Unsupervised Anomaly Detection and Diagnosis in Power Electronic Networks: Informative Leverage and Multivariate Functional Clustering Approaches.
IEEE Trans. Smart Grid(2024)
摘要
We propose a novel unsupervised anomaly detection and diagnosis algorithm in power electronic networks. Since most anomaly detection and diagnosis algorithms in the literature are based on supervised methods that can hardly be generalized to broader scenarios, we propose unsupervised algorithms. Our algorithm extracts the Time-Frequency Domain (TFD) features from the three-phase currents and three-phase voltages of the point of coupling (PCC) nodes to detect anomalies and distinguish between different types of anomalies, such as cyber-attacks and physical faults. To detect anomalies through TFD features, we propose a novel Informative Leveraging for Anomaly Detection (ILAD) algorithm. The proposed unsupervised ILAD algorithm automatically extracts noise-reduced anomalous signals, resulting in more accurate anomaly detection results than other score-based methods. To assign anomaly types for anomaly diagnosis, we apply a novel Multivariate Functional Principal Component Analysis (MFPCA) clustering method. Unlike the deep learning methods, the MFPCA clustering method does not require labels for training and provides more accurate results than other deep embedding-based clustering approaches. Furthermore, it is even comparable to supervised algorithms in both offline and online experiments. To the best of our knowledge, the proposed unsupervised framework accomplishing anomaly detection and anomaly diagnosis tasks is the first of its kind in power electronic networks.
更多查看译文
关键词
Anomaly Detection,Anomaly Diagnosis,Leverage Score,Multivariate Principal Component Analysis based Clustering,Power Electronic Networks
AI 理解论文
溯源树
样例
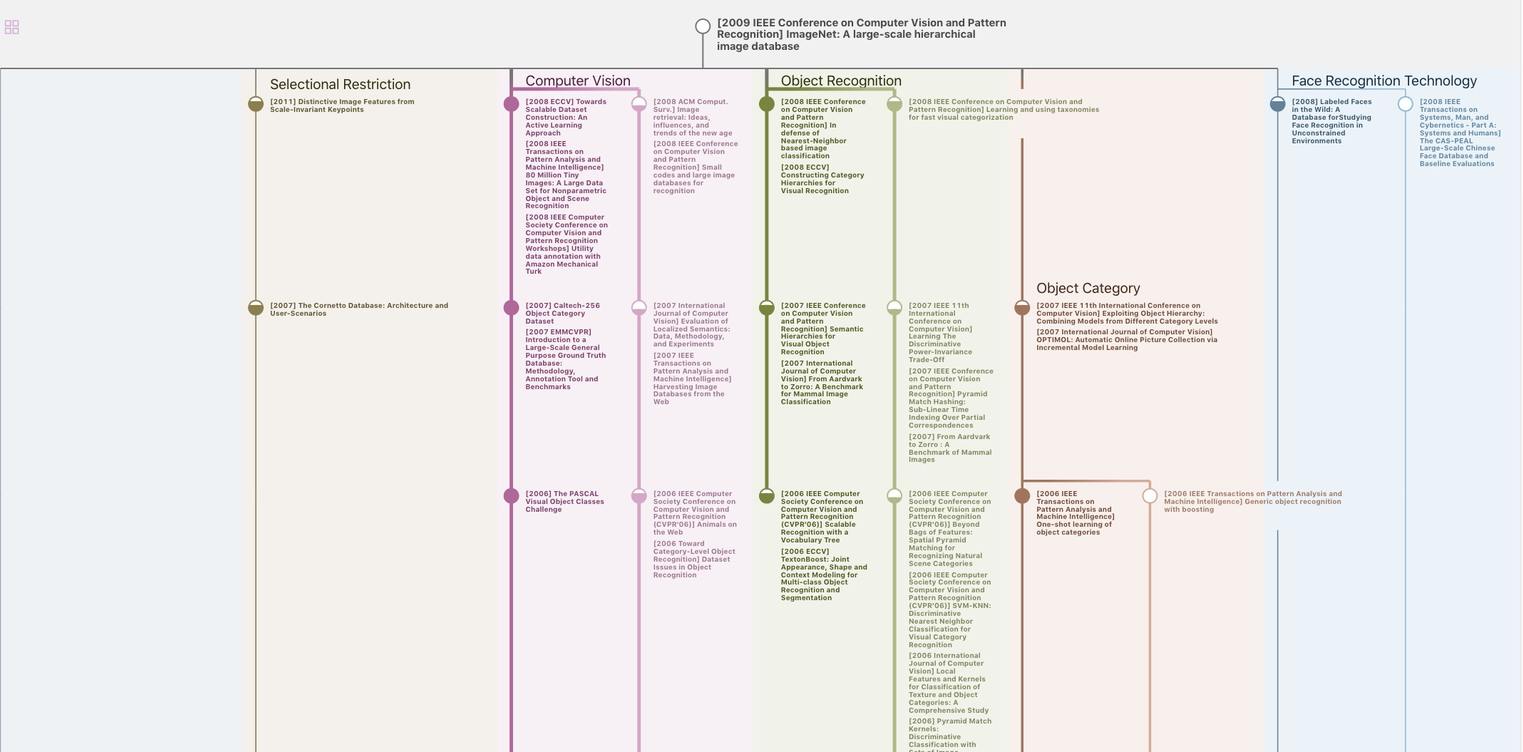
生成溯源树,研究论文发展脉络
Chat Paper
正在生成论文摘要