Graph Multi-Agent Reinforcement Learning for Inverter-Based Active Voltage Control
IEEE TRANSACTIONS ON SMART GRID(2024)
摘要
Active voltage control (AVC) is a widely-used technique to improve voltage quality essential in the emerging active distribution networks (ADNs). However, the voltage fluctuation caused by intermittent renewable energy makes it difficult for traditional voltage control methods to deal with. In this paper, the voltage control problem is formulated as a decentralized partial observable Markov decision process (Dec-POMDP), and a multi-agent reinforcement learning (MARL) algorithm is developed considering each controllable device as an agent. The new formulation aims to adjust the strategies of agents to stabilize the voltage within the specified range and reduce the network loss. To better represent the mutual interaction between the agents, a graph convolutional network (GCN) is introduced. By aggregating the information of adjacent agents, complex latent features are effectively extracted by the GCN, hence promotes the generation of voltage control strategy for the agents. Meanwhile, a barrier function is applied in MARL to ensure the system voltage within a safe operation range. Comparative studies are conducted with traditional voltage control and other MARL methods on IEEE 33-bus and 141-bus systems, which demonstrate the performance of the proposed approach.
更多查看译文
关键词
Automatic voltage control,Topology,Power system stability,Training,Reinforcement learning,Distribution networks,Reactive power,Active voltage control,multi-agent reinforcement learning,graph convolutional network,barrier function,distribution network
AI 理解论文
溯源树
样例
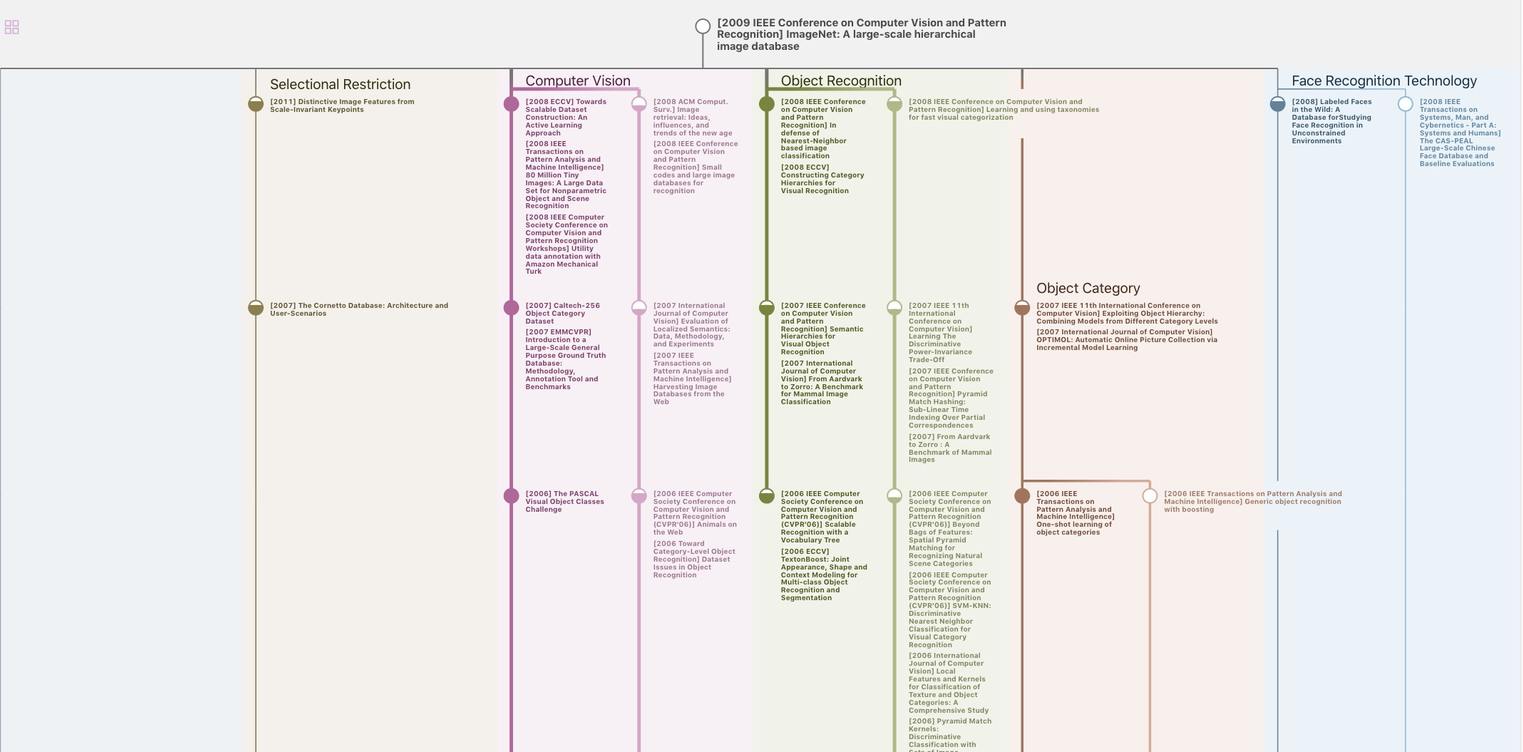
生成溯源树,研究论文发展脉络
Chat Paper
正在生成论文摘要