Physics-Informed Neural Networks for Fault Slip Monitoring: Simulation, Frictional Parameter Estimation, and Prediction on Slow Slip Events in a Spring-Slider System
JOURNAL OF GEOPHYSICAL RESEARCH-SOLID EARTH(2023)
摘要
The episodic transient fault slips called slow slip events (SSEs) have been observed in many subduction zones. These slips often occur in regions adjacent to the seismogenic zone during the interseismic period, making monitoring SSEs significant for understanding large earthquakes. Various fault slip behaviors, including SSEs and earthquakes, can be explained by the spatial heterogeneity of frictional properties on the fault. Therefore, estimating frictional properties from geodetic observations and physics-based models is crucial for fault slip monitoring. In this study, we propose a Physics-Informed Neural Network (PINN)-based new approach to simulate fault slip evolutions, estimate frictional parameters from observation data, and predict subsequent fault slips. PINNs, which integrate physical laws and observation data, represent the solution of physics-based differential equations. As a first step, we validate the effectiveness of the PINN-based approach using a simple single-degree-of-freedom spring-slider system to model SSEs. As a forward problem, we successfully reproduced the temporal evolution of SSEs using PINNs and obtained implications on how to choose the appropriate collocation points by analyzing the residuals of physics-based differential equations. As an inverse problem, we estimated the frictional parameters from synthetic observation data and demonstrated the ability to obtain accurate values regardless of the choice of first-guess values. Furthermore, we discussed the potential of the predictability of the subsequent fault slips using limited observation data, taking into account uncertainties. Our results indicate the significant potential of PINNs for fault slip monitoring. Slow slip events (SSEs), which are fault slips characterized by slower velocity and longer duration compared to earthquakes, have been observed in many subduction zones. Monitoring SSEs is important for understanding large earthquakes because they occur adjacent to areas where significant earthquakes could potentially occur. Different types of fault slips, including SSEs and earthquakes, can be explained by distinct frictional properties on the fault. These frictional properties can be estimated from physical laws of fault slip and observed crustal deformation. In this study, we propose a new machine-learning based approach for fault slip monitoring. We employed Physics-Informed Neural Networks (PINNs), which simultaneously learn the physical laws and data, to simulate fault slip, estimate the frictional parameters, and predict subsequent fault slip. As a first step, we utilized a single-degree-of-freedom spring-slider system, which is the simplest physical model to simulate SSEs. We successfully simulated SSEs, estimated frictional properties from synthetic observation data, and discussed the potential for fault slip prediction. Our results suggest the significant potential of PINNs for fault slip monitoring. We propose Physics-Informed Neural Networks (PINNs) for fault slip simulation, frictional parameter estimation, and slip predictionPINNs can reproduce slow slip events in a spring-slider system and estimate the frictional parameters from synthetic observation dataWe investigated the potential of the predictability of subsequent fault slips from limited observation data including uncertainties
更多查看译文
AI 理解论文
溯源树
样例
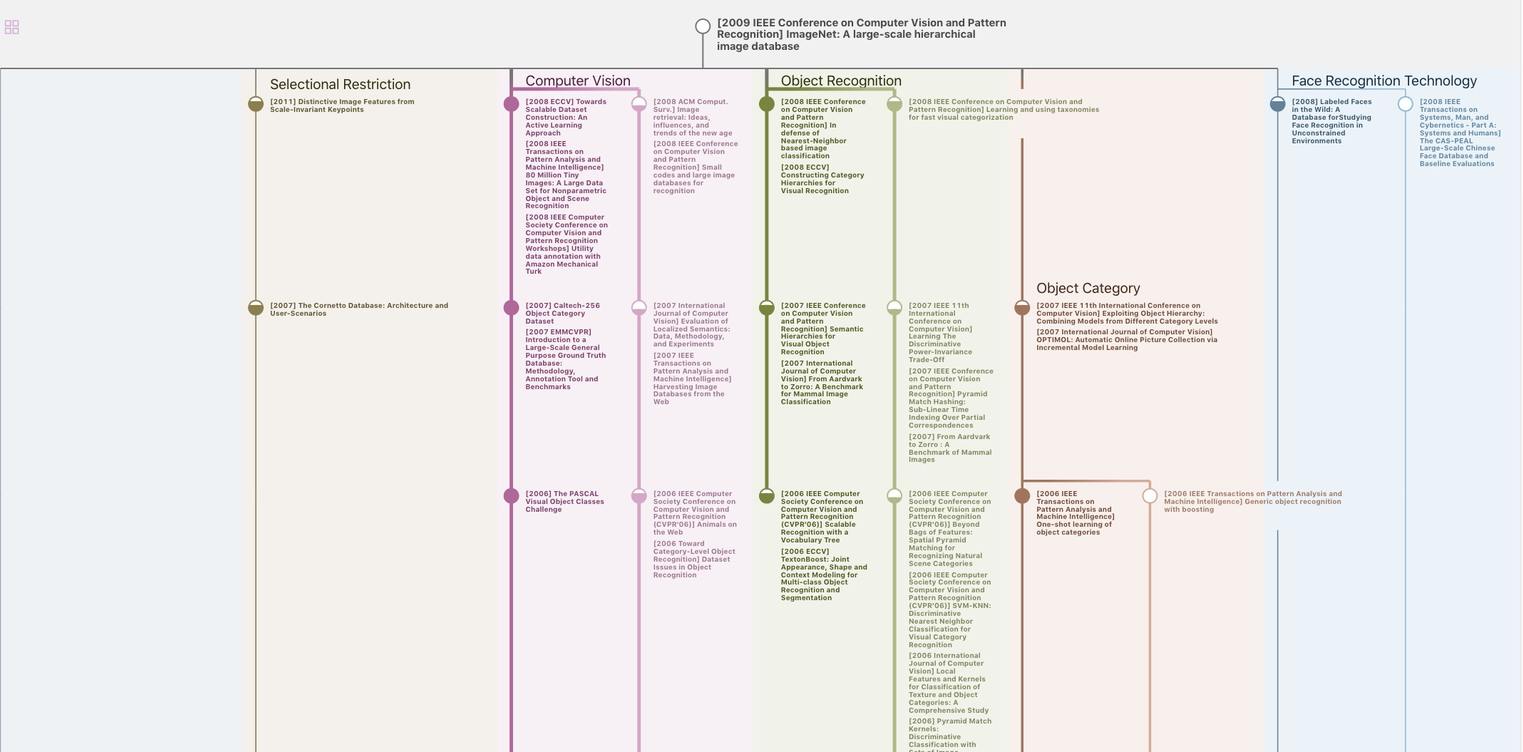
生成溯源树,研究论文发展脉络
Chat Paper
正在生成论文摘要