Parameter Transferability in QAOA Under Noisy Conditions
2023 IEEE International Conference on Quantum Computing and Engineering (QCE)(2023)
摘要
The quantum approximate optimization algorithm (QAOA) is widely recognized for its potential to deliver quantum advantage in combinatorial optimization tasks. As a hybrid classical-quantum algorithm, it achieves an approximate solution to the combinatorial optimization problem by preparing a specific quantum state via a classical optimization of the quantum circuit parameters. A key property of QAOA is the observed concentration and transferability of optimal parameters across distinct problem instances, linked to local graph characteristics such as subgraph types (lightcones) and node degrees (parity) [1]. However, quantum noise poses a critical challenge in the current NISQ era, hindering the performance of QAOA, particularly for large system sizes. In this follow-up study, we investigate the resilience of parameter transferability under noisy conditions. Leveraging zero-noise extrapolation (ZNE), we evaluate the transferability's robustness across various random graphs under different noise levels. Our analysis reveals that the transferability of optimal parameters from smaller ‘donor’ graphs to larger ‘acceptor’ graphs retains its effectiveness, even under substantial noise when combined with ZNE. Importantly, by illustrating that QAOA parameter transferability can still be exploited effectively among NISQ devices, we offer a pragmatic approach to enhance the algorithm's performance. Our research reinforces the promise of QAOA for large-scale applications under the practical challenges of quantum noise.
更多查看译文
关键词
quantum error mitigation,NISQ algorithms,hybrid quantum-classical architectures & computing
AI 理解论文
溯源树
样例
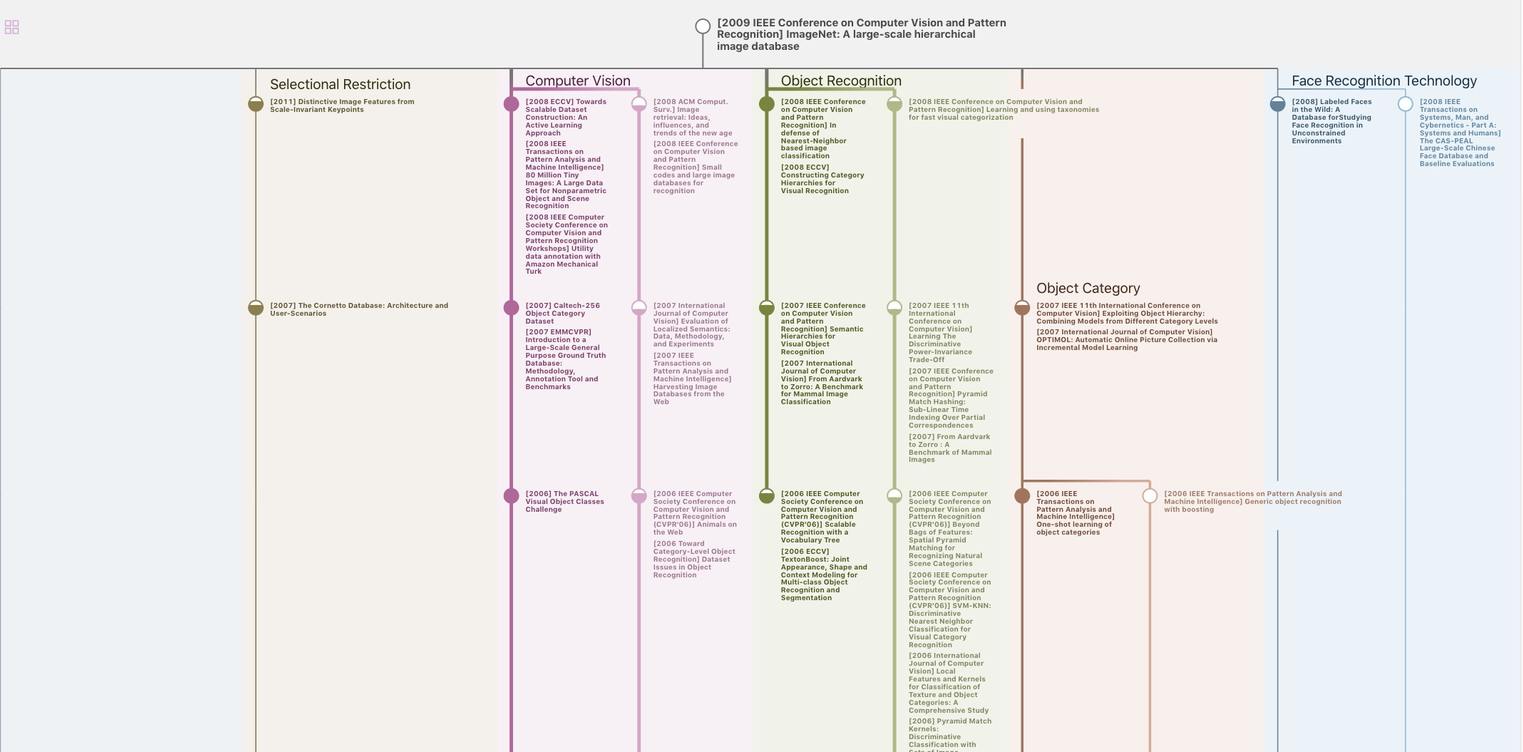
生成溯源树,研究论文发展脉络
Chat Paper
正在生成论文摘要