A penalized modified huber regularization to improve adversarial robustness
2023 IEEE INTERNATIONAL CONFERENCE ON IMAGE PROCESSING, ICIP(2023)
摘要
Adversarial training (AT) is a learning procedure that trains a deep neural network with adversary examples to improve robustness. AT and its variants are widely considered the most empirically successful against adversary examples. Along the same line, this work proposes a new training objective, PMHR-AT (Penalized Modified Huber Regularization for Adversarial training) for improving adversarial robustness. PMHR-AT minimizes both natural and adversarial risk and introduces a modified Huber loss between the natural and adversarial logits as a regularization with the regularization strength adjusted based on the similarity between the predicted natural and adversarial class probabilities. Experimental results show that the proposed method recorded a better performance than existing methods on strong attacks and offers a better trade-off between the natural accuracy and adversarial robustness.
更多查看译文
关键词
Adversarial Robustness,Adversarial Training,modified Huber loss
AI 理解论文
溯源树
样例
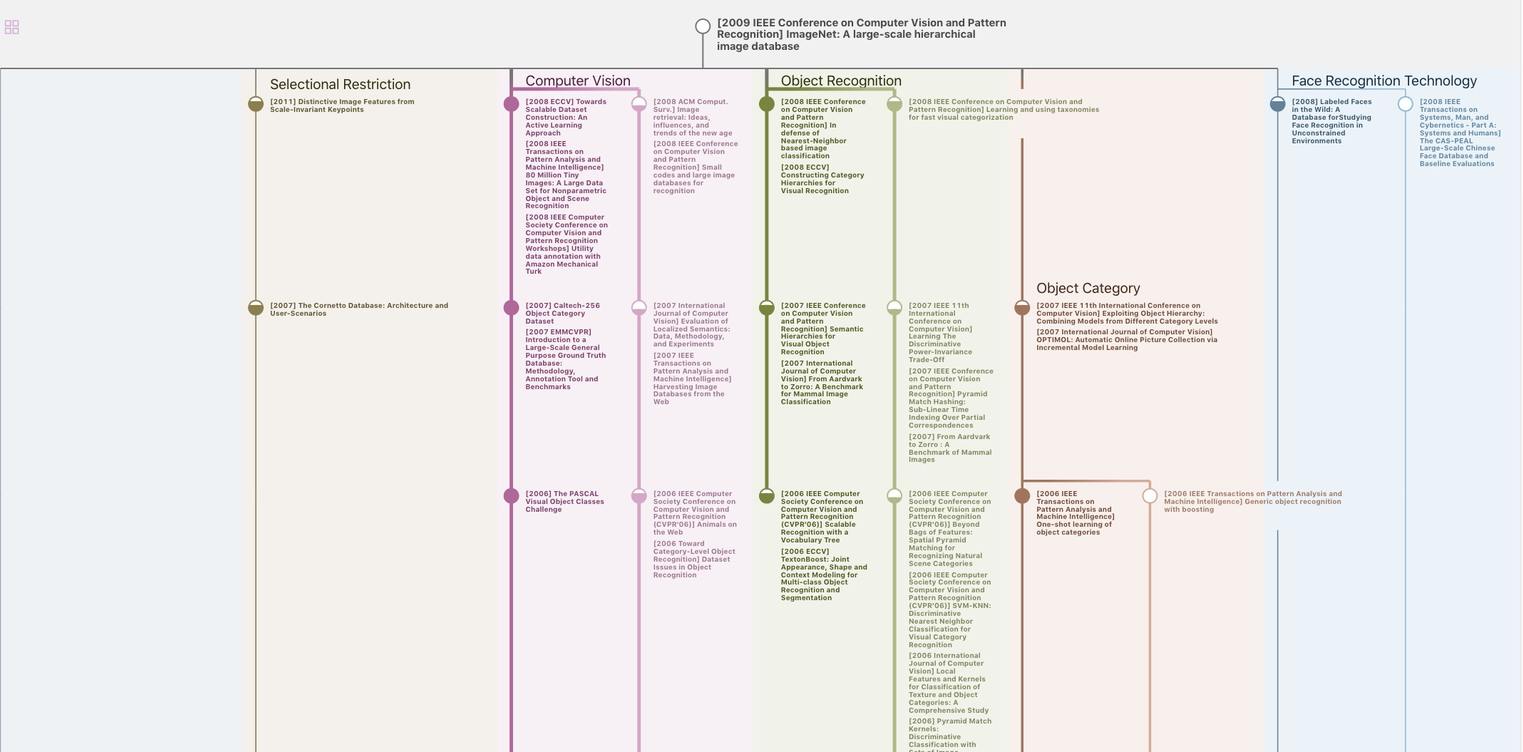
生成溯源树,研究论文发展脉络
Chat Paper
正在生成论文摘要