Highway Traffic State Estimation Based on Data Fusion in a Connected Vehicle Environment
2023 7th International Conference on Transportation Information and Safety (ICTIS)(2023)
摘要
Traffic state estimation plays an important role in urban intelligent transportation management. Compared to traditional traffic data collection means using fixed and mobile detectors, connected vehicles can acquire richer and more diverse data of high precision, which is ideal for discerning traffic states. Nevertheless, the market penetration rate (MPR) of connected vehicles may remain low for a relatively long time. In order to predict traffic states more accurately using the connected vehicle data, in this paper, we propose a data fusion method based on Back-Propagation (BP) neural network model optimized by Particle Swarm optimization (PSO). The model considers the time correlation of traffic flow and combines the information of real-time and historical speeds of the connected vehicles to estimate the travel time on a highway section. The results show that the traffic data fusion method can reduce the estimation errors caused by the low penetration rate of connected vehicles, and improve the accuracy of road traffic state estimation in partially connected driving environments, compared to using only the current connected vehicle data.
更多查看译文
关键词
data fusion,BP neural network,PSO,traffic speed estimation,connected vehicle
AI 理解论文
溯源树
样例
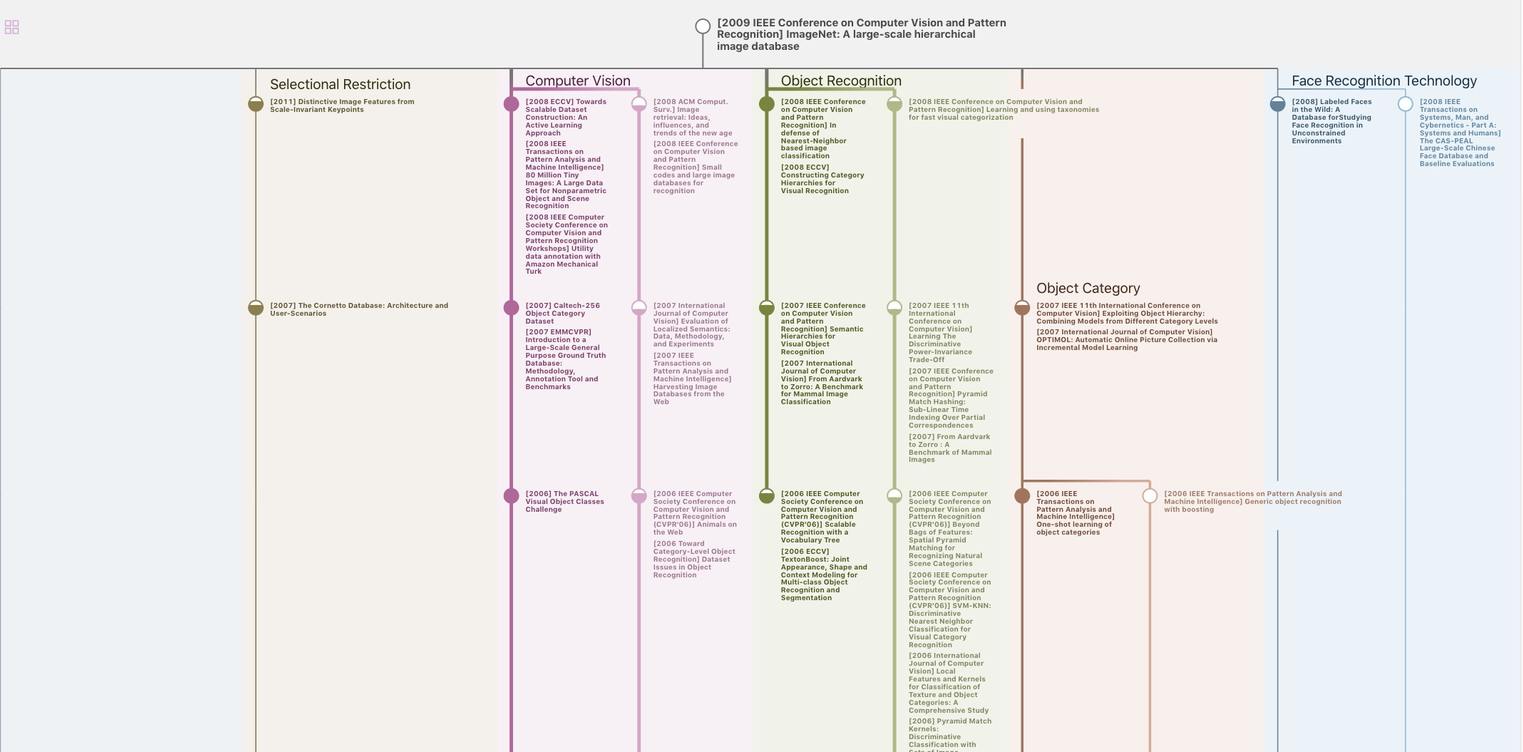
生成溯源树,研究论文发展脉络
Chat Paper
正在生成论文摘要