Embedded machine learning of IoT streams to promote early detection of unsafe environments
INTERNET OF THINGS(2024)
摘要
Indoor particulate matter (PM) are small solid and liquid particles present in the air, and its monitoring is one of the key challenges regarding workplace safety because of its impact on human health. To address this issue, the Internet of Things (IoT) paradigm allows the implementation of hyperlocal monitoring systems, typically using traditional cloud architectures, which can be enhanced using edge computing architectures. For this reason, we propose an IoTEdge-Cloud architecture for a platform which promotes the early detection of unsafe environments through machine learning, composed of a sensing layer that collects all the data, an edge layer that performs the artificial intelligence tasks and a cloud layer orchestrating. This architecture is based on the FogFlow framework and the FIWARE components. Our solution proposes an embedded model that can predict the occurrence of PM values higher than the recommended ones-according to the Occupational Safety and Health Administration (OSHA) indicators-with an 87 % of accuracy and a reduction of latency of 26 %. Our platform is innovative because it is based on the FogFlow framework for edge computing and supported by the Smart Spot device validated in a field test. This step is missing from similar state-of-the-art platforms. Thus, we believe that this work contributes to demonstrating the usefulness of AIoT to monitor workplace safety and make trustable predictions, avoiding risky environments.
更多查看译文
关键词
Embedded machine learning,Edge computing,Indoor air quality monitoring,Real-time systems,Sensor analysis,Data management and analytics
AI 理解论文
溯源树
样例
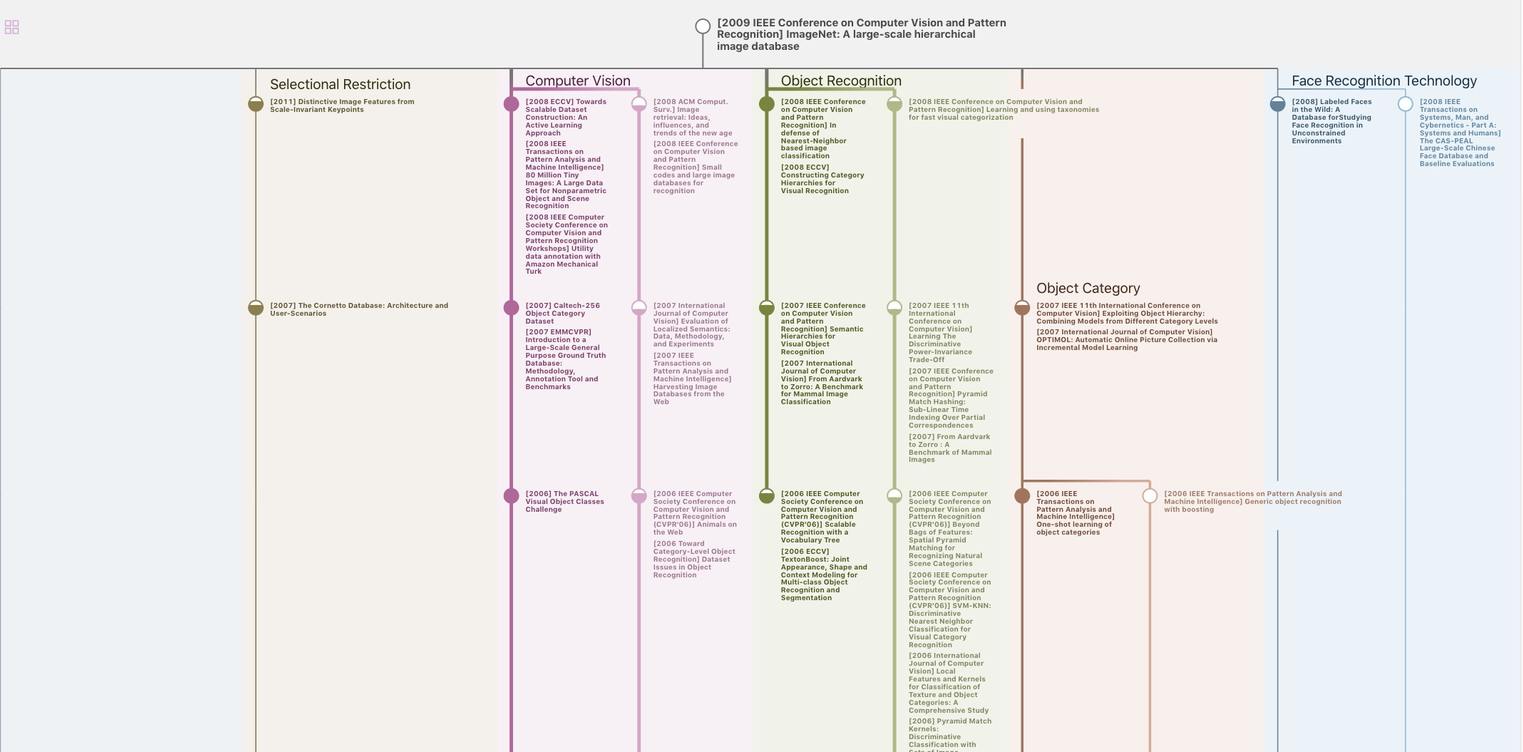
生成溯源树,研究论文发展脉络
Chat Paper
正在生成论文摘要