Exploiting a No-Regret Opponent in Repeated Zero-Sum Games
Journal of Shanghai Jiaotong University (Science)(2023)
摘要
In repeated zero-sum games, instead of constantly playing an equilibrium strategy of the stage game, learning to exploit the opponent given historical interactions could typically obtain a higher utility. However, when playing against a fully adaptive opponent, one would have difficulty identifying the opponent’s adaptive dynamics and further exploiting its potential weakness. In this paper, we study the problem of optimizing against the adaptive opponent who uses no-regret learning. No-regret learning is a classic and widely-used branch of adaptive learning algorithms. We propose a general framework for online modeling no-regret opponents and exploiting their weakness. With this framework, one could approximate the opponent’s no-regret learning dynamics and then develop a response plan to obtain a significant profit based on the inferences of the opponent’s strategies. We employ two system identification architectures, including the recurrent neural network (RNN) and the nonlinear autoregressive exogenous model, and adopt an efficient greedy response plan within the framework. Theoretically, we prove the approximation capability of our RNN architecture at approximating specific no-regret dynamics. Empirically, we demonstrate that during interactions at a low level of non-stationarity, our architectures could approximate the dynamics with a low error, and the derived policies could exploit the no-regret opponent to obtain a decent utility.
更多查看译文
关键词
no-regret learning,repeated game,opponent exploitation,opponent modeling,dynamical system,system identification,recurrent neural network (RNN)
AI 理解论文
溯源树
样例
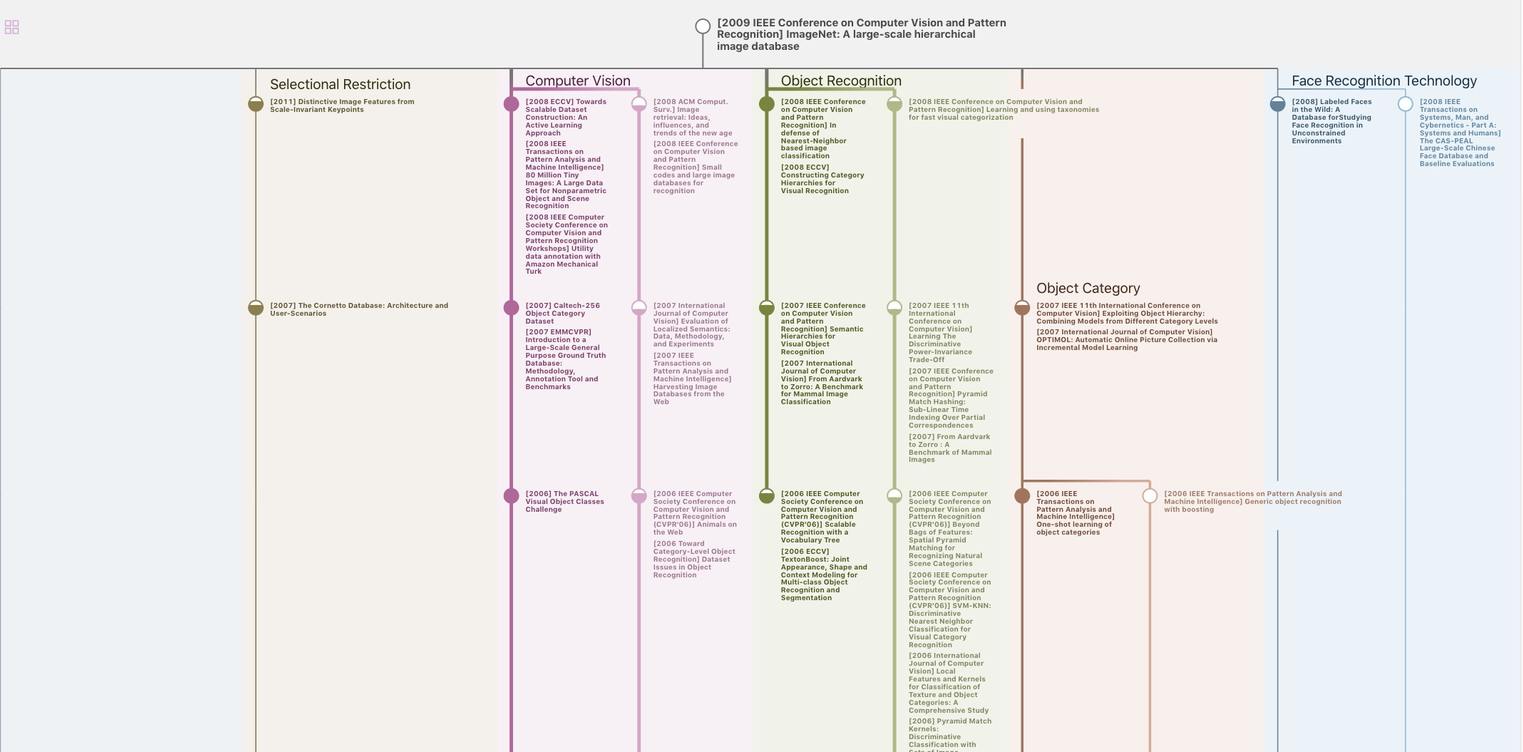
生成溯源树,研究论文发展脉络
Chat Paper
正在生成论文摘要