Robot causal discovery aided by human interaction
2023 32ND IEEE INTERNATIONAL CONFERENCE ON ROBOT AND HUMAN INTERACTIVE COMMUNICATION, RO-MAN(2023)
摘要
Causality is relatively unexplored in robotics even if it is highly relevant, in several respects. In this paper, we study how a robot's causal understanding can be improved by allowing the robot to ask humans causal questions. We propose a general algorithm for selecting direct causal effects to ask about, given a partial causal representation (using partially directed acyclic graphs, PDAGs) obtained from observational data. We propose three versions of the algorithm inspired by different causal discovery techniques, such as constraint-based, score-based, and interventions. We evaluate the versions in a simulation study and our results show that asking causal questions improves the causal representation over all simulated scenarios. Further, the results show that asking causal questions based on PDAGs discovered from data provides a significant improvement compared to asking questions at random, and the version inspired by score-based techniques performs particularly well over all simulated experiments.
更多查看译文
AI 理解论文
溯源树
样例
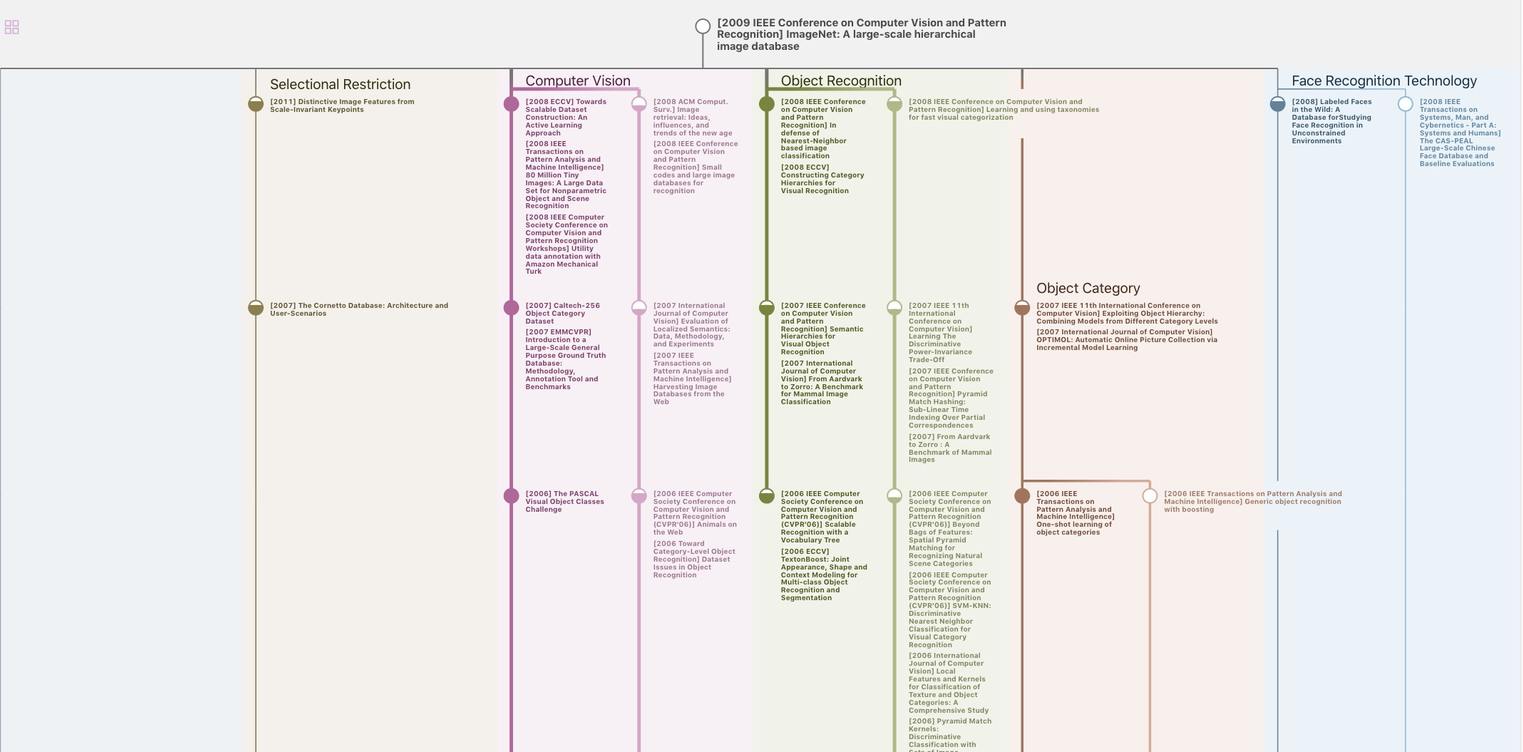
生成溯源树,研究论文发展脉络
Chat Paper
正在生成论文摘要