CLAD-Net: cross-layer aggregation attention network for real-time endoscopic instrument detection
Health Information Science and Systems(2023)
摘要
As medical treatments continue to advance rapidly, minimally invasive surgery (MIS) has found extensive applications across various clinical procedures. Accurate identification of medical instruments plays a vital role in comprehending surgical situations and facilitating endoscopic image-guided surgical procedures. However, the endoscopic instrument detection poses a great challenge owing to the narrow operating space, with various interfering factors (e.g. smoke, blood, body fluids) and inevitable issues (e.g. mirror reflection, visual obstruction, illumination variation) in the surgery. To promote surgical efficiency and safety in MIS, this paper proposes a cross-layer aggregated attention detection network (CLAD-Net) for accurate and real-time detection of endoscopic instruments in complex surgical scenarios. We propose a cross-layer aggregation attention module to enhance the fusion of features and raise the effectiveness of lateral propagation of feature information. We propose a composite attention mechanism (CAM) to extract contextual information at different scales and model the importance of each channel in the feature map, mitigate the information loss due to feature fusion, and effectively solve the problem of inconsistent target size and low contrast in complex contexts. Moreover, the proposed feature refinement module (RM) enhances the network’s ability to extract target edge and detail information by adaptively adjusting the feature weights to fuse different layers of features. The performance of CLAD-Net was evaluated using a public laparoscopic dataset Cholec80 and another set of neuroendoscopic dataset from Sun Yat-sen University Cancer Center. From both datasets and comparisons, CLAD-Net achieves the AP_0.5 of 98.9 https://github.com/A0268/video-demo .
更多查看译文
关键词
Cross-layer feature aggregation,Composite attention mechanism,Refinement module,Surgical instrument detection
AI 理解论文
溯源树
样例
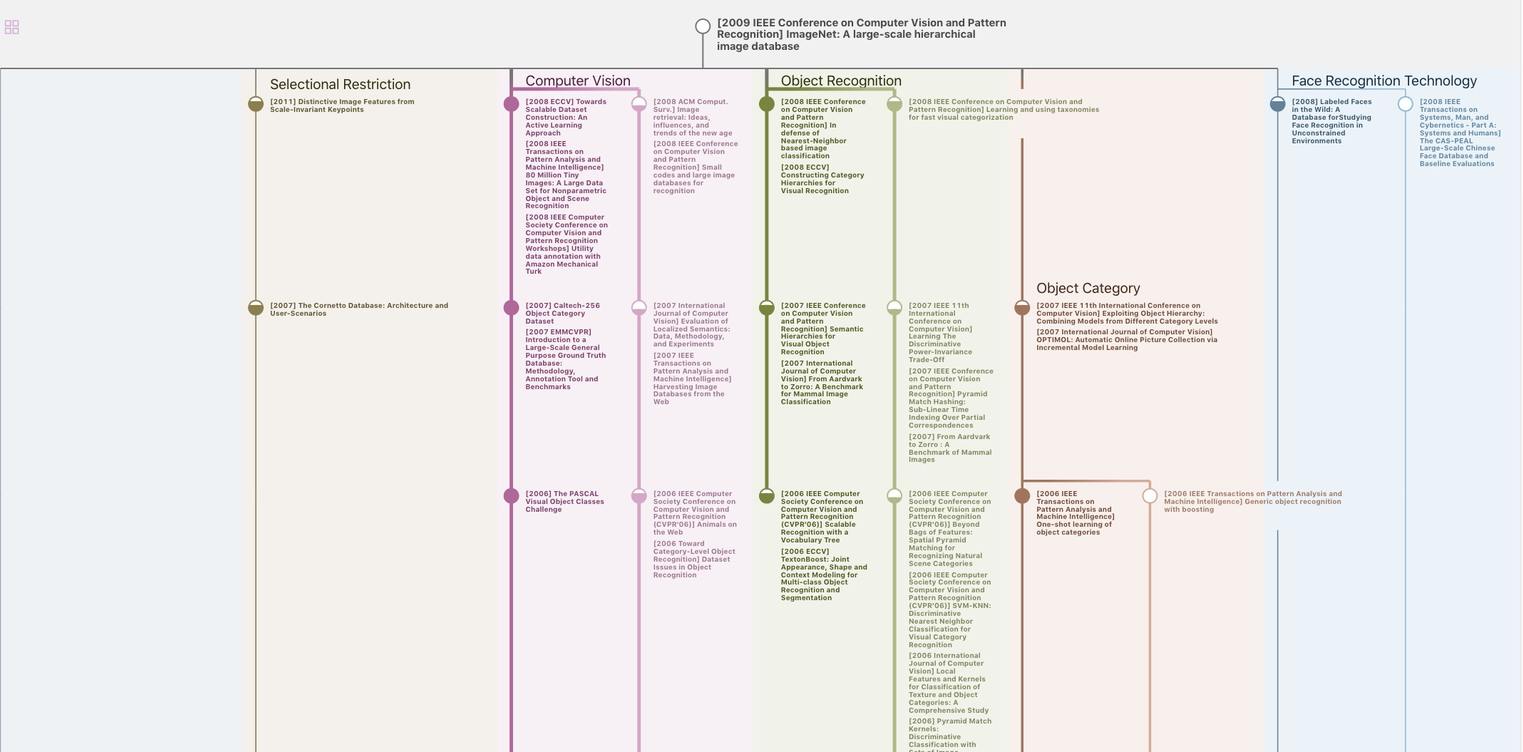
生成溯源树,研究论文发展脉络
Chat Paper
正在生成论文摘要