Exemplar Models with Missing Feedback: A Theoretical Analysis
crossref(2023)
摘要
In many real-life settings, feedback is only available for cases decision makers accept. The selection bias generated by such missing feedback poses problems for data-driven learning algorithms, such as exemplar based models that rely on the available data to make predictions about new cases. How can exemplar algorithms deal with such biased data? Elwin et al. (2007) argued that people augment the available data with imputed missing observations: people code rejected cases, for which no feedback is available, as if the feedback was negative. It is not intuitively obvious whether relying on such internally generated feedback is sensible or leads to bias. To examine this, we analyze how exemplar learning algorithms work when missing data is imputed. We show, using simulations, mathematical analysis, and empirical data, that imputing a fictive outcome to rejected cases (Elwin et al., 2007) is an adaptive strategy: it maximizes total expected reward. Imputation helps because it compensates for the optimistic bias exemplar models suffer from when they generalize from the available data, which is subject to a selection bias. We also show that such exemplar model with imputation can perform almost as well as the optimal Bayesian approach and can provide a simple and computationally efficient way to approximate the Bayesian approach for data with a selection bias.
更多查看译文
AI 理解论文
溯源树
样例
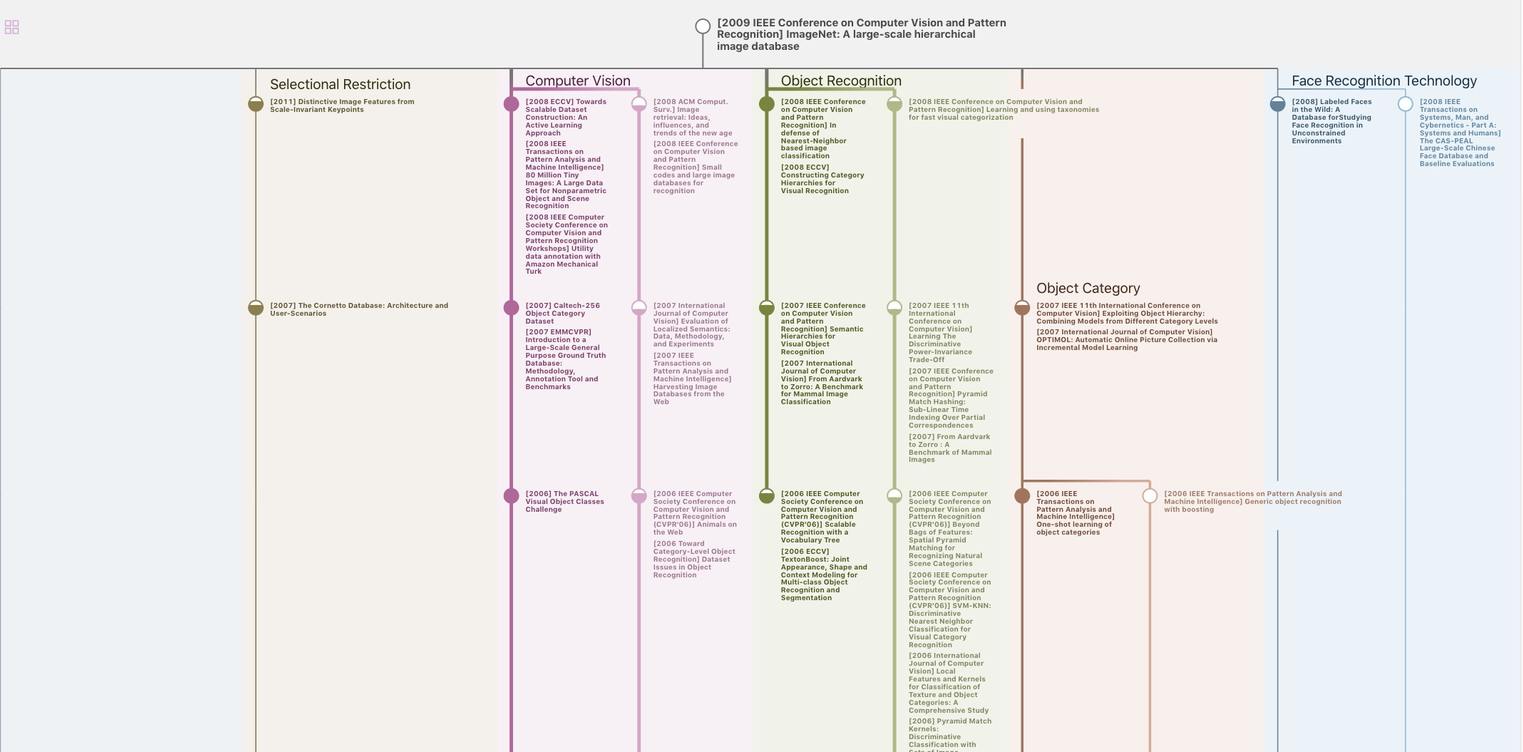
生成溯源树,研究论文发展脉络
Chat Paper
正在生成论文摘要