Unsupervised deep hashing with deep semantic distillation
2023 IEEE INTERNATIONAL CONFERENCE ON IMAGE PROCESSING, ICIP(2023)
摘要
Many existing unsupervised hashing methods attempt to preserve as much semantic information as possible by reconstructing the input data. However, this approach can result in the hash code preserving a lot of redundant information. Besides, previous works usually adopt local structures to guide hashing learning, which will mislead hashing model due to a large amount of noise existing in the local structure. In this paper, we propose a novel Deep Semantic Distillation Hashing (DSDH) to solve the above problems. Specifically, to ensure that the hashing model focuses on preserving more discriminative information rather than background noise, we use random masked images as input for feature extraction. We then apply empirical Maximum Mean Discrepancy to match the output feature distribution with that of the original image. Additionally, to avoid misleading, we propose to constrain the consistency of the similarity structures of the two spaces from the perspective of global distribution, thus transferring the knowledge of the feature space to Hamming space. Experiments conducted on three benchmarks show the superiority of DSDH.
更多查看译文
关键词
deep hashing,image retrieval,unsupervised method
AI 理解论文
溯源树
样例
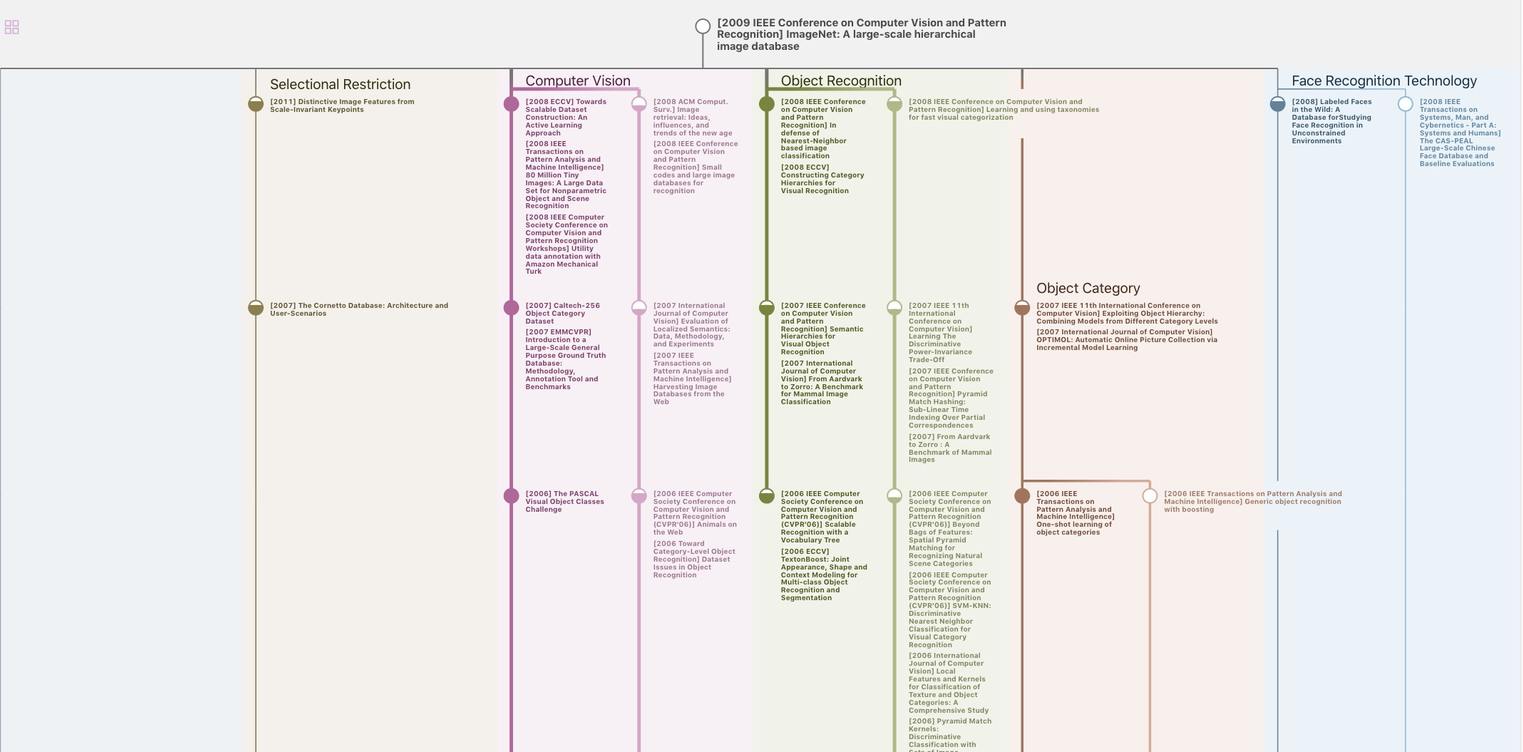
生成溯源树,研究论文发展脉络
Chat Paper
正在生成论文摘要