FL-AMM: Federated Learning Augmented Map Matching With Heterogeneous Cellular Moving Trajectories
IEEE JOURNAL ON SELECTED AREAS IN COMMUNICATIONS(2023)
摘要
Map matching is a fundamental component for location-based services (LBSs), such as vehicle mobility analysis, navigation services, traffic scheduling, etc. In this paper, we investigate federated learning augmented map matching based on heterogeneous cellular moving trajectories from different operator systems, the goal of which is to improve matching accuracy without violating the user privacy. First, we develop a data collection platform with one Android-based application, and conduct rigorous data collection campaigns. Second, we perform systematic data analytics to reveal the data-driven technical challenges, including the impact of sampling rate, high location error of cellular moving data, and poor heterogeneous matching performance. Third, we propose an augmented map matching model, named FL-AMM, i.e., Federated Learning Augmented Map Matching, in which we i) adopt the vertical federated learning framework to achieve data collaboration and privacy protection for heterogeneous operators; ii) devise a data augmentation component to enhance the capability of representing the raw cellular data; and iii) design a map matching model to further learn the mapping function from cellular trajectory points to road segments. Finally, we conduct extensive data-driven experiments to corroborate the efficiency and robustness of the proposed FL-AMM.
更多查看译文
关键词
Trajectory,Hidden Markov models,Data models,Federated learning,Global Positioning System,Roads,Data privacy,Vertical federated learning,map matching augmentation,heterogeneous trajectory fusion,stacked bidirectional gated recurrent unit,attention mechanism
AI 理解论文
溯源树
样例
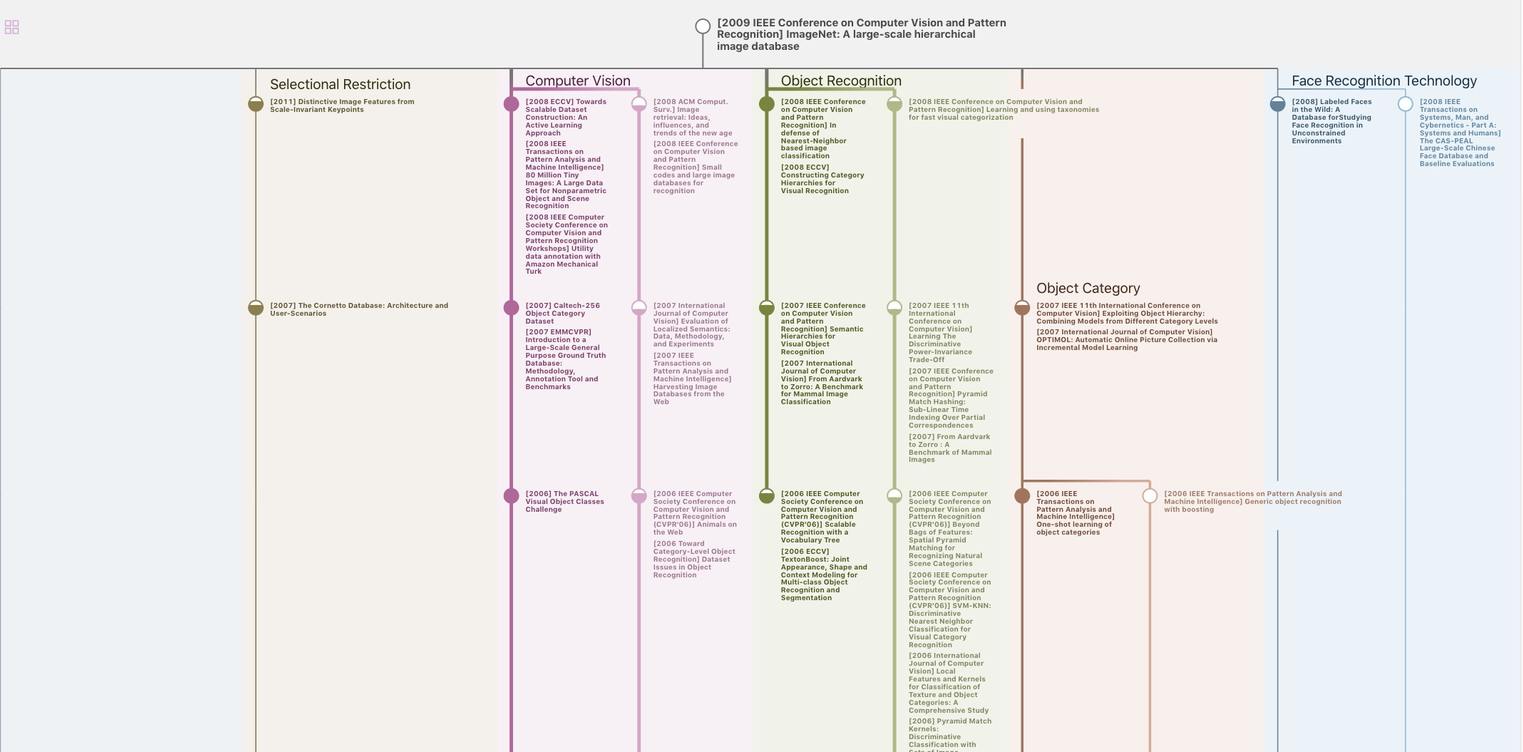
生成溯源树,研究论文发展脉络
Chat Paper
正在生成论文摘要