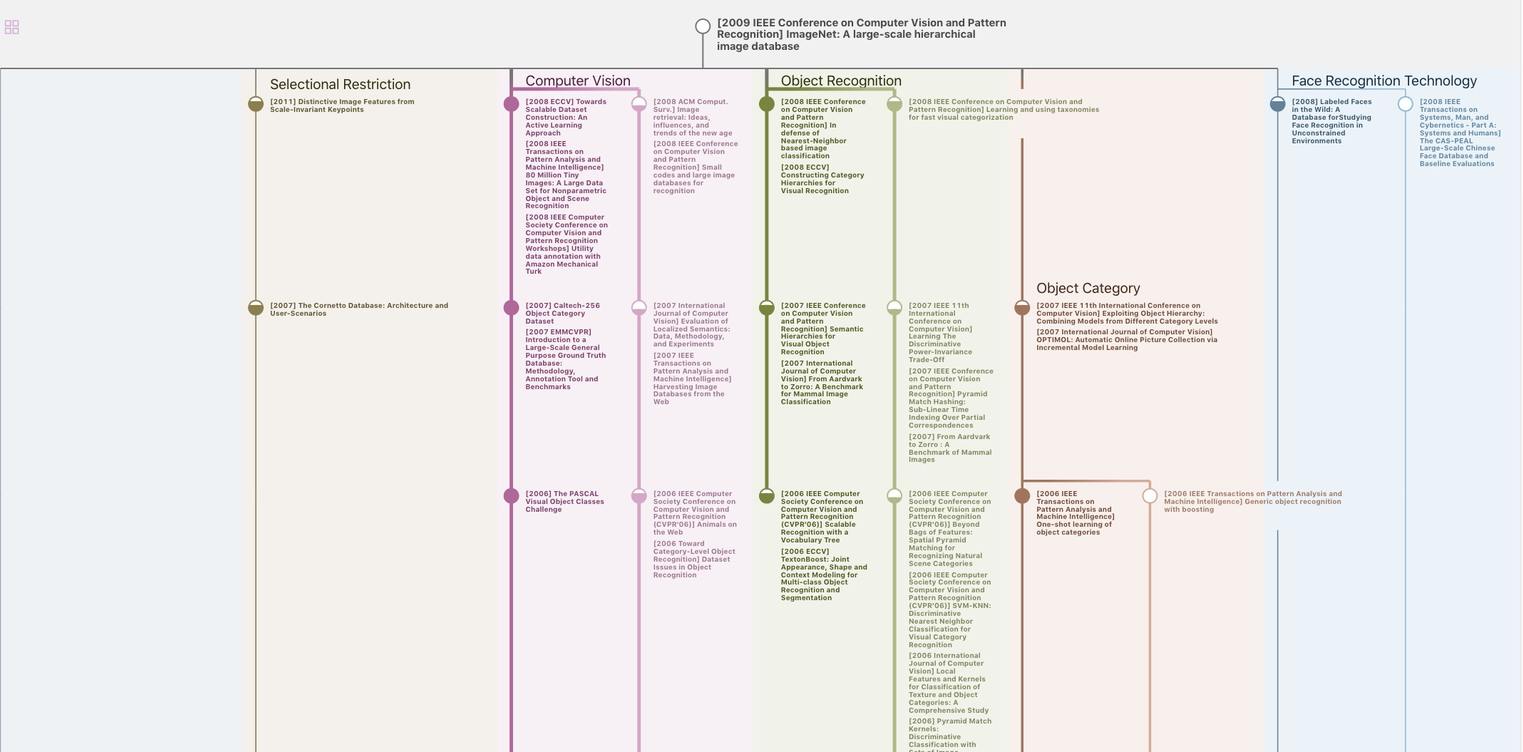
生成溯源树,研究论文发展脉络
Chat Paper
正在生成论文摘要