The 2-layer elasto-visco-plastic rheological model for the material parameter identification of bone tissue extended by a damage law
JOURNAL OF THE MECHANICAL BEHAVIOR OF BIOMEDICAL MATERIALS(2024)
摘要
The response of bone tissue to mechanical load is complex and includes plastic hardening, viscosity and damage. The quantification of these effects plays a mayor role in bone research and in biomechanical clinical trials as to better understand related diseases. In this study, the damage growth in individual wet human trabeculae subjected to cyclic overloading is quantified by inverse rheological modeling. Therefore, an already published rheological material model, that includes linear elasticity, plasticity and viscosity is extended by a damage law. The model is utilized in an optimization process to identify the corresponding material parameters and damage growth in single human trabeculae under tensile load. Results show that the damage model is leading to a better fit of the test data with an average root-mean -square-error (RMSE) of 2.52 MPa compared to the non-damage model with a RMSE of 3.03 MPa. Although this improvement is not significant, the damage model qualitatively better represents the data as it accounts for the visible stiffness reduction along the load history. It returns realistic stiffness values of 11.92 GPa for the instantaneous modulus and 5.73 GPa for the long term modulus of wet trabecular human bone. Further, the growth of damage in the tissue along the load history is substantial, with values above 0.8 close to failure. The relative loss of stiffness per cycle is in good agreement with comparable literature. Inverse rheological modeling proves to be a valuable tool for quantifying complex constitutive behavior from a single mechanical measurement. The evolution of damage in the tissue can be identified continuously over the load history and separated from other effects.
更多查看译文
关键词
2-layer rheological model,Damage,Trabecular bone,Single trabecula,Plasticity,Viscosity,Material parameter identification,Optimization
AI 理解论文
溯源树
样例
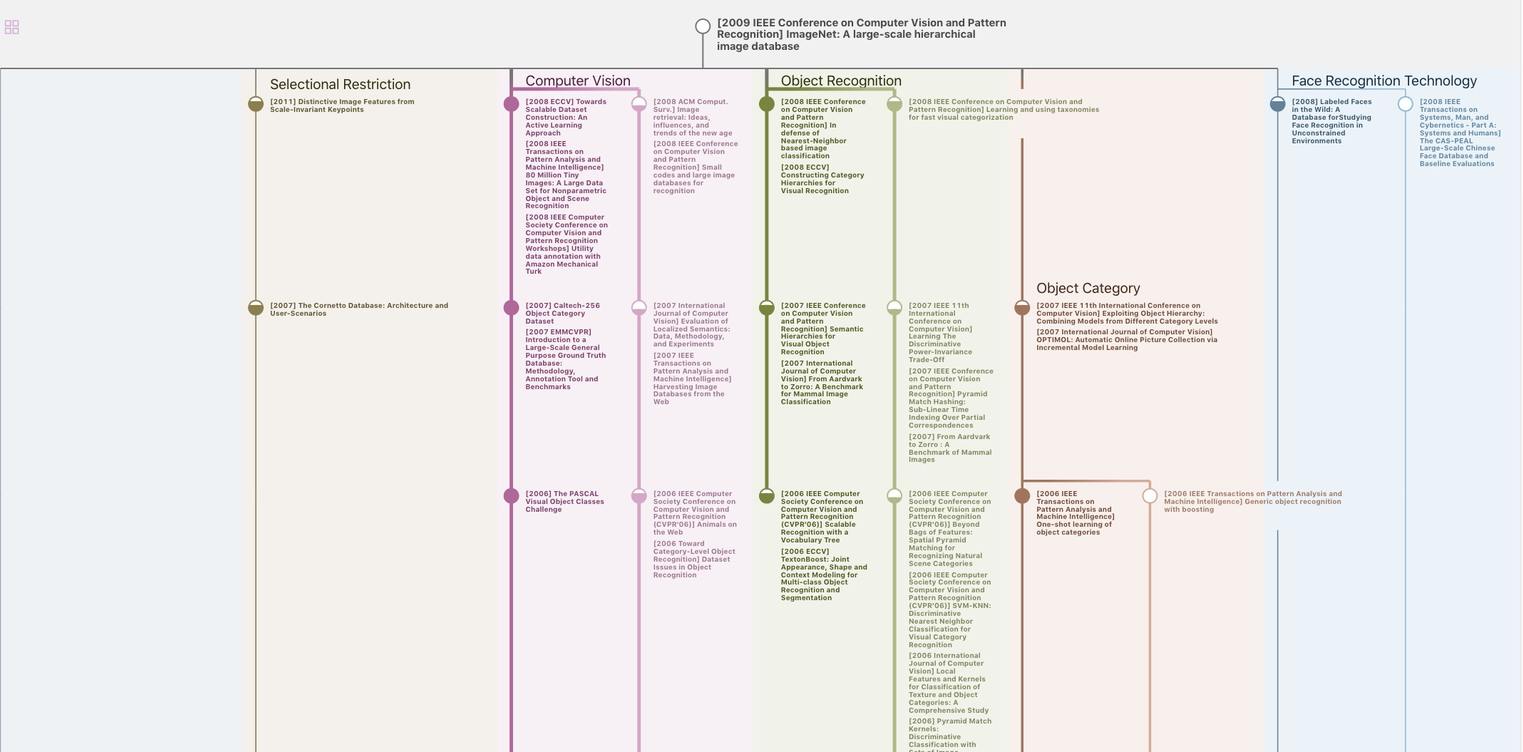
生成溯源树,研究论文发展脉络
Chat Paper
正在生成论文摘要