An Adaptive GLMB Filter with Unknown Environmental Parameters
2023 8th International Conference on Intelligent Computing and Signal Processing (ICSP)(2023)
摘要
In the context of multi-target tracking based on random finite sets, the main challenge lies in the random fluctuations in both the number and state of targets. In most target tracking application scenarios, model parameters such as clutter rate and detection probability are unknown and time-varying; in addition, the birth intensity of nascent targets is difficult to predict. All of this information significantly affects the tracking accuracy of the filter and is usually assumed to be known and provided by the user. In this study, we propose an adaptive generalized labeled multi-Bernoulli (GLMB) filter capable of estimating the unknown parameters and the birth intensity of nascent targets. This filter combines the measurement-driven method and uses the robust multi-Bernoulli filter to calculate the clutter rate and detection probability, which can track multiple targets without the need for priori information. The simulation results demonstrate that the proposed method significantly improves the multi-target tracking performance, outperforming the CBMeMBer and CPHD filters with known tracking scene information in terms of tracking effectiveness. Meanwhile, the proposed method achieves tracking performance that is comparable to that of the GLMB filter.
更多查看译文
关键词
component,random finite sets,generalized labeled multi-Bernoulli,multi-object tracking
AI 理解论文
溯源树
样例
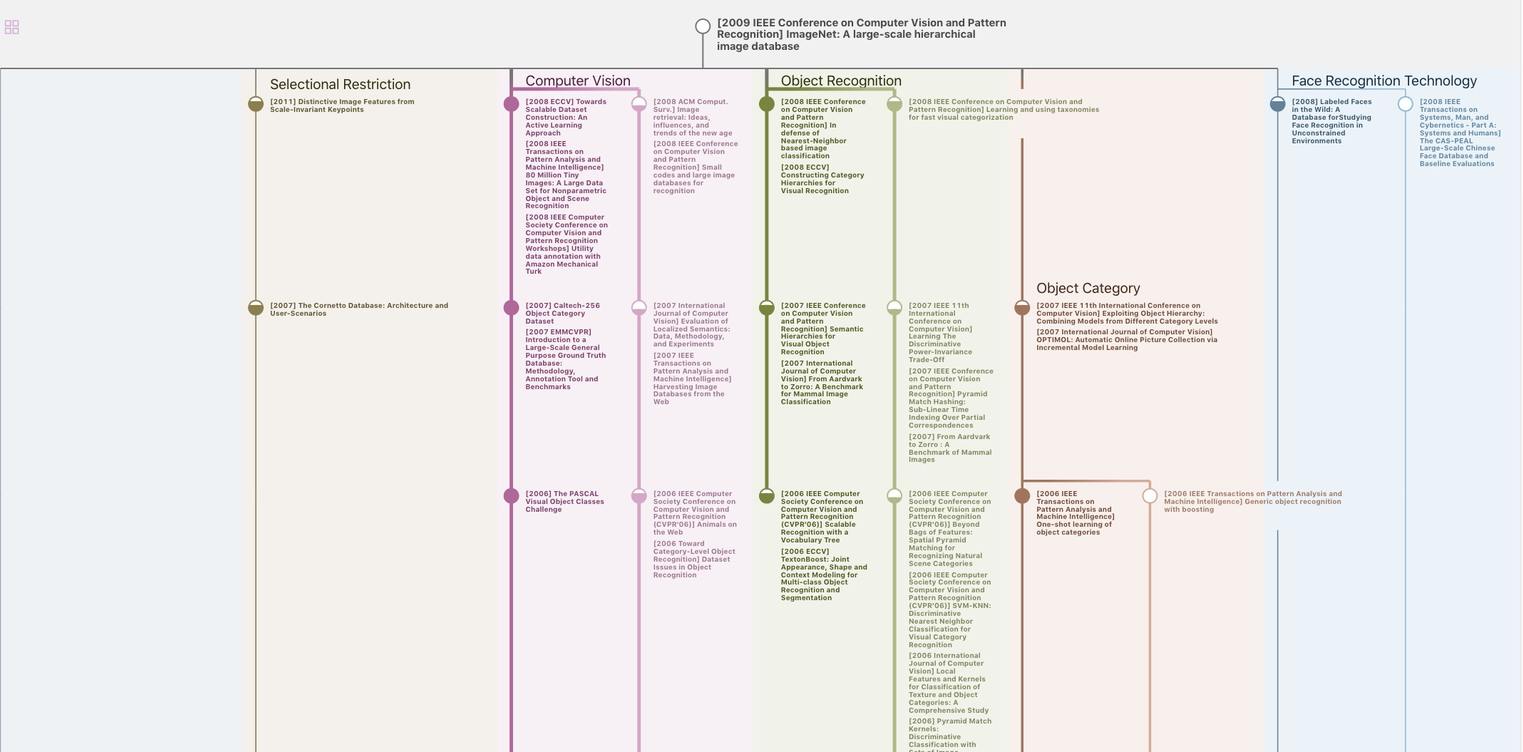
生成溯源树,研究论文发展脉络
Chat Paper
正在生成论文摘要