Ultrasonic Imaging and Flaw Detection with Optimized Convolutional Transformer Neural Networks
2023 IEEE International Ultrasonics Symposium (IUS)(2023)
摘要
Flaw detection using the ultrasonic imaging technique has been widely used for Structural Health Monitoring (SHM). Ultrasonic testing has the advantages of one-sided measurement, high penetration depth, and inspection accuracy. Artificial intelligence (AI) such as Deep Learning (DL) methods can automate the inspection process with high reliability for imaging and SHM. Neural Networks (NNs) and DL methods can detect flaws using ultrasonic images with high accuracy but suffer extensive computational costs for training and deployment. In this study, we propose a lightweight transformer NN, UFDCTNet: Ultrasonic Flaw Detection Convolutional Transformer Neural Network (TNN), optimized with data-efficient Convolutional NN (CNN) for flaw detection using ultrasonic imaging. TNN utilizes the self-attention network architecture that learns global representations and allows high parallelism in computation resulting in reduced training time. CNNs learn local representations with fewer parameters because of inherent spatial inductive bias. This UFDCTNet utilizes the advantages of CNNs to learn spatially local representations resulting in fewer model parameters for fast training. For performance analysis, we trained data-efficient TNN and CNN using ultrasonic images to detect flaws. To examine training results, NNs are trained with the USimgAIST dataset consisting of 7000 experimental B-scan images representing without-flaw and with-flaw cases. A pulsed laser ultrasonic scanning system was used to collect these B-scan images from 17 stainless steel specimen plates with various types of flaws and some plates without any damage.
更多查看译文
关键词
Flaw Detection,Convolutional Optimization,Lightweight Transformer Neural Networks,Data-Efficient Neural Networks,Ultrasonic Imaging,In-situation SHM
AI 理解论文
溯源树
样例
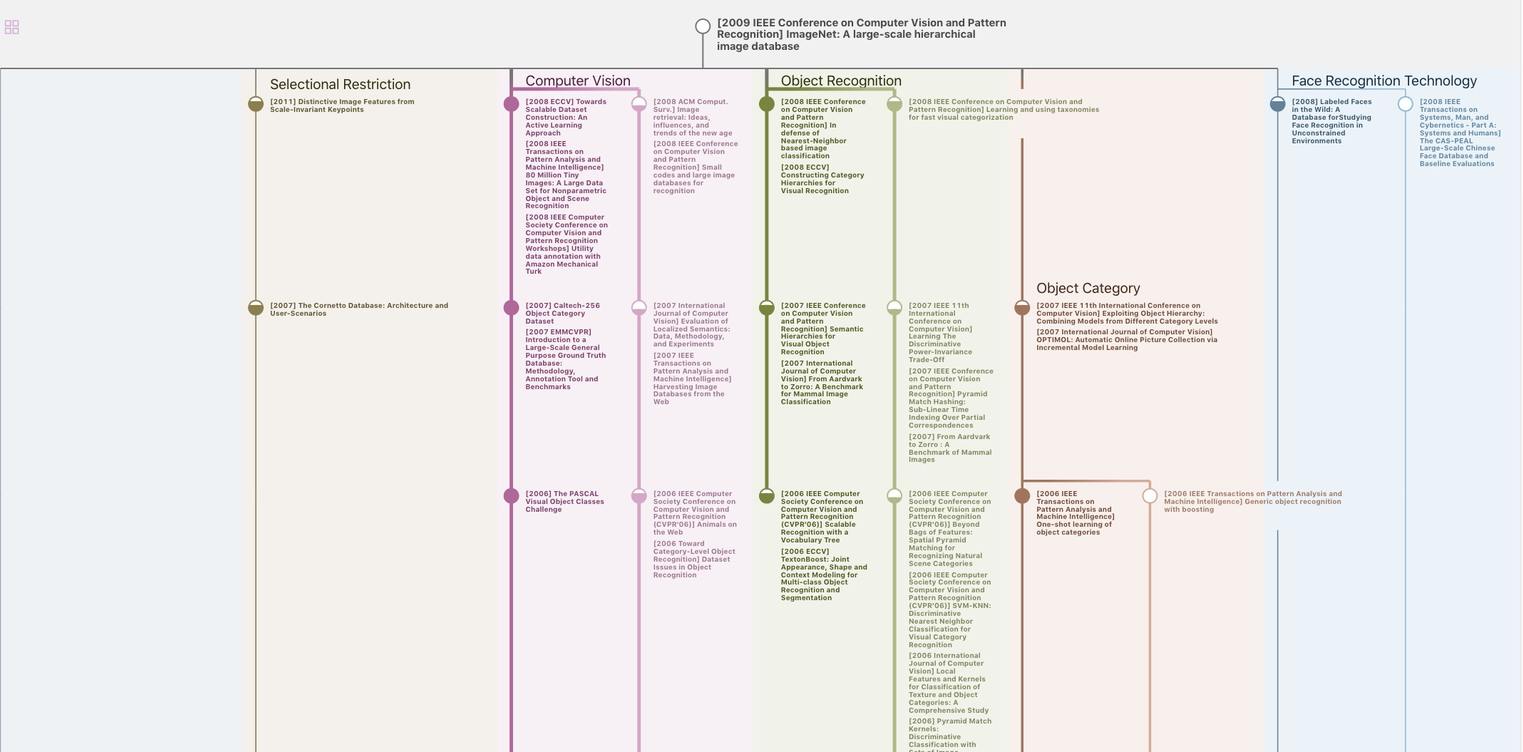
生成溯源树,研究论文发展脉络
Chat Paper
正在生成论文摘要