Distribution network fault work order prediction based on improved LSTNet
2023 5th International Conference on Electronic Engineering and Informatics (EEI)(2023)
摘要
The fault work order data of the distribution network can reflect the operation status of the network, and predicting the future number of work orders can provide data support for the maintenance of the network operation. Fault work order data is a time series with non-linear characteristics, which is influenced by meteorological factors and has a high feature dimension. In this paper, an improved LSTN t network is used to build a fault work order prediction model with multi-form outputs, and a residual connection strategy is introduced to address the gradient disappearance problem of the model in practical application scenarios. At the same time, an attention mechanism is introduced to improve the learning ability of the model for critical information. The experimental results prove that the RMSE and MAE of the proposed model are 12.266 and 8.087, and the AUC is 0.932, which is better than other machine learning and deep learning algorithms. The fault work order prediction algorithm in this paper can meet the application requirements in terms of prediction accuracy and speed.
更多查看译文
关键词
fault work order,meteorological factors,improved LSTNet,residual connectivity,attention mechanism
AI 理解论文
溯源树
样例
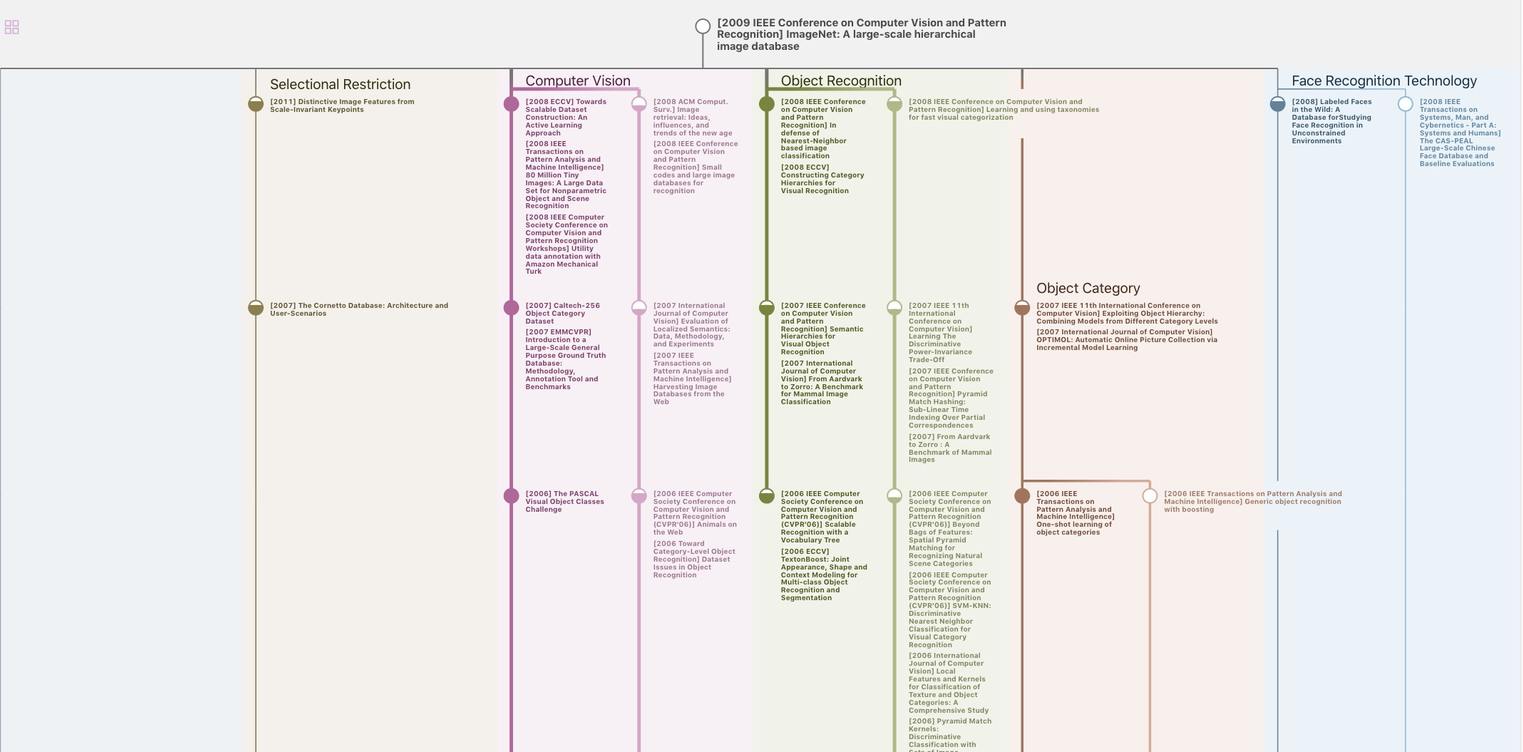
生成溯源树,研究论文发展脉络
Chat Paper
正在生成论文摘要