Data Compression for Ultrasonic Microstructure Scattering Signals using Unsupervised Neural Networks
2023 IEEE International Ultrasonics Symposium (IUS)(2023)
摘要
Ultrasonic Nondestructive Evaluation (NDE) has been extensively used to characterize the microstructure of metallic structures for early exposure of materials integrity. However, industrial NDE requires the processing, storage, and real-time transmission of large volumes of ultrasonic data. Therefore, it is indispensable to compress ultrasonic data with high fidelity. In this study, we explore the development of Unsupervised Learning (UL) based Neural Network (NN) models for massive ultrasonic data compression and an innovative multilayer perceptron residual autoencoder: Ultrasonic Residual Compressive Autoencoder (URCA), is introduced to compress ultrasonic data with high compression performance. This URCA can be fast-trained and utilizes the sparsity penalty with residual connection to optimize compression performance. UL-based NNs allow for memory-efficient training and rapid online augmentation of the model. To examine the results, a high-performance ultrasonic signal acquisition system was assembled to automatically collect ultrasonic data from heat-treated 1,018 steel blocks for microstructure characterization. Compression performance is analyzed based on compression ratio, reconstruction accuracy and model training time. The reconstruction accuracy was measured using the Structural Similarity Index Measure (SSIM) and Peak Signal-to-Noise Ratio (PSNR). By training the URCA NN for a high reconstruction performance of 0.96 SSIM, we obtained 91.25% memory space-saving. For a higher compression performance of 0.80 SSIM, we obtained 96.04% memory space-saving.
更多查看译文
关键词
Unsupervised Neural Networks,Ultrasonic NDE Data Compression,Compressive Autoencoder,Sparse Dictionary Learning,Incremental Learning,Microstructure Characterization
AI 理解论文
溯源树
样例
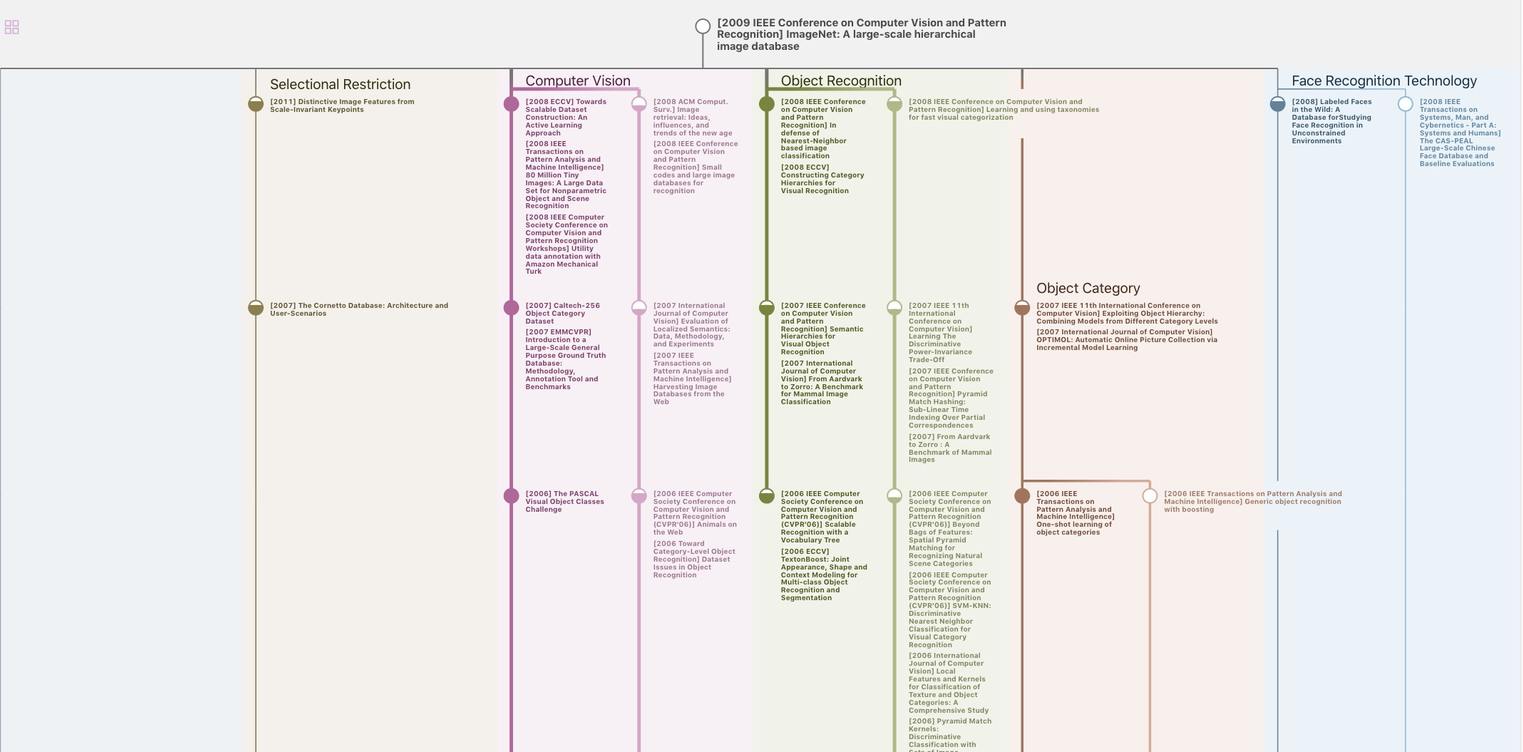
生成溯源树,研究论文发展脉络
Chat Paper
正在生成论文摘要