Cocca: point cloud completion through cad cross-attention
2023 IEEE INTERNATIONAL CONFERENCE ON IMAGE PROCESSING, ICIP(2023)
摘要
3D scene- and object-level scans typically result in sparse and incomplete point clouds. Since dense point clouds of high quality are essential for the 3D reconstruction process, a promising approach is to improve the scan quality by point cloud completion. In this paper, we present COCCA, an extension of point cloud completion networks for scan-to-CAD use cases. The proposed extension is based on cross-attention of features extracted from a scan with rotation-, translation-, and scale-invariant features extracted from a sampled CAD point cloud. With the proposed cross-attention operation, we improve the learning of scan features and the subsequent decoding to a complete shape. We demonstrate the effectiveness of COCCA on the ShapeNet dataset in quantitative and qualitative experiments. COCCA improves the overall completion performance of point cloud completion networks by up to 11.8% for Chamfer Distance and up to 2.2% for F-Score. Our qualitative experiments visualize how COCCA completes point clouds with higher geometric detail. In addition, we demonstrate how completion by COCCA improves the point cloud registration task required for scan-to-CAD alignment.
更多查看译文
关键词
Group-invariance,Cross-Attention,Point Cloud Completion,Scan-to-CAD,Deep Learning
AI 理解论文
溯源树
样例
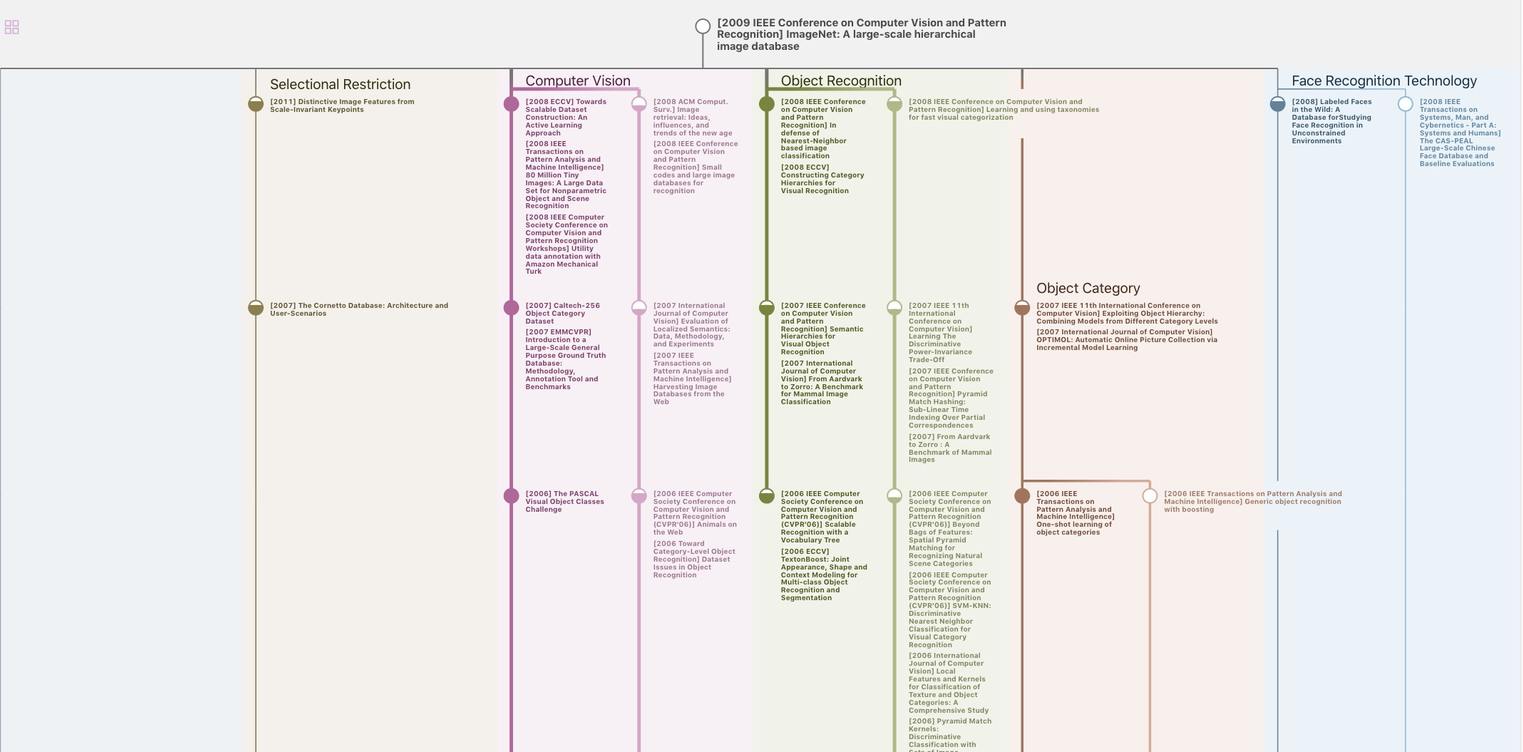
生成溯源树,研究论文发展脉络
Chat Paper
正在生成论文摘要