BockNet: Blind-Block Reconstruction Network With a Guard Window for Hyperspectral Anomaly Detection
IEEE TRANSACTIONS ON GEOSCIENCE AND REMOTE SENSING(2023)
摘要
Hyperspectral anomaly detection (HAD) aims to identify anomalous targets that deviate from the surrounding background in unlabeled hyperspectral images (HSIs). Most existing deep networks that exploit reconstruction errors to detect anomalies are prone to fit anomalous pixels, thus yielding small reconstruction errors for anomalies, which is not favorable for separating targets from HSIs. To achieve a superior background reconstruction network for HAD purposes, this article proposes a self-supervised blind-block network (termed BockNet) with a guard window. BockNet creates a blind-block (guard window) in the center of the network's receptive field, rendering it unable to see the information inside the guard window when reconstructing the central pixel. This process seamlessly embeds a sliding dual-window model into our BockNet, in which the inner window is the guard window and the outer window is the receptive field outside the guard window. Naturally, BockNet utilizes only the outer window information to predict/reconstruct the central pixel of the perceptive field. During the reconstruction of pixels inside anomalous targets of varying sizes, the targets typically fall into the guard window, weakening the contribution of anomalies to the reconstruction results so that those reconstructed pixels converge to the background distribution of the outer window area. Accordingly, the reconstructed HSI can be deemed as a pure background HSI, and the reconstruction error of anomalous pixels will be further enlarged, thus improving the discrimination ability of the BockNet model for anomalies. Extensive experiments on four datasets illustrate the competitive and satisfactory performance of our BockNet compared to other state-of-the-art detectors.
更多查看译文
关键词
Blind-spot network,convolutional neural network,deep learning (DL),hyperspectral image (HSI),image reconstruction,neural network,self-supervised learning
AI 理解论文
溯源树
样例
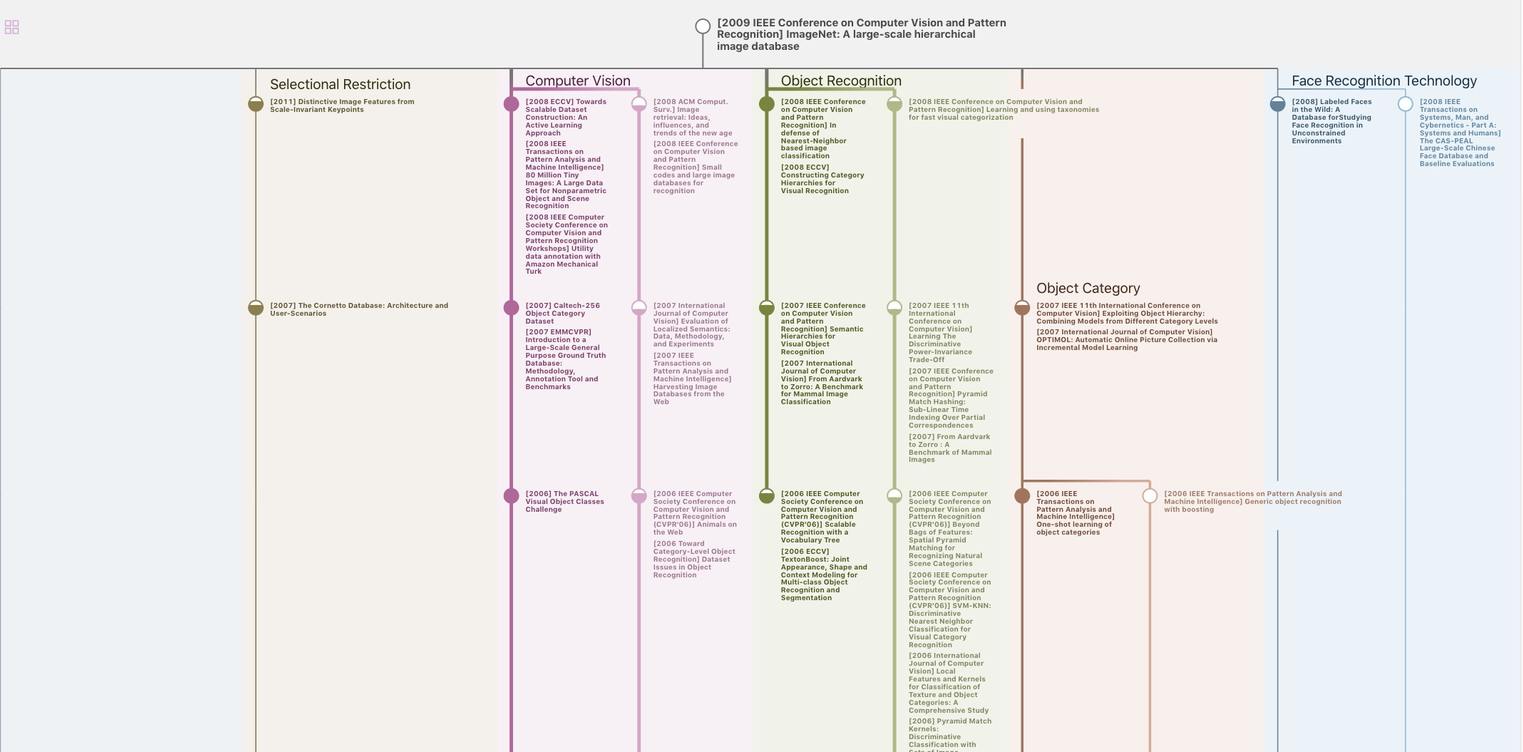
生成溯源树,研究论文发展脉络
Chat Paper
正在生成论文摘要