Fairness-Aware Dimensionality Reduction
2023 31st European Signal Processing Conference (EUSIPCO)(2023)
摘要
In this era of digitalization, the massive increase in available data leads to great potential for advancing various domains. However, the available data, such as images, videos, and speech signals, generally lie in high-dimensional space, which calls for efficient dimensionality reduction techniques to process them efficiently. Furthermore, while the fairness of algorithms is essential for their deployment in real-world systems, the effect of dimensionality reduction on fairness is an under-explored research area. Motivated by this, this paper puts forth a fairness-aware dimensionality reduction framework that is capable of properly compressing the data while mitigating bias. Specifically, our design targets at reducing the correlation between the compressed data and sensitive attributes, while projecting the data into a new coordinate system where most of its variation can be described. Experimental results on the CelebA dataset demonstrate that the proposed dimensionality reduction framework can improve group fairness measures for image classification while providing comparable utility to the conventional techniques.
更多查看译文
关键词
dimension reduction,fairness,PCA,image classification
AI 理解论文
溯源树
样例
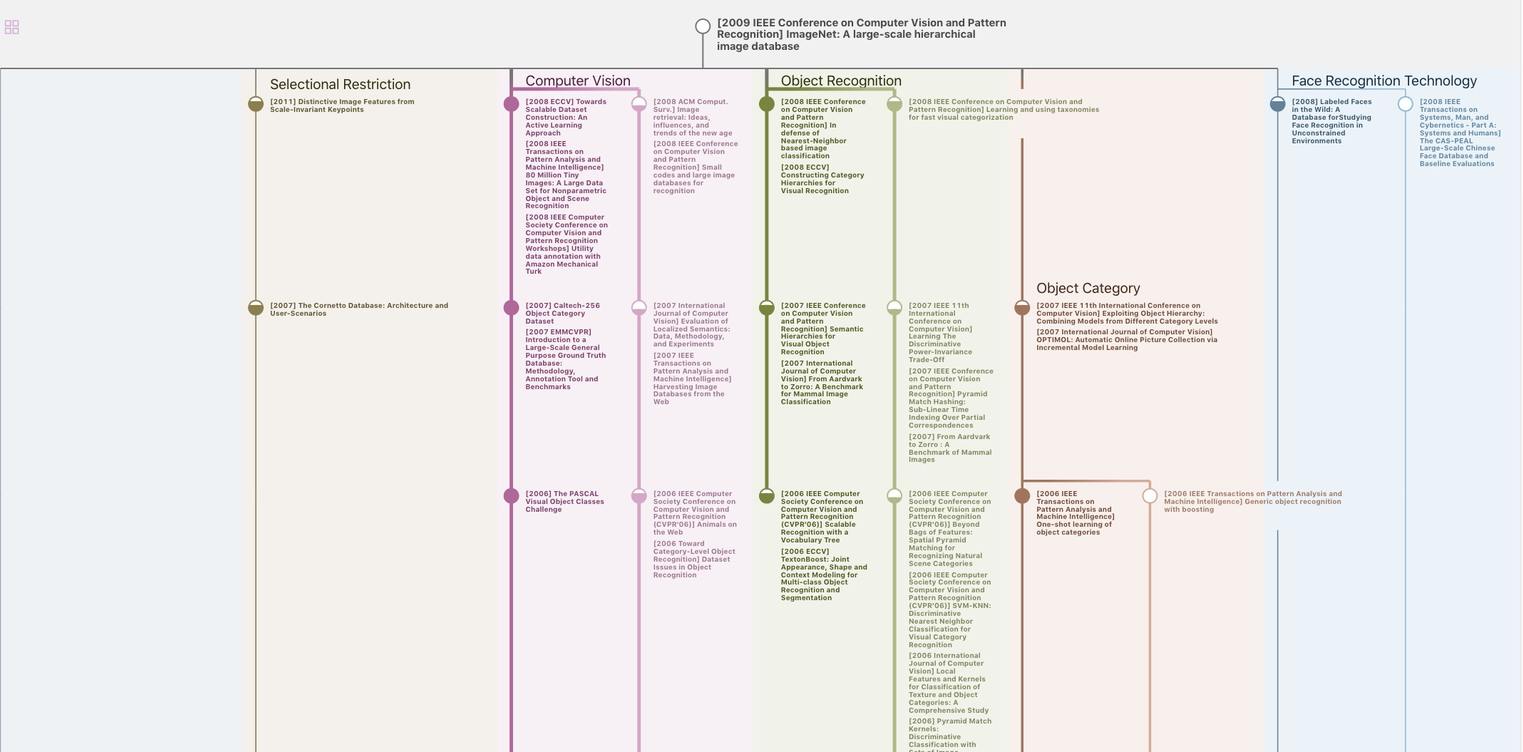
生成溯源树,研究论文发展脉络
Chat Paper
正在生成论文摘要