TLS-Net: A Hybrid Time Series Prediction Model Combining TCN and LSTM for Ship-Satellite Network Traffic
2023 7th International Conference on Transportation Information and Safety (ICTIS)(2023)
摘要
Accurate prediction of ocean-going ship satellite network traffic (OSSNT) can optimize communication resource allocation and improve communication efficiency. But ordinary statistical learning models cannot capture the non-linear relationships between data well. Standalone single deep learning prediction models cannot extract short-term features and capture long-term dependence in time series data simultaneously. In addition, traffic forecasting studies for ship satellite networks have not been investigated due to the unavailability of data. In this paper, a real-world situation-based ship-satellite network traffic dataset is constructed. Furthermore, a hybrid time series prediction neural network model (TLS-Net), which is built on Temporal Convolutional Network (TCN) and Long Short-Term Memory (LSTM), is proposed to forecast OSSNT. This model learns short-term local feature in the time series from TCN, and then captures the long-term dependence of relevant data by LSTM, which extracts multi-source temporal feature and high and low-frequency information. Considering the high noise and non-linear in the raw data, we use Savitzky-Golay filters to eliminate potential noise. Experimental results demonstrate that the proposed model predicts network traffic more accurately than other vanilla prediction models.
更多查看译文
关键词
Satellite network traffic forecasting for ships,Temporal Convolutional Network,Long Short-Term Memory
AI 理解论文
溯源树
样例
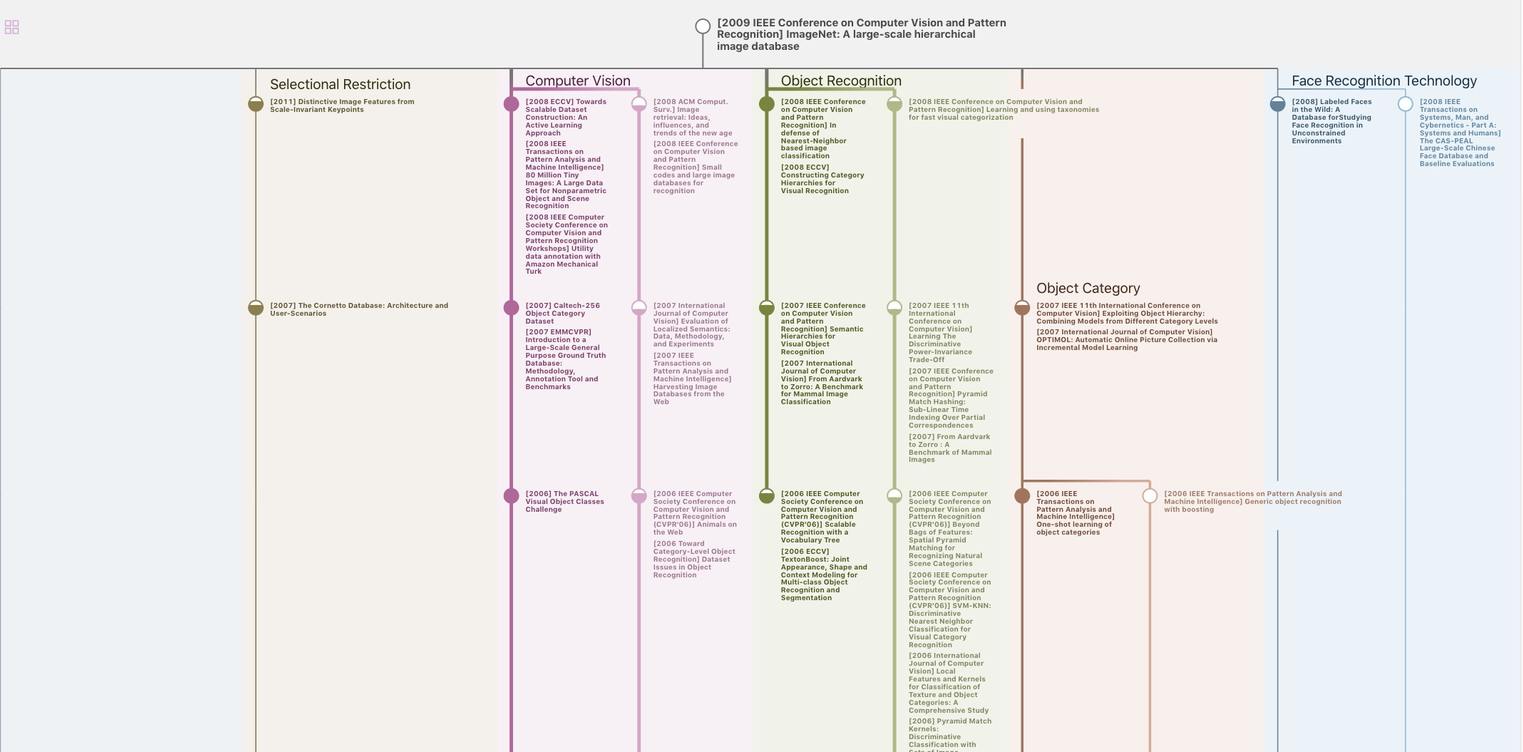
生成溯源树,研究论文发展脉络
Chat Paper
正在生成论文摘要