Circularly Polarized Antenna Array Synthesis Based on Machine-Learning-Assisted Surrogate Modeling
IEEE TRANSACTIONS ON ANTENNAS AND PROPAGATION(2024)
摘要
In this article, a machine-learning-assisted surrogate modeling (MLSM) method is presented to facilitate the circularly polarized (CP) antenna array synthesis with mutual coupling considered. In the premise that the vectorial active element pattern (Vec. AEP) of an array element is only susceptible to its surrounding region, the MLSM, primarily comprised of two deep neural networks (DNNs), is employed to predict the Vec. AEPs of all the array elements sequentially based on their corresponding subarray features. Subsequently, the co- polarization (CoP) pattern, cross-polarization (XP) pattern, and axial ratio (AR) of the CP array are computed accordingly. In this manner, the MLSM enables the fast performance prediction of arrays with arbitrary geometries and adds an extra degree of freedom (DoF), namely element position, to CP antenna array design. The trained MLSM is also employed to predict the performances of arrays with any given element numbers exploiting the ideology of heterogeneous transfer learning (HTL). To verify the effectiveness of the proposed framework, the MLSM is employed to predict the performance of arrays in several CP array syntheses. Numerical results demonstrate that the proposed MLSM is a feasible and efficient method for the fast prediction of CP arrays with arbitrary geometries and any given element numbers.
更多查看译文
关键词
Circularly polarized (CP) antenna array,cross-polarization (XP) level,deep neural network (DNN),machine-learning (ML),shaped pattern synthesis,transfer learning,vectorial active element pattern (Vec AEP)
AI 理解论文
溯源树
样例
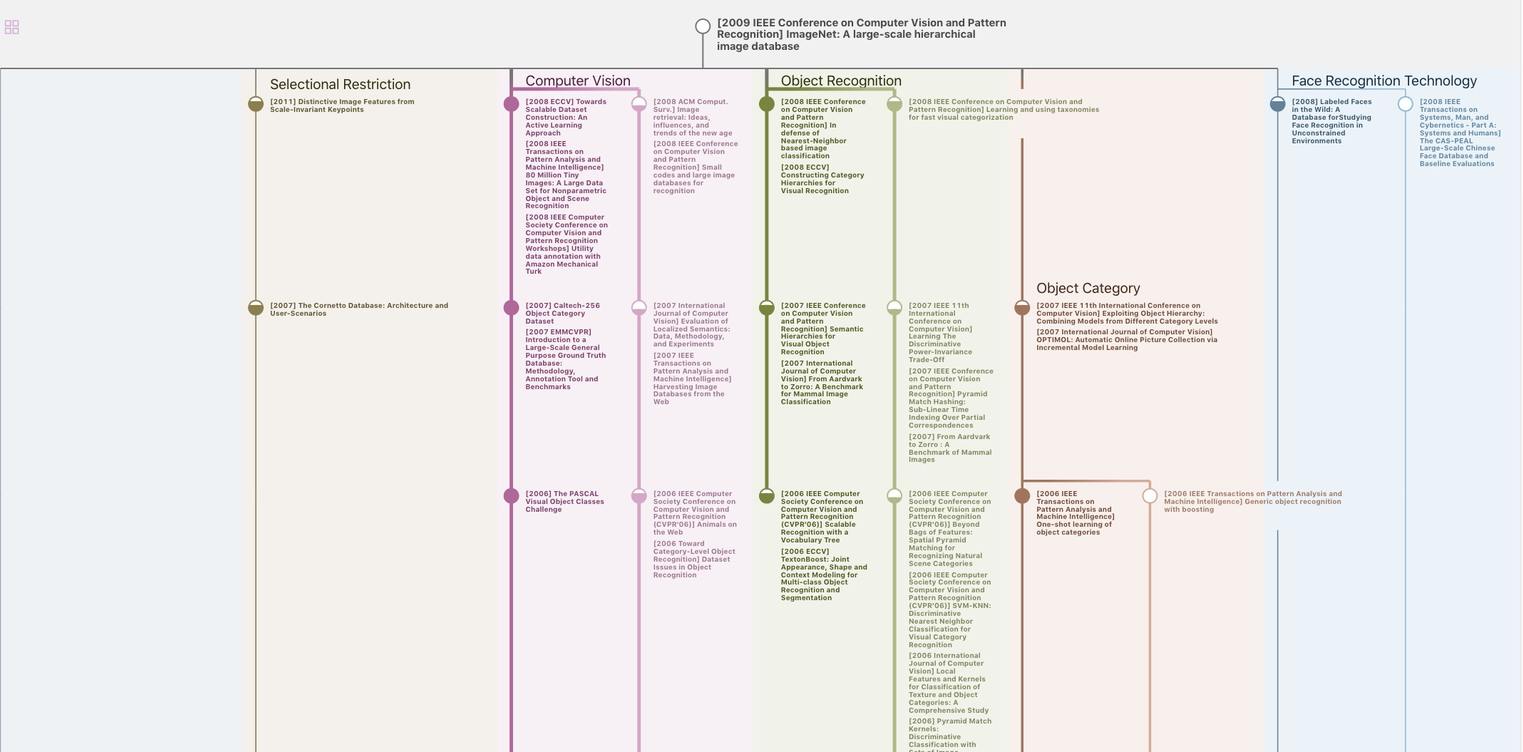
生成溯源树,研究论文发展脉络
Chat Paper
正在生成论文摘要