Artificial Neural Network Training on an Optical Processor via Direct Feedback Alignment
2023 Conference on Lasers and Electro-Optics Europe & European Quantum Electronics Conference (CLEO/Europe-EQEC)(2023)
摘要
Artificial Neural Networks (ANN) are habitually trained via the back-propagation (BP) algorithm. This approach has been extremely successful: Current models like GPT-3 have O(10
11
) parameters, are trained on O(10
11
) words and produce awe-inspiring results. However, there are good reasons to look for alternative training methods: With current algorithms and hardware constraints sometimes only half the available computing power is actually used. This is due to a complicated interplay between the size of the ANN, the available memory, throughput limitations of interconnects, the architecture of the network of computers, and the training algorithm. Training a model like the aforementioned GPT-3 takes months and costs millions. A different training paradigm, which could make clever use of specialized hardware, may train large ANNs more efficiently.
更多查看译文
关键词
ANN,artificial neural network training,back-propagation algorithm,BP,direct feedback alignment,GPT-3,hardware constraints,optical processor,training algorithm
AI 理解论文
溯源树
样例
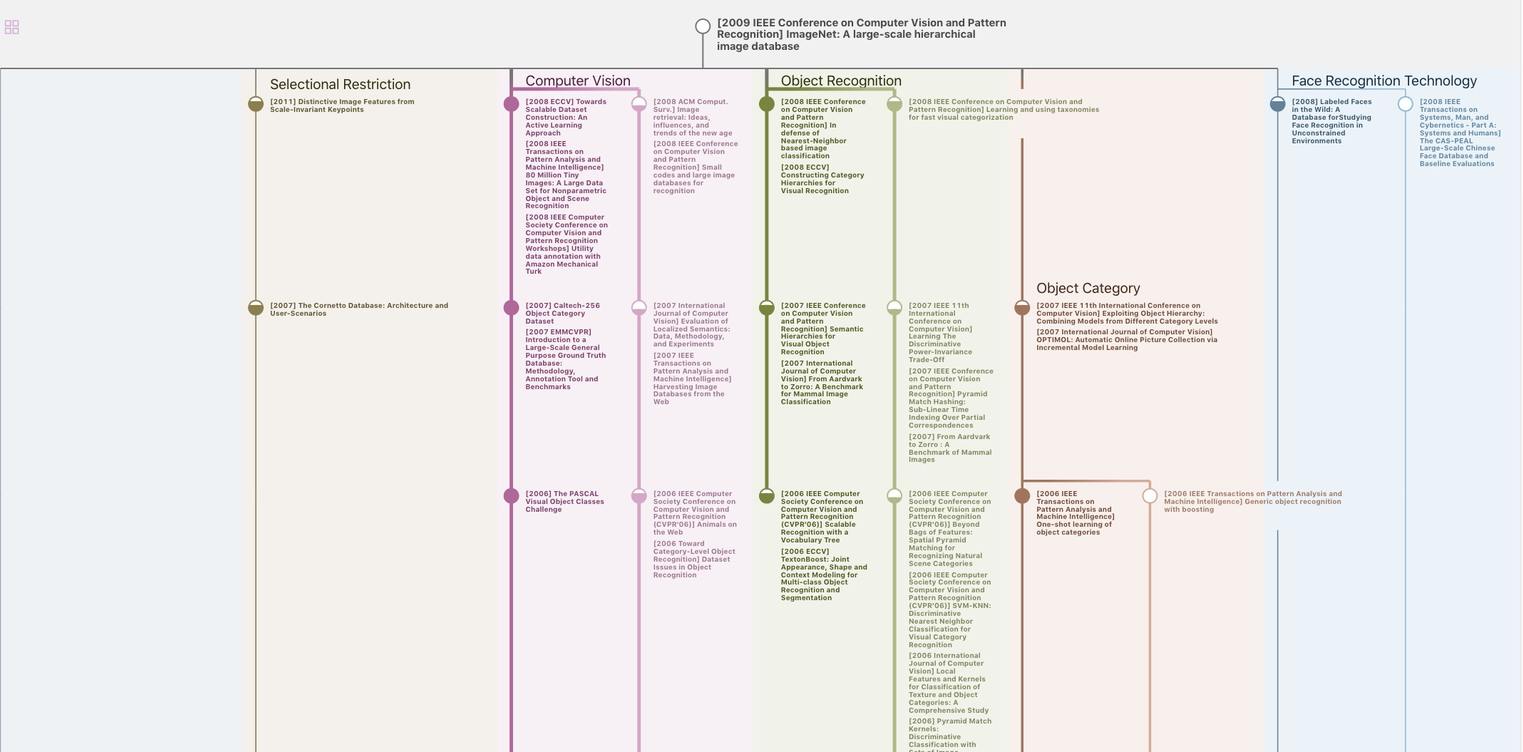
生成溯源树,研究论文发展脉络
Chat Paper
正在生成论文摘要