DistSNE: Distributed computing and online visualization of DNA methylation-based central nervous system tumor classification
BRAIN PATHOLOGY(2024)
摘要
The current state-of-the-art analysis of central nervous system (CNS) tumors through DNA methylation profiling relies on the tumor classifier developed by Capper and colleagues, which centrally harnesses DNA methylation data provided by users. Here, we present a distributed-computing-based approach for CNS tumor classification that achieves a comparable performance to centralized systems while safeguarding privacy. We utilize the t-distributed neighborhood embedding (t-SNE) model for dimensionality reduction and visualization of tumor classification results in two-dimensional graphs in a distributed approach across multiple sites (DistSNE). DistSNE provides an intuitive web interface () for user-friendly local data management and federated methylome-based tumor classification calculations for multiple collaborators in a DataSHIELD environment. The freely accessible web interface supports convenient data upload, result review, and summary report generation. Importantly, increasing sample size as achieved through distributed access to additional datasets allows DistSNE to improve cluster analysis and enhance predictive power. Collectively, DistSNE enables a simple and fast classification of CNS tumors using large-scale methylation data from distributed sources, while maintaining the privacy and allowing easy and flexible network expansion to other institutes. This approach holds great potential for advancing human brain tumor classification and fostering collaborative precision medicine in neuro-oncology. Overview of CNS tumor methylome data and the computational setup (a). Data contribution of the collected data. The most frequent subgroups are color-coded. (b) R Shiny web application for distributed t-SNE plotting. Upon user login, raw data can be uploaded to the analysis server via the R Shiny interface. A zoom-in of the area of interest provides a detailed view of the sample (marked with an asterisk) and its surrounding area. (c) Structure of the distributed t-SNE across the three sites. A central R client portal manages the computation of the distributed datasets in Frankfurt, Marburg, and Giessen.image
更多查看译文
关键词
brain tumor classification,distributed computing,DataSHIELD,methylome,tSNE,webinterface
AI 理解论文
溯源树
样例
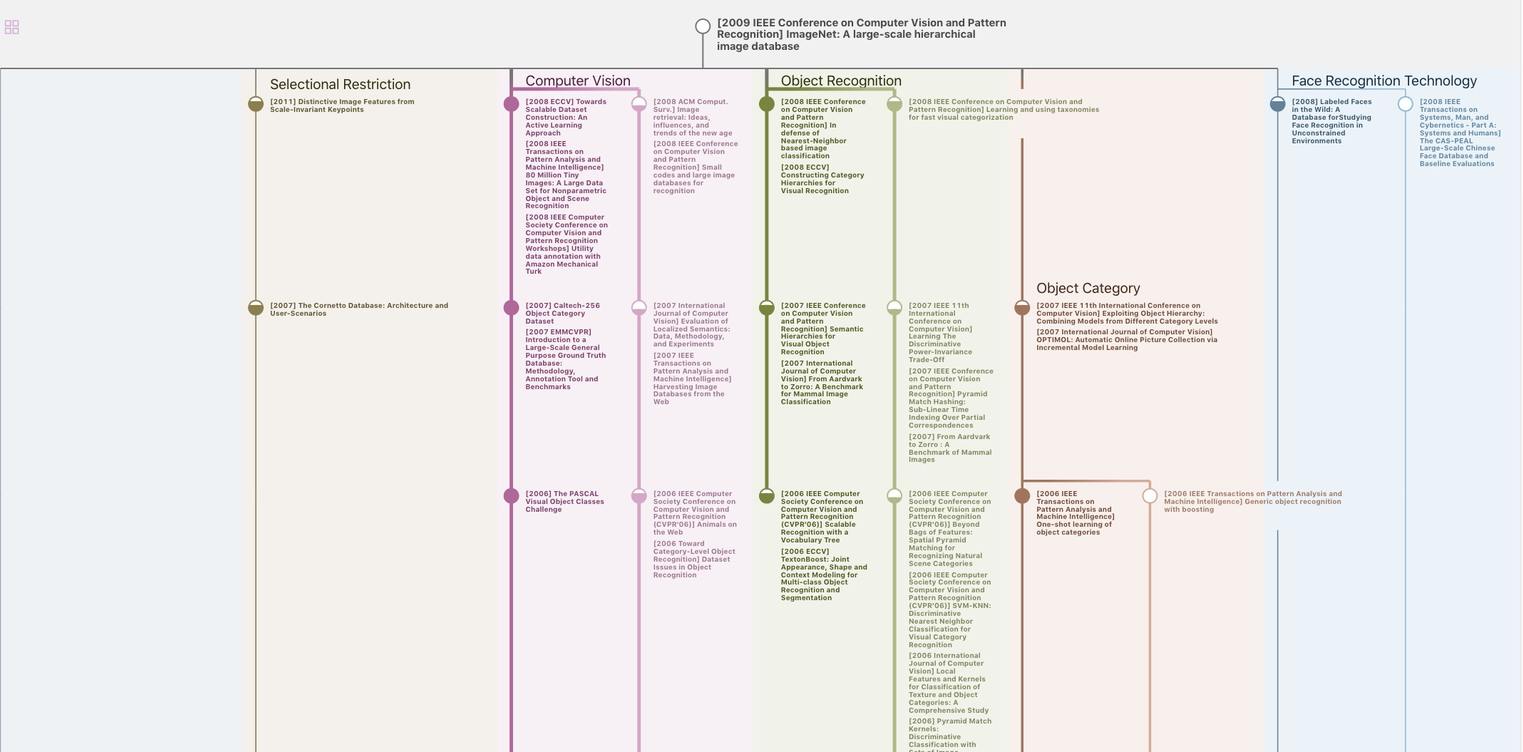
生成溯源树,研究论文发展脉络
Chat Paper
正在生成论文摘要