A Lightweight Binarized Convolutional Neural Network Model for Small Memory and Low-Cost Mobile Devices
Mobile Information Systems(2023)
摘要
In recent years, the high cost of implementing deep neural networks due to their large model size and parameter complexity has made it a challenging problem to design lightweight models that reduce application costs. The existing binarized neural networks suffer from both the large memory occupancy and the big number of trainable params they use. We propose a lightweight binarized convolutional neural network (CBCNN) model to address the multiclass classification/identification problem. We use both binary weights and activation. We show experimentally that a model using only 0.59 M trainable params is sufficient to reach about 92.94% accuracy on the GTSRB dataset, and it has similar performances compared to other methods on MNIST and Fashion-MNIST datasets. Accordingly, most arithmetic operations with bitwise operations are simplified, thus both used memory size and memory accesses are reduced by 32 times. Moreover, the color information was removed, which also reduced drastically the training time. All these together allow our architecture to run effectively and in real time on simple CPUs (rather than GPUs). Through the results we obtained, we show that despite simplifications and color information removal, our network achieves similar performances compared to classical CNNs with lower costs in both in training and embedded deployment.
更多查看译文
关键词
small memory,mobile devices,low-cost
AI 理解论文
溯源树
样例
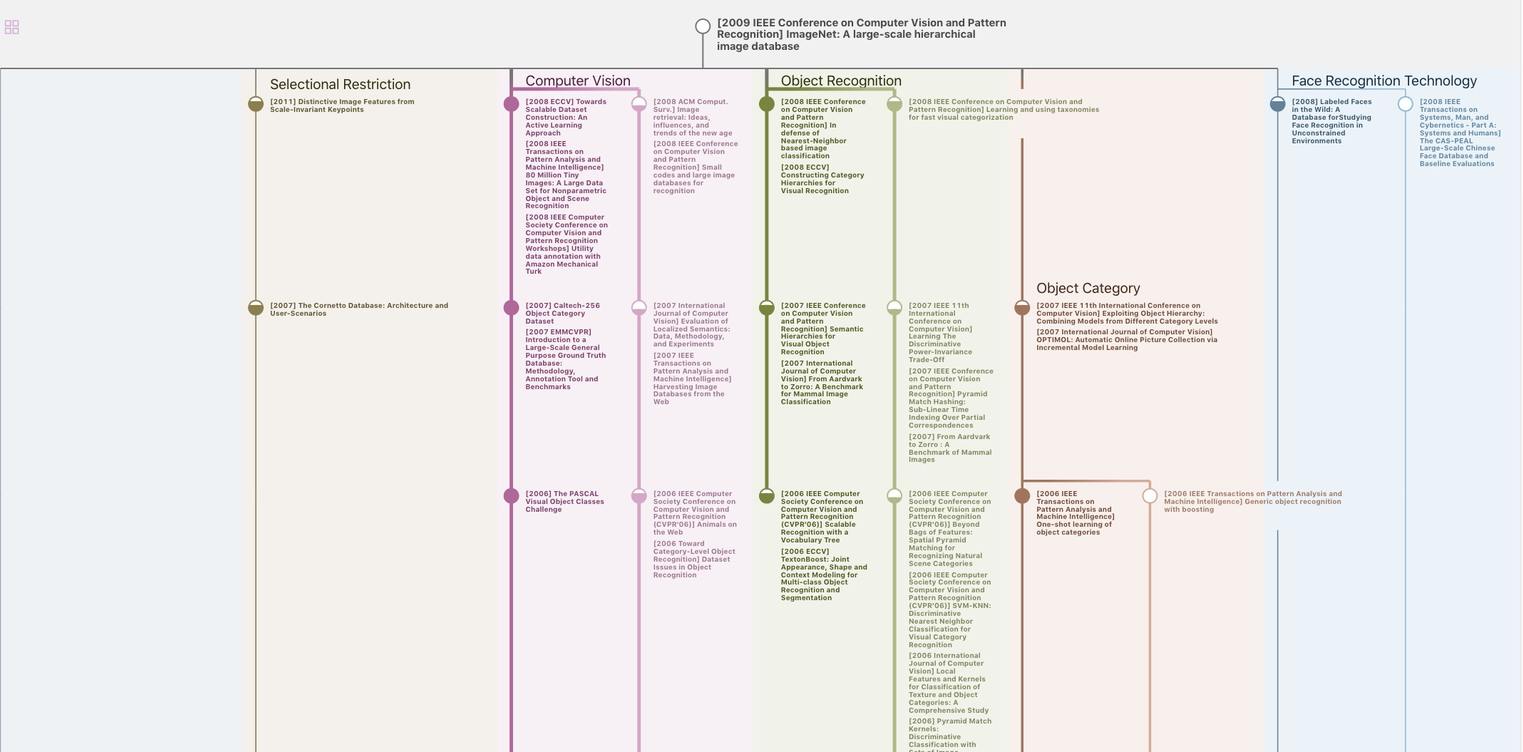
生成溯源树,研究论文发展脉络
Chat Paper
正在生成论文摘要