A Robust Multilabel Feature Selection Approach Based on Graph Structure Considering Fuzzy Dependency and Feature Interaction
IEEE TRANSACTIONS ON FUZZY SYSTEMS(2023)
摘要
The performance of multilabel learning depends heavily on the quality of the input features. A mass of irrelevant and redundant features may seriously affect the performance of multilabel learning, and feature selection is an effective technique to solve this problem. However, most multilabel feature selection methods mainly emphasize removing these useless features, and the exploration of feature interaction is ignored. Moreover, the widespread existence of real-world data with uncertainty, ambiguity, and noise limits the performance of feature selection. To this end, our work is dedicated to designing an efficient and robust multilabel feature selection scheme. First, the distribution character of multilabel data is analyzed to generate robust fuzzy multineighborhood granules. By exploring the classification information implied in the data under the granularity structure, a robust multilabel k-nearest neighbor fuzzy rough set model is constructed, and the concept of fuzzy dependency is studied. Second, a series of fuzzy multineighborhood uncertainty measures in k-nearest neighbor fuzzy rough approximation spaces are studied to analyze the correlations of feature pairs, including interactivity. Third, by investigating the uncertainty measure between feature and label, between features, multilabel data is modeled as a complete weighted graph. Then, these vertices are assessed iteratively to guide the assignment of feature weights. Finally, a graph structure-based robust multilabel feature selection algorithm (GRMFS) is designed. The experiments are conducted on 15 multilabel datasets. The results verify the superior performance of GRMFS as compared with nine representative feature selection methods.
更多查看译文
关键词
Feature interaction,fuzzy rough sets,graph structure,multilabel feature selection,robustness
AI 理解论文
溯源树
样例
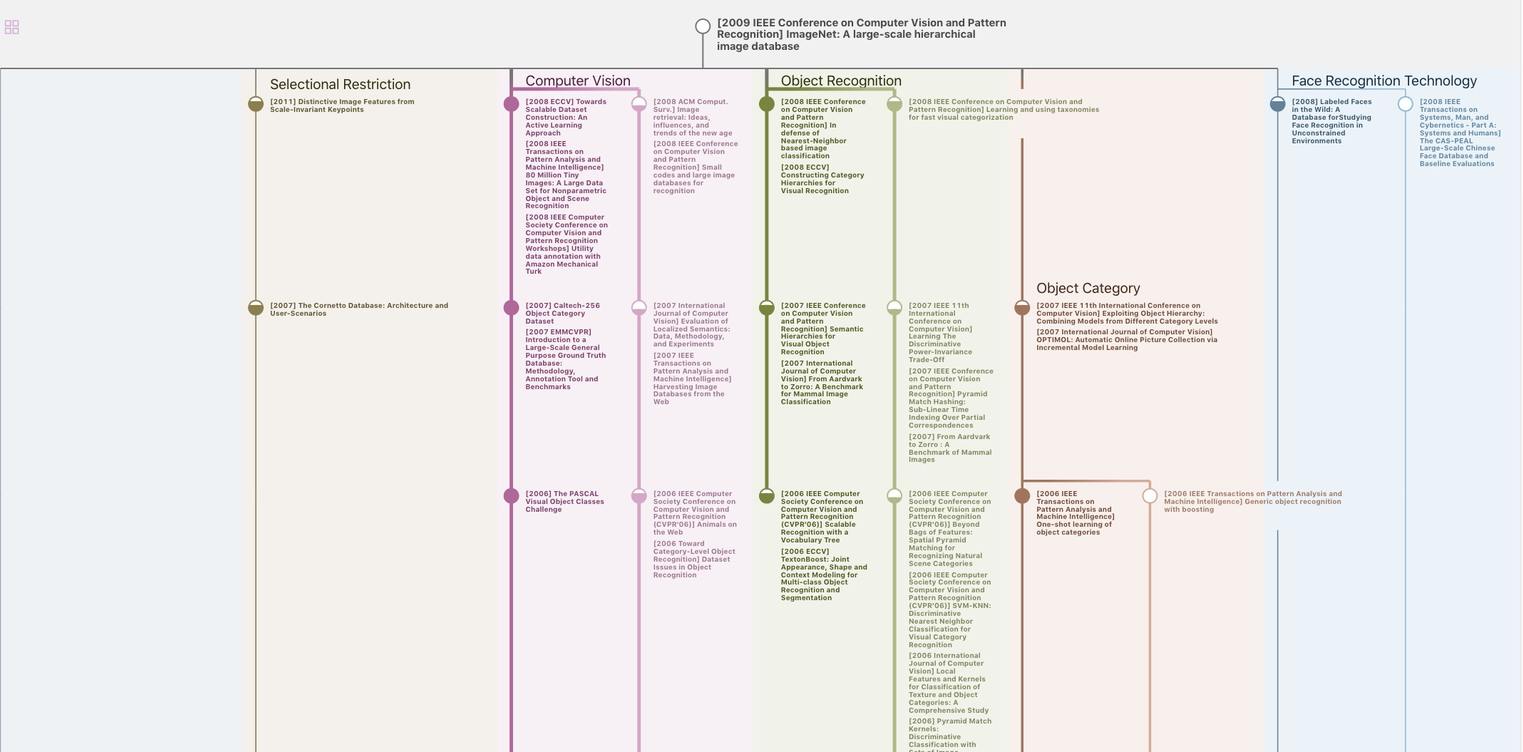
生成溯源树,研究论文发展脉络
Chat Paper
正在生成论文摘要