LiteEnhanceNet: A lightweight network for real-time single underwater image enhancement
EXPERT SYSTEMS WITH APPLICATIONS(2024)
摘要
In recent years, the field of underwater image enhancement has evolved from physics-based approaches to various neural network-based methods, all of which have achieved promising results. However, these state-ofthe-art algorithms often demand substantial computational resources, posing challenges for real-time applications and deployment on underwater mobile devices. To tackle this issue, this paper introduces a lightweight network called LiteEnhanceNet for single underwater image enhancement. Specifically, the network adopts depthwise separable convolution as the main building block to reduce computational complexity. The one-shot aggregation connection is employed to effectively extract features from both low-level and intermediate layers. Additionally, appropriate activation functions and the squeeze-and-excitation module are incorporated at suitable positions within the network to reduce computational complexity. Quantitative and qualitative experiments demonstrate that the proposed network achieves superior running speed while maintaining enhancement performance compared to the current state-of-the-art techniques. Our code is available at https://github.com/zhang song1213/LiteEnhanceNet.
更多查看译文
关键词
Underwater image enhancement,Depthwise separable convolution,One-shot aggregation connection,Lightweight
AI 理解论文
溯源树
样例
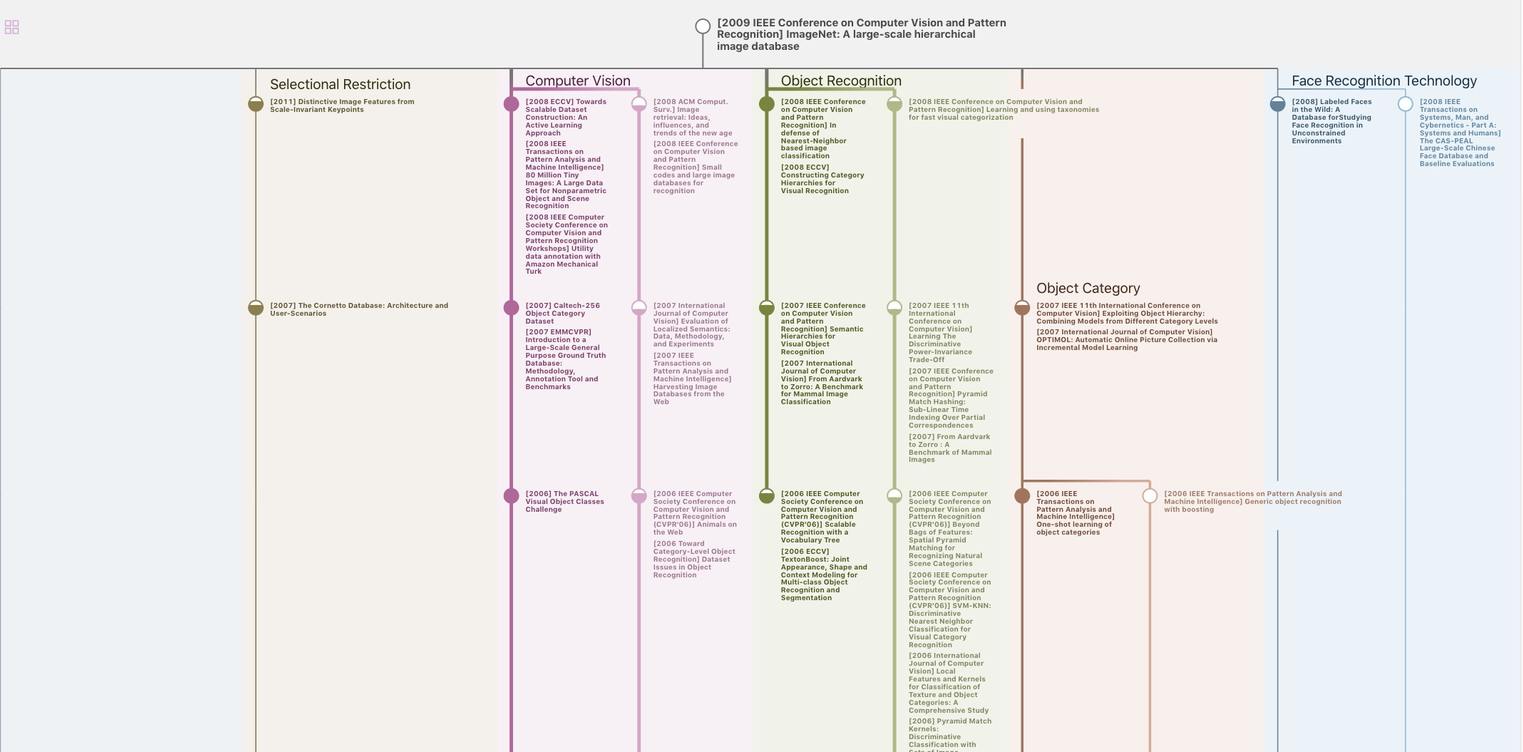
生成溯源树,研究论文发展脉络
Chat Paper
正在生成论文摘要