An adversarial training method for text classification
JOURNAL OF KING SAUD UNIVERSITY-COMPUTER AND INFORMATION SCIENCES(2023)
摘要
Text classification is an emerging topic in the field of text data mining, but the current methods of deducing sentence polarity have two major shortcomings: on the one hand, there is currently a lack of a large and well-curated corpus; on the other hand, current solutions based on deep learning are particularly vulnerable to attacks from adversarial samples. To overcome the limitations above, we propose an adversarial training method HNN-GRAT (Hierarchical Neural Network and Gradient Reversal) for text classification. Firstly, A Robustly Optimized BERT Pretraining Approach (RoBERTa) pretraining model is used to extract text features and feature gradient information; secondly, the original gradient information is passed through the gradient reversal layer designed to obtain the inverted gradient information; finally, the original gradient information and the inverted gradient information are fused to obtain the new gradient of the model. HNN-GRAT method are tested on three real datasets and five attack methods, compared with RoBERTa pretraining model, HNN-GRAT improves the robustness accuracy and reduces the probability of the model being attacked. In addition, using six text defense methods, HNN-GRAT achieves the best Boa and Succ (Such as, DeepWordBug attack, for AGNEWS, IMDB, SST-2 datasets, with an improvement of Boa up to 41.50%, 67.50%, 28.15% and Succ drop to 55.90%, 27.45%, 69.89%, respectively). (c) 2023 The Author(s). Published by Elsevier B.V. on behalf of King Saud University. This is an open access article under the CC BY-NC-ND license (http://creativecommons.org/licenses/by-nc-nd/4.0/).
更多查看译文
关键词
Gradient reversal,Adversarial training,Text classification,Neural networks,Adversarial sample
AI 理解论文
溯源树
样例
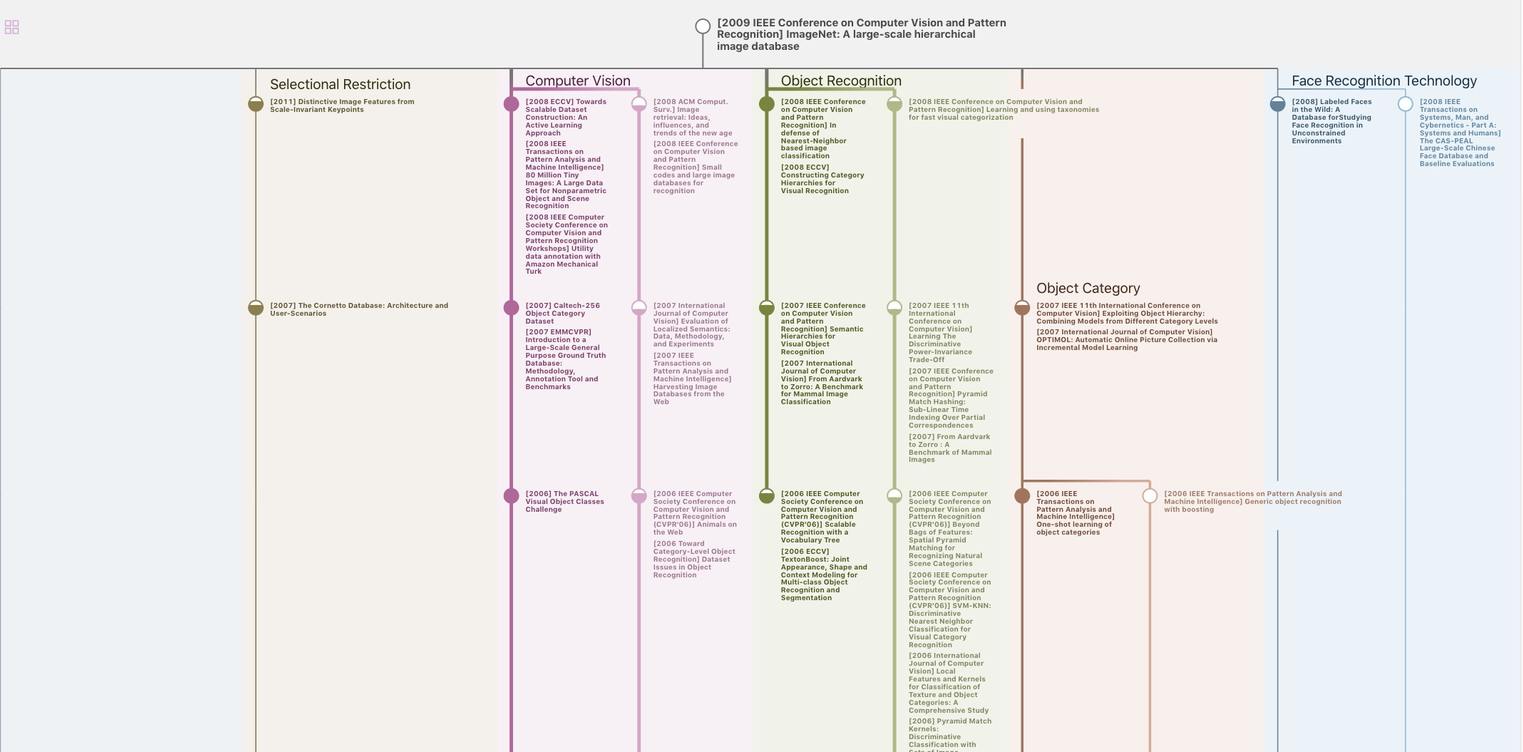
生成溯源树,研究论文发展脉络
Chat Paper
正在生成论文摘要