Localization Exploiting Semantic and Metric Information in Non-static Indoor Environments
Journal of Intelligent and Robotic Systems(2023)
摘要
Mobile robot localization is an important task in navigation and can be challenging, especially in non-static environments as the scene naturally involves movable objects and appearance changes. In this paper, we address the problem of estimating the robot’s pose in non-static environments containing movable objects. We understand as non-static environments, dynamic environments in which objects might be moved or changed their appearance. We propose a probabilistic localization approach that combines metric and semantic information and takes into account both, static and movable objects. We perform a pixel-wise association of depth and semantic data from an RGB-D sensor with a semantically-augmented truncated signed distance field (TSDF) in order to estimate the robot’s pose. The combination of metric and semantic information increases the robustness w.r.t. movable objects and object appearance changes. The experiments conducted in a real indoor environment and a publicly-available dataset suggest that our approach successfully estimates robot pose in non-static environments and they show an improvement compared to robot localization based only on metric or semantic information and compared to a feature-based method.
更多查看译文
关键词
Visual localization,Semantic localization,Dynamic environments,Monte Carlo localization
AI 理解论文
溯源树
样例
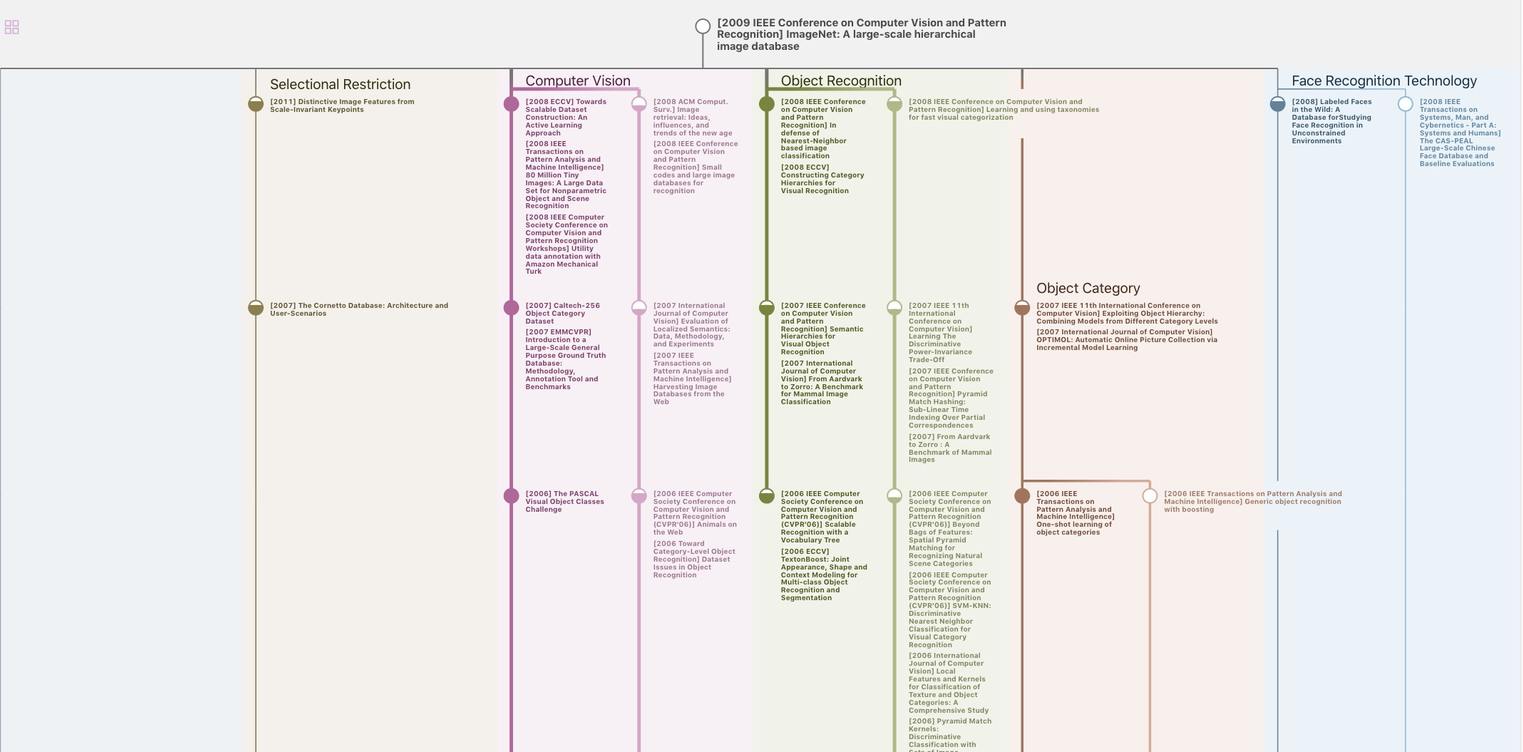
生成溯源树,研究论文发展脉络
Chat Paper
正在生成论文摘要