A survey on federated learning: a perspective from multi-party computation
Frontiers of Computer Science(2024)
摘要
Federated learning is a promising learning paradigm that allows collaborative training of models across multiple data owners without sharing their raw datasets. To enhance privacy in federated learning, multi-party computation can be leveraged for secure communication and computation during model training. This survey provides a comprehensive review on how to integrate mainstream multi-party computation techniques into diverse federated learning setups for guaranteed privacy, as well as the corresponding optimization techniques to improve model accuracy and training efficiency. We also pinpoint future directions to deploy federated learning to a wider range of applications.
更多查看译文
关键词
federated learning,multi-party computation,privacy-preserving data mining,distributed learning
AI 理解论文
溯源树
样例
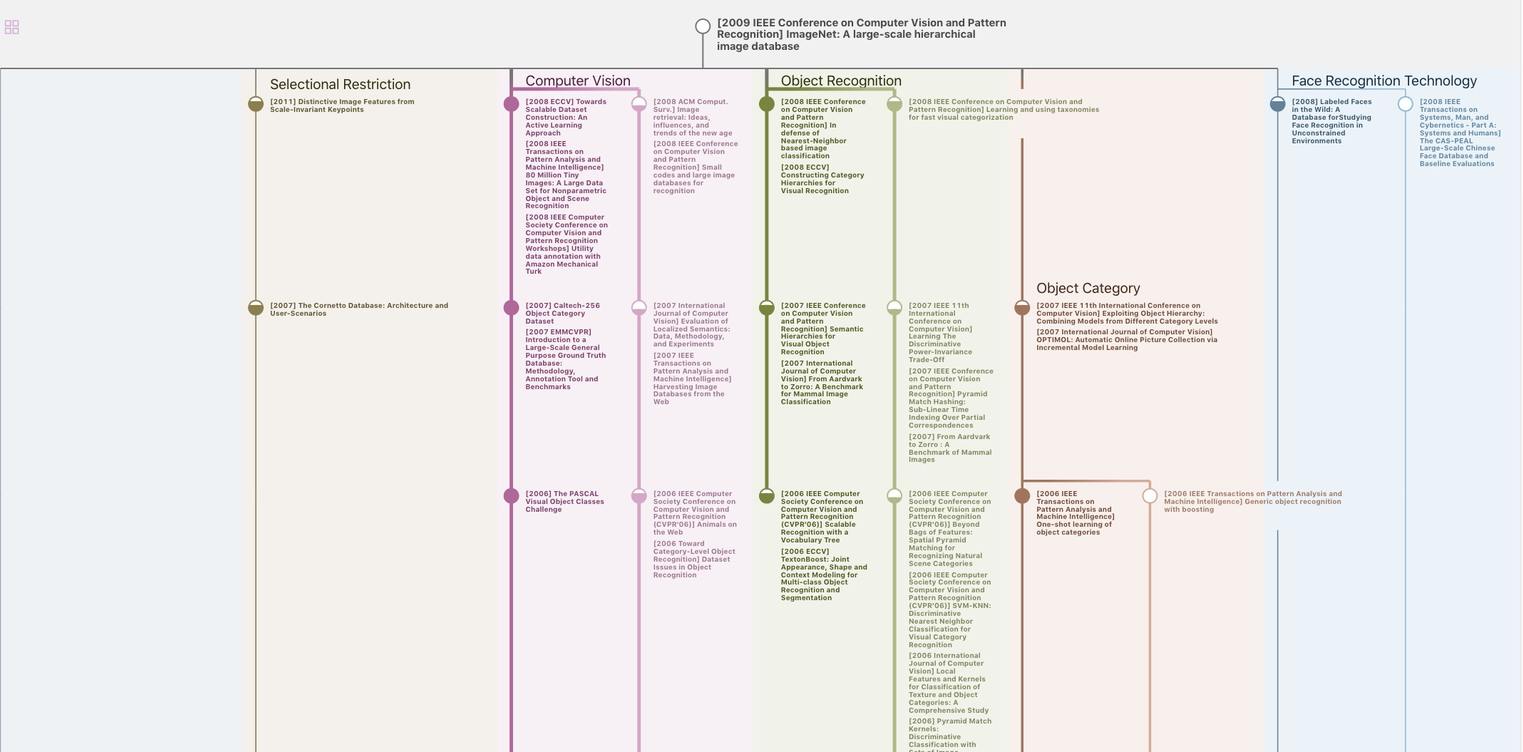
生成溯源树,研究论文发展脉络
Chat Paper
正在生成论文摘要