Application of the PSO algorithm based on federated learning strategy to optimize LSTM model in power load forecasting
2023 IEEE 18th Conference on Industrial Electronics and Applications (ICIEA)(2023)
摘要
As an important part of the development of smart grid, power load forecasting plays an important role in the important links of power system operation such as energy management and power system infrastructure planning. In order to improve the accuracy of the machine learning model as much as possible, it is necessary to use a large amount of data to train the model. However, sharing household power consumption data seriously threatens the privacy of users, and data migration will occupy a large amount of communication resources. In this paper, we propose the application of federated learning strategies to electric load forecasting in smart grids, and evaluate the performance of the federated learning strategy model on load forecasting. Compare it with centralized learning models and individual models, and discuss the advantages and disadvantages of the three strategies. The results show that the federated learning strategy has superior predictive performance.
更多查看译文
关键词
electric load forecasting,federated learning,particle swarm optimization (PSO),long short-term memory network (LSTM)
AI 理解论文
溯源树
样例
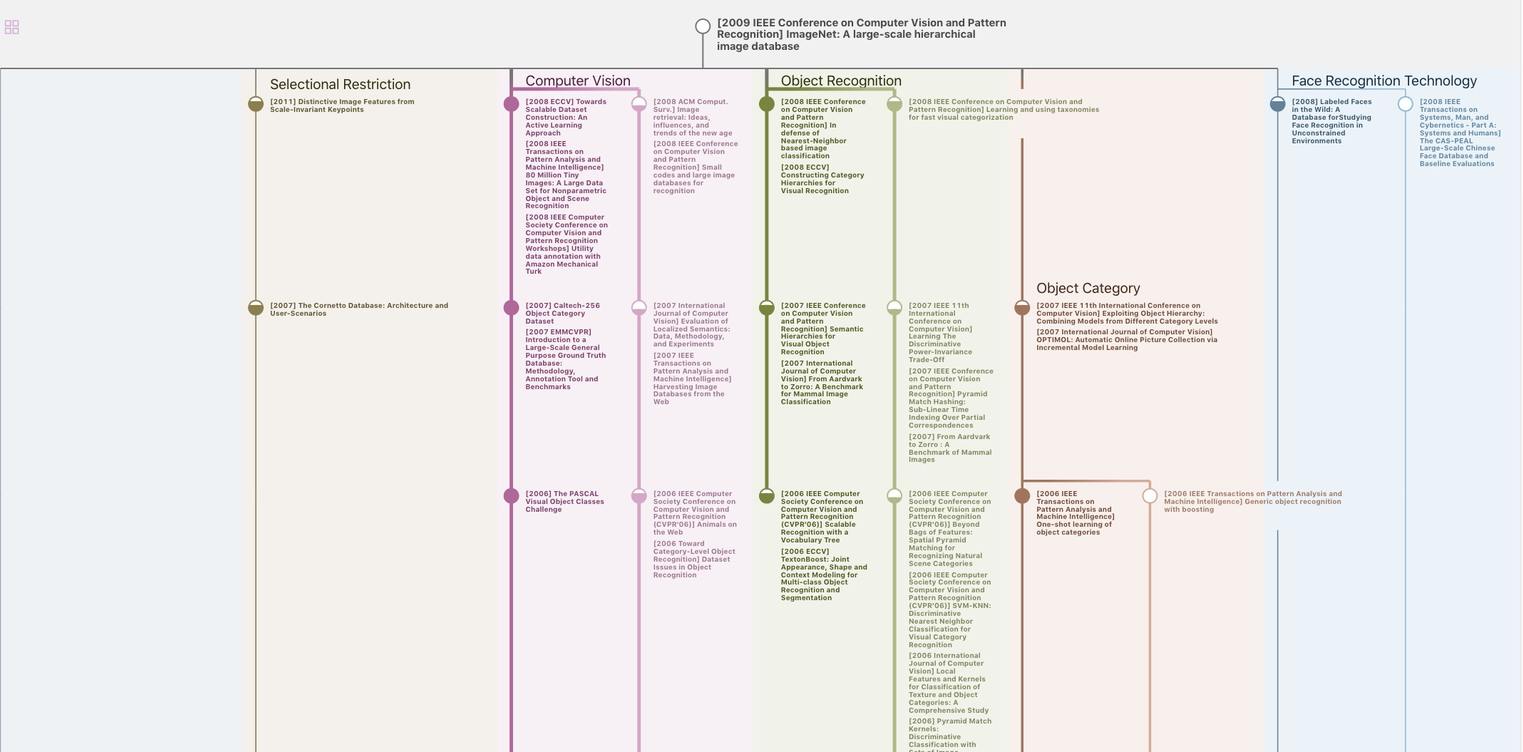
生成溯源树,研究论文发展脉络
Chat Paper
正在生成论文摘要