Drug repositioning based on weighted local information augmented graph neural network
BRIEFINGS IN BIOINFORMATICS(2024)
摘要
Drug repositioning, the strategy of redirecting existing drugs to new therapeutic purposes, is pivotal in accelerating drug discovery. While many studies have engaged in modeling complex drug-disease associations, they often overlook the relevance between different node embeddings. Consequently, we propose a novel weighted local information augmented graph neural network model, termed DRAGNN, for drug repositioning. Specifically, DRAGNN firstly incorporates a graph attention mechanism to dynamically allocate attention coefficients to drug and disease heterogeneous nodes, enhancing the effectiveness of target node information collection. To prevent excessive embedding of information in a limited vector space, we omit self-node information aggregation, thereby emphasizing valuable heterogeneous and homogeneous information. Additionally, average pooling in neighbor information aggregation is introduced to enhance local information while maintaining simplicity. A multi-layer perceptron is then employed to generate the final association predictions. The model's effectiveness for drug repositioning is supported by a 10-times 10-fold cross-validation on three benchmark datasets. Further validation is provided through analysis of the predicted associations using multiple authoritative data sources, molecular docking experiments and drug-disease network analysis, laying a solid foundation for future drug discovery.
更多查看译文
关键词
drug-disease association,drug repositioning,graph neural network,graph attention mechanism,local information augmentation
AI 理解论文
溯源树
样例
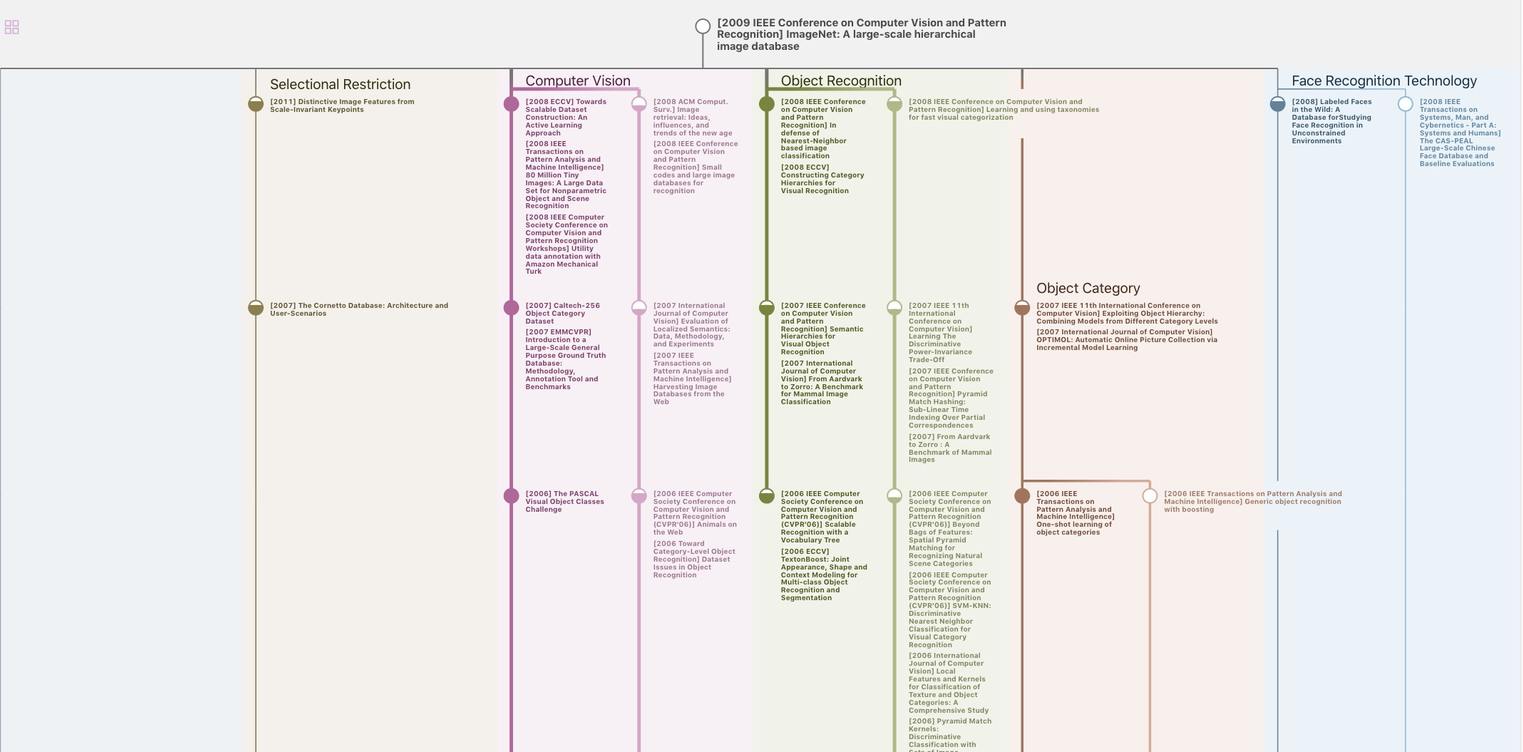
生成溯源树,研究论文发展脉络
Chat Paper
正在生成论文摘要