Efficient Mapping Between Void Shapes and Stress Fields Using Deep Convolutional Neural Networks With Sparse Data
Volume 2: 43rd Computers and Information in Engineering Conference (CIE)(2023)
摘要
Abstract Establishing fast and accurate structure-to-property relationships is an important component in the design and discovery of materials. Physics-based simulation models like the finite element method (FEM) are often used to predict deformation, stress, and strain fields as a function of material microstructure in material and structural systems. Such models may be computationally expensive and time intensive if the underlying physics of the system is complex. This limits their application to solve inverse design problems and identify structures that maximizes performance. In such scenarios, surrogate models are employed to make the forward mapping efficient. Still, the high dimensionality of the input microstructure and the output field of interest may render them inefficient, especially when dealing with sparse data. Deep convolutional neural network (CNN) based surrogate models have been typically found to be very useful in handling such high-dimensional problems. In this paper, the system under study is a single ellipsoidal void structure under a uniaxial tensile load represented by a linear elastic FEM model. We consider two deep CNN architectures, a modified Convolutional Autoencoder (CAE) framework with a fully connected bottleneck and a UNet Convolutional Neural Network (CNN), and compare their accuracy in predicting the Von Mises stress field for any given input void shape in the FEM model. A sensitivity analysis is also performed using the two methods, where the variation in the prediction accuracy on unseen test data is studied with the increasing number of training samples from 20 to 100.
更多查看译文
AI 理解论文
溯源树
样例
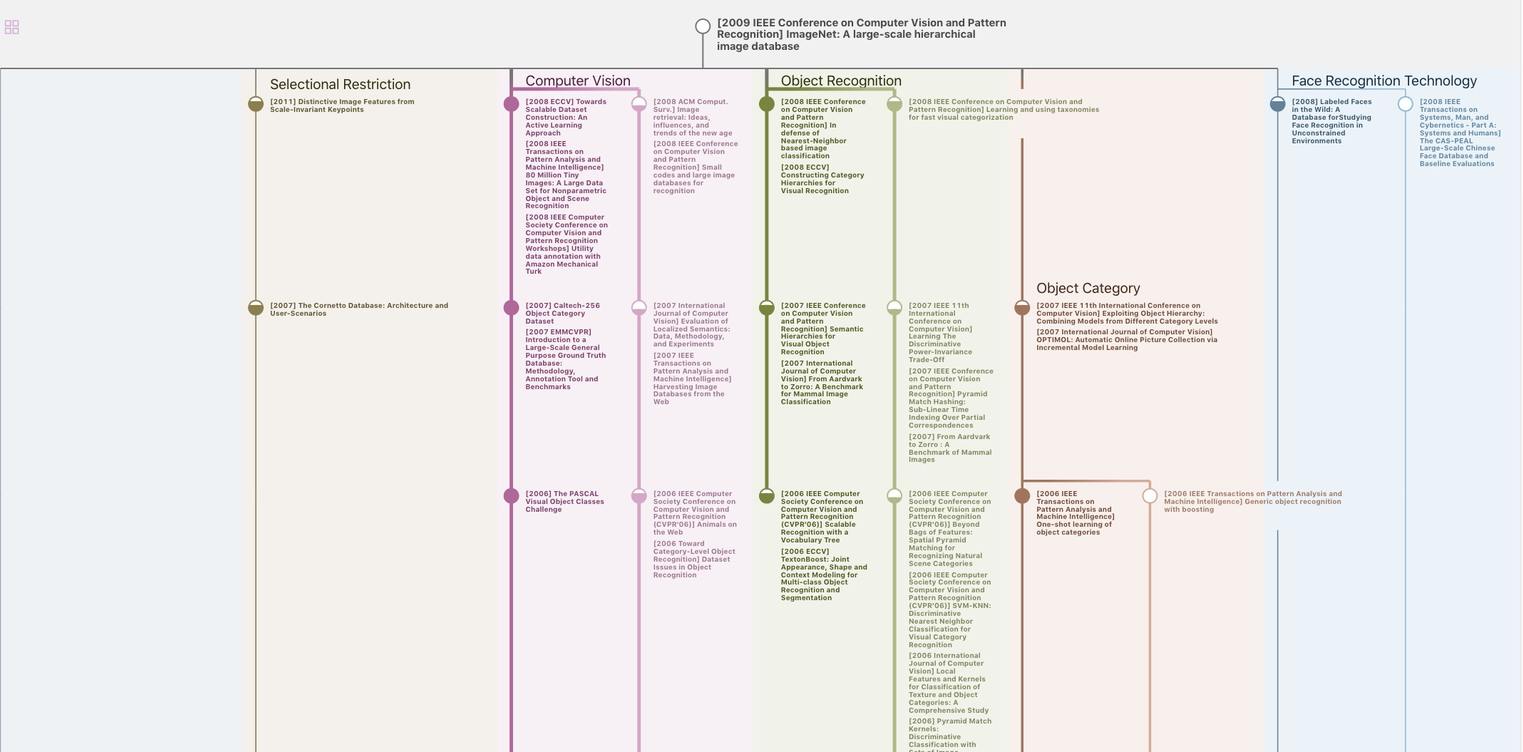
生成溯源树,研究论文发展脉络
Chat Paper
正在生成论文摘要