Hybrid contrastive prototypical network for few-shot scene classification
2023 IEEE INTERNATIONAL CONFERENCE ON IMAGE PROCESSING, ICIP(2023)
摘要
Few-shot learning has received widespread attention in remote sensing image scene classification. Many existing methods address this challenge by utilizing meta-learning and metric learning, which focus on developing feature extractors that can quickly adapt to novel few-shot scene classification (FSSC) tasks. However, these methods are often insufficient for real-world datasets with class confusion, where there is high inter-class compactness and intra-class diversity. To overcome this issue, we investigate efficient strategies, i.e., meta-learning-based transferable feature representation and contrastive-based prototypical regularization for learning task-adaptive class boundaries for FSSC. Specifically, we designed a combination of Query-vs-Prototype contrastive loss and Prototype-vs-Prototype contrastive loss to normalize the prototypical representation to be more discriminative in a novel FSSC task. Our proposed model is named the Hybrid Contrastive Prototypical Network (HCP-Net). Experiment results on three popular datasets under two standard benchmarks, i.e., general few-shot classification and few-shot domain generalization, indicate the effectiveness of the proposed method.
更多查看译文
关键词
Remote Sensing,Few-shot scene classification,Meta-learning,Contrastive Learning
AI 理解论文
溯源树
样例
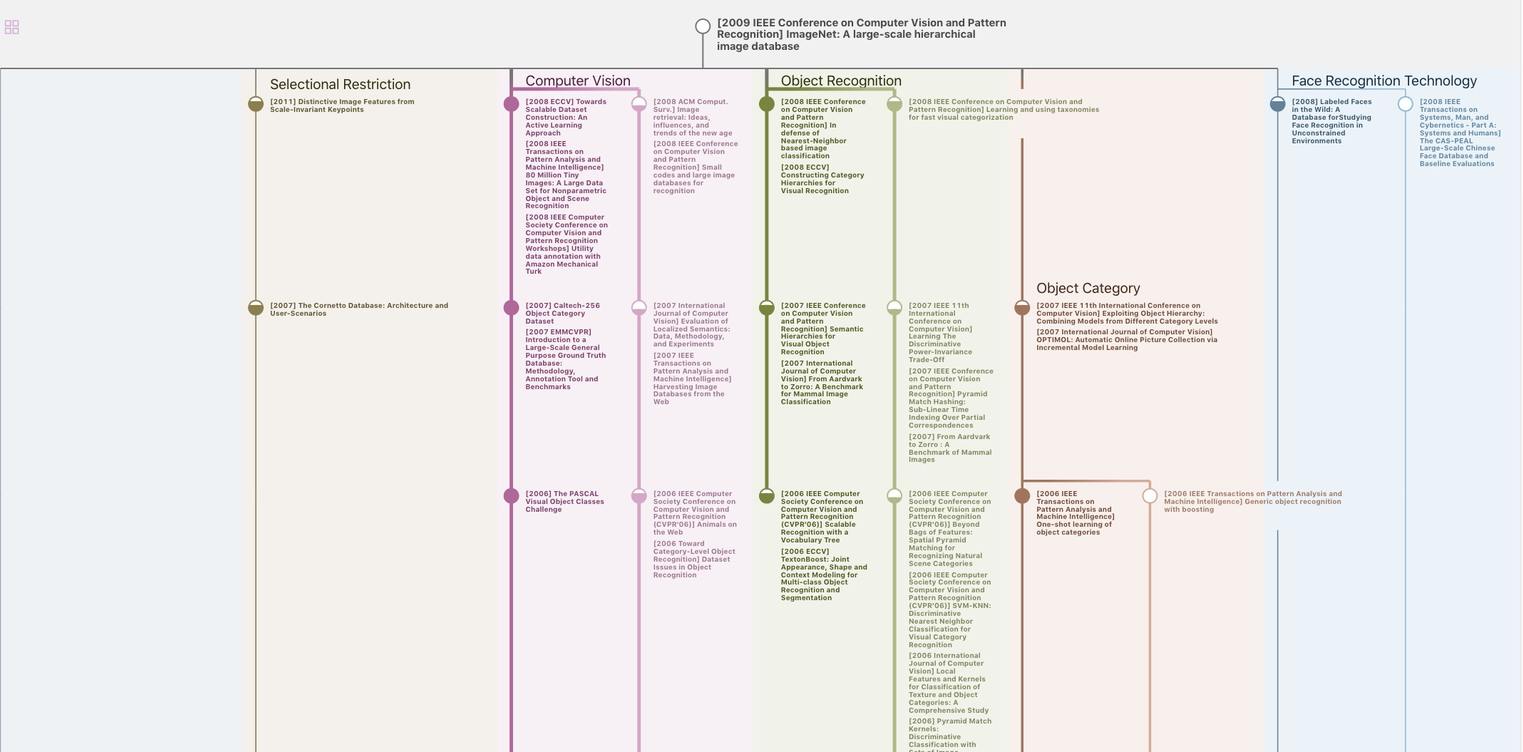
生成溯源树,研究论文发展脉络
Chat Paper
正在生成论文摘要